Data analytics is crucial for businesses today. With vast amounts of data generated every second, making sense of this data can be overwhelming. This is where AI tools come into play. They can analyze large datasets quickly, identify patterns, and provide actionable insights. In this article, we’ll explore some of the top AI tools for advanced data analytics, highlighting their features and how they can benefit your business.
Understanding the Importance of AI in Data Analytics
What is AI in Data Analytics?
AI in data analytics involves using artificial intelligence techniques to process and analyze data. This includes machine learning algorithms, natural language processing, and neural networks.
These tools can handle massive datasets, identify trends, and make predictions, all with minimal human intervention.
Why Use AI for Data Analytics?
The sheer volume of data businesses collect today makes manual analysis impractical. AI tools can quickly sift through this data, providing insights that would take humans much longer to uncover.
This leads to more informed decision-making, better customer insights, and optimized operations.
Top AI Tools for Advanced Data Analytics
IBM Watson Analytics
IBM Watson Analytics is a powerful AI tool that offers data visualization, automated data preparation, and predictive analytics. Its natural language processing capabilities make it easy to interact with, allowing users to ask questions in plain English and get insights in return.
IBM Watson can help businesses identify trends, patterns, and anomalies in their data, making it a valuable tool for any organization.
Google Cloud AI Platform
Google Cloud AI Platform provides a comprehensive suite of tools for building, training, and deploying machine learning models. It supports various machine learning frameworks like TensorFlow and PyTorch, making it versatile for different use cases.
Google Cloud AI Platform also offers AutoML, which enables users to create custom models without needing deep machine learning expertise. This platform is ideal for businesses looking to leverage AI for predictive analytics and decision-making.
Microsoft Azure Machine Learning
Microsoft Azure Machine Learning is another robust AI tool that helps businesses build, train, and deploy machine learning models. It offers a drag-and-drop interface, making it accessible even for those without extensive programming skills.
Azure Machine Learning also integrates seamlessly with other Microsoft services, providing a cohesive ecosystem for data analytics. Its automated machine learning capabilities streamline the model-building process, allowing businesses to focus on deriving insights rather than technical details.
DataRobot
DataRobot is designed to automate the end-to-end process of building, deploying, and maintaining machine learning models. It provides an intuitive interface that guides users through data preparation, model selection, and deployment.
DataRobot’s automated machine learning features enable businesses to quickly develop models and gain insights from their data. This tool is particularly useful for organizations that want to leverage AI without investing heavily in data science resources.
SAS Viya
SAS Viya is an analytics platform that supports AI and machine learning. It offers a wide range of tools for data preparation, visualization, and model building. SAS Viya’s strength lies in its ability to handle large datasets and complex analytical tasks.
It provides a collaborative environment where data scientists and business analysts can work together to extract insights from data. SAS Viya is ideal for organizations looking to perform advanced analytics on a scalable platform.
H2O.ai
H2O.ai is an open-source AI platform that offers tools for building machine learning models. It supports various algorithms and provides an easy-to-use interface for model development.
H2O.ai’s AutoML feature automates the model-building process, making it accessible to users with limited machine learning expertise. This platform is known for its speed and efficiency, making it a popular choice for businesses that need to analyze large datasets quickly.
RapidMiner
RapidMiner is a data science platform that offers a suite of tools for data preparation, machine learning, and model deployment. It provides a visual workflow designer that makes it easy to build complex analytical processes without extensive coding knowledge.
RapidMiner supports a wide range of data sources and integrates with popular machine learning libraries. Its collaborative features allow data scientists and business analysts to work together seamlessly, making it a great choice for organizations looking to leverage AI for data analytics.
Alteryx
Alteryx is a powerful data analytics platform that combines data preparation, blending, and advanced analytics. It offers an intuitive drag-and-drop interface that simplifies the process of building analytical workflows.
Alteryx’s predictive analytics tools enable users to create machine learning models and derive actionable insights from their data. It also supports integration with various data sources and popular visualization tools like Tableau and Qlik. Alteryx is ideal for businesses that need to streamline their data analytics processes and improve decision-making.
KNIME
KNIME is an open-source analytics platform that offers a comprehensive suite of tools for data integration, processing, and analysis. It provides a visual workflow editor that allows users to build complex data pipelines without writing code.
KNIME supports a wide range of data sources and machine learning libraries, making it a versatile tool for data scientists and analysts. Its community-driven approach ensures continuous development and improvement, making KNIME a reliable choice for advanced data analytics.
Domino Data Lab
Domino Data Lab is an enterprise data science platform that helps organizations manage the end-to-end data science lifecycle. It provides tools for data preparation, model development, and deployment, along with collaboration features that enable teams to work together effectively.
Domino’s version control and reproducibility features ensure that data science projects are well-documented and can be easily shared and replicated. This platform is suitable for large organizations that need to scale their data science efforts and maintain control over their analytical processes.
Databricks
Databricks is a unified analytics platform that combines data engineering, data science, and machine learning. Built on Apache Spark, Databricks provides a scalable and efficient environment for processing large datasets.
It offers collaborative notebooks that allow data scientists and engineers to work together on data pipelines and machine learning models. Databricks also integrates with popular data storage and visualization tools, making it a comprehensive solution for advanced data analytics.
TIBCO Spotfire
TIBCO Spotfire is an analytics platform that provides tools for data visualization, predictive analytics, and machine learning. It offers an interactive interface that allows users to explore data and create visualizations easily.
Spotfire’s advanced analytics capabilities enable businesses to build machine learning models and derive insights from their data. Its integration with various data sources and enterprise systems makes Spotfire a versatile tool for organizations looking to enhance their data analytics capabilities.
Best Practices for Using AI Tools in Data Analytics
Define Clear Objectives
Before implementing AI tools for data analytics, it’s essential to define clear objectives. Understand what you want to achieve with AI, whether it’s improving decision-making, optimizing operations, or gaining deeper customer insights.
Clear objectives will guide your AI strategy and ensure that you’re focusing on the right areas.
Ensure Data Quality
High-quality data is crucial for effective AI analytics. Ensure that your data is accurate, complete, and up-to-date. Invest in data cleaning and validation processes to maintain data integrity.
Good data management practices will lead to more reliable insights and better business outcomes.
Choose the Right Tools
Select AI tools that align with your business needs and technical capabilities. Evaluate the features, ease of use, scalability, and integration options of different tools.
Choosing the right tools will help you get the most out of your AI investment and achieve your analytics goals.
Build a Skilled Team
A skilled team is essential for successful AI implementation. Ensure that you have data scientists, data engineers, and business analysts who can work together to leverage AI tools effectively.
Provide training and development opportunities to keep your team updated with the latest AI advancements.
Foster a Culture of Innovation
Encourage a culture of innovation within your organization. Promote experimentation with AI tools and techniques to discover new ways to leverage data.
Foster collaboration between different departments to ensure that AI initiatives are aligned with business objectives and drive meaningful results.
Monitor and Improve Continuously
AI models and tools require continuous monitoring and improvement. Regularly assess the performance of your AI models, update them with new data, and refine your analytical processes.
Staying up-to-date with the latest developments in AI technology will help you maintain a competitive edge.
Real-World Applications of AI Tools in Data Analytics
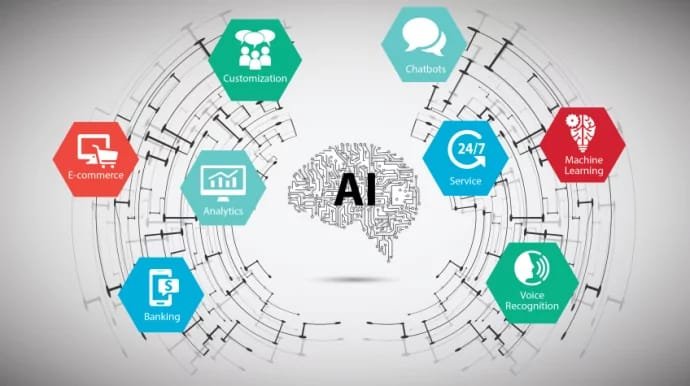
Retail Industry
In the retail industry, AI tools are used to analyze customer behavior, optimize inventory management, and personalize marketing campaigns.
For example, a major retail chain implemented AI-driven predictive analytics to forecast demand more accurately, reducing stockouts and overstock situations. This led to improved customer satisfaction and increased sales.
Financial Services
Financial institutions use AI tools to detect fraud, assess risks, and optimize investment strategies. A financial services firm used AI to analyze transaction data and identify suspicious activities, enhancing its fraud detection capabilities.
The firm also leveraged AI-driven predictive models to improve risk assessment and investment decision-making.
Healthcare Sector
In healthcare, AI tools are used to analyze patient data, improve treatment outcomes, and optimize operational efficiency. A healthcare provider used AI-driven predictive analytics to identify high-risk patients and intervene early, resulting in better patient outcomes and reduced healthcare costs.
AI tools also streamlined administrative processes, freeing up staff to focus on patient care.
Manufacturing Industry
Enhancing Quality Control
In the manufacturing industry, AI tools are used to enhance quality control by analyzing production data in real-time. AI models can detect defects and irregularities in the production process, allowing businesses to address issues quickly and maintain high product standards.
For instance, a manufacturing company implemented AI-driven image recognition to inspect products on the assembly line, reducing defects and improving overall product quality.
Predictive Maintenance
AI tools are also used for predictive maintenance in manufacturing. By analyzing data from sensors and machinery, AI can predict when equipment is likely to fail and schedule maintenance before issues arise.
This approach minimizes downtime, reduces maintenance costs, and ensures smooth production operations. A manufacturing plant utilized AI-driven predictive maintenance to monitor machinery health, resulting in significant cost savings and improved efficiency.
Telecommunications Industry
Network Optimization
In the telecommunications industry, AI tools are used to optimize network performance and ensure reliable service delivery. AI models analyze network data to identify congestion points, predict demand, and optimize resource allocation.
This helps telecommunications companies provide better service quality and reduce operational costs. A telecom provider used AI-driven network optimization to enhance bandwidth management, leading to improved customer satisfaction and reduced outages.
Customer Service Enhancement
AI tools are also deployed to improve customer service in the telecommunications industry. AI-powered chatbots and virtual assistants handle routine customer inquiries, provide technical support, and resolve issues quickly.
This enhances customer satisfaction and allows human agents to focus on more complex tasks. A telecom company implemented AI-driven chatbots to handle customer queries, resulting in faster response times and improved customer service ratings.
Energy Sector
Demand Forecasting
In the energy sector, AI tools are used for demand forecasting to ensure efficient energy distribution and consumption. AI models analyze historical consumption data, weather patterns, and other factors to predict energy demand accurately.
This helps energy providers optimize supply, reduce waste, and ensure a stable energy supply. An energy company utilized AI-driven demand forecasting to better match supply with demand, leading to reduced energy costs and increased efficiency.
Renewable Energy Management
AI tools also play a crucial role in managing renewable energy sources. By analyzing data from solar panels, wind turbines, and other renewable energy systems, AI models optimize energy production and storage.
This ensures a consistent and reliable supply of renewable energy. A renewable energy firm implemented AI-driven management systems to optimize the performance of its solar farms, resulting in increased energy output and reduced operational costs.
Education Sector
Personalized Learning
In the education sector, AI tools are used to create personalized learning experiences for students. AI models analyze student performance data to identify learning gaps and recommend customized learning paths.
This approach enhances student engagement and improves learning outcomes. An educational institution used AI-driven personalized learning platforms to tailor coursework to individual student needs, resulting in higher student satisfaction and better academic performance.
Administrative Efficiency
AI tools also streamline administrative tasks in educational institutions. By automating processes such as enrollment, scheduling, and grading, AI reduces administrative burden and frees up staff to focus on teaching and student support.
A university implemented AI-driven administrative systems to handle routine tasks, improving efficiency and allowing faculty to dedicate more time to student interaction.
Implementing AI Tools in Your Business
Define Your Goals
Before implementing AI tools, clearly define your goals and what you hope to achieve. Whether it’s improving decision-making, enhancing customer service, or optimizing operations, having clear objectives will guide your AI strategy and ensure you focus on the right areas.
Assess Your Data
Evaluate the quality and availability of your data. AI tools rely on high-quality data to generate accurate insights.
Ensure your data is clean, complete, and up-to-date. Invest in data management practices to maintain data integrity and reliability.
Choose the Right AI Tools
Select AI tools that align with your business needs and technical capabilities. Consider factors such as ease of use, scalability, integration options, and support resources.
Choosing the right tools will help you achieve your analytics goals more effectively.
Build a Skilled Team
Assemble a team with the necessary skills to leverage AI tools effectively. This includes data scientists, data engineers, and business analysts.
Provide training and development opportunities to keep your team updated with the latest AI advancements.
Foster a Culture of Innovation
Encourage a culture of innovation within your organization. Promote experimentation with AI tools and techniques to discover new ways to leverage data.
Foster collaboration between different departments to ensure that AI initiatives are aligned with business objectives and drive meaningful results.
Monitor and Improve Continuously
Regularly assess the performance of your AI models and tools. Update them with new data and refine your analytical processes to maintain accuracy and relevance.
Stay informed about the latest developments in AI technology to keep your business competitive.
Overcoming Challenges in AI Data Analytics Implementation
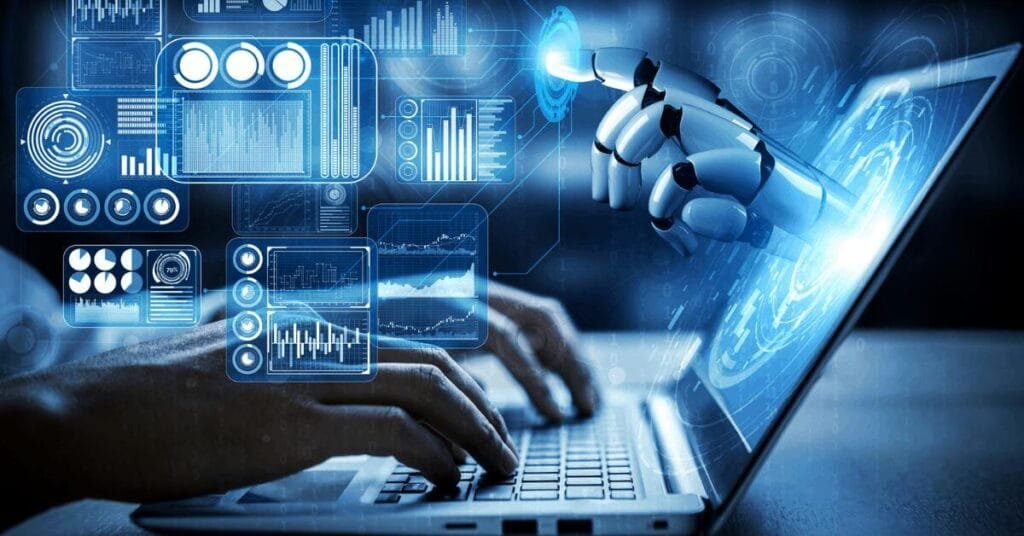
Addressing Data Quality Issues
One of the biggest challenges in implementing AI data analytics is ensuring data quality. Poor-quality data can lead to inaccurate insights and flawed decision-making. To address this, businesses should invest in robust data management practices.
This includes data cleaning, validation, and enrichment processes. Regular audits and data governance frameworks can help maintain high data quality, ensuring reliable AI-driven insights.
Managing Complexity and Integration
AI tools can be complex to implement and integrate with existing systems. Businesses need to carefully plan the integration process to avoid disruptions.
This involves assessing the compatibility of AI tools with current infrastructure and ensuring seamless data flow between systems. Using middleware and APIs can facilitate integration, making it easier to incorporate AI tools into existing workflows.
Ensuring Data Privacy and Security
AI systems often require access to large amounts of data, including sensitive information. Ensuring data privacy and security is paramount.
Businesses should implement strong data protection measures, such as encryption, access controls, and compliance with relevant regulations like GDPR. Regular security assessments and audits can help identify and mitigate potential risks, ensuring that AI systems handle data responsibly.
Mitigating Bias in AI Models
AI models can inherit biases present in the training data, leading to unfair or inaccurate outcomes. To mitigate bias, businesses should use diverse and representative datasets.
Regularly reviewing and testing AI models for biases is essential. Implementing fairness-aware algorithms and promoting transparency in AI processes can also help build trust and accountability.
Building Trust in AI Systems
Building trust in AI systems is crucial for their successful adoption. Transparency and explainability are key factors in building trust.
Providing clear explanations for AI-driven insights helps stakeholders understand and trust the technology. Regular audits and assessments of AI systems ensure their reliability and effectiveness, fostering confidence in their use.
Future Trends in AI Data Analytics
Integration with Blockchain Technology
The integration of AI with blockchain technology is emerging as a significant trend. Blockchain provides a secure and transparent way to store and manage data, enhancing data integrity and security.
Combining AI with blockchain can improve data provenance, auditability, and trustworthiness. This integration is particularly valuable in industries such as finance, supply chain, and healthcare, where data security and transparency are critical.
AI-Driven Automation
AI-driven automation is set to revolutionize various business processes. From automating customer service interactions to streamlining supply chain operations, AI can handle repetitive tasks efficiently.
This leads to increased productivity and reduced operational costs. Businesses are increasingly adopting AI-driven automation to enhance their operations and focus on strategic activities.
Explainable AI (XAI)
As AI becomes more integrated into business processes, the need for explainable AI grows. Explainable AI (XAI) focuses on making AI models more transparent and understandable.
XAI helps businesses trust AI decisions and ensures they align with their values and objectives. By providing clear explanations for how AI models reach their conclusions, businesses can improve accountability and trust in AI systems.
AI in Edge Computing
Edge computing involves processing data closer to where it is generated, rather than in a centralized data center. AI in edge computing allows real-time data analysis and decision-making at the edge of the network.
This reduces latency and bandwidth usage, making it ideal for applications that require quick responses, such as autonomous vehicles and IoT devices. The combination of AI and edge computing is expected to drive significant advancements in various industries.
Enhanced Natural Language Processing (NLP)
Natural Language Processing (NLP) is continuously evolving, making it easier for businesses to analyze and interact with textual data.
Advances in NLP are opening up new possibilities for AI in data analytics, from sentiment analysis in customer feedback to automated report generation. Improved NLP capabilities enhance the accuracy and usability of AI-driven insights, enabling businesses to make more informed decisions.
Preparing for AI-Driven Innovation
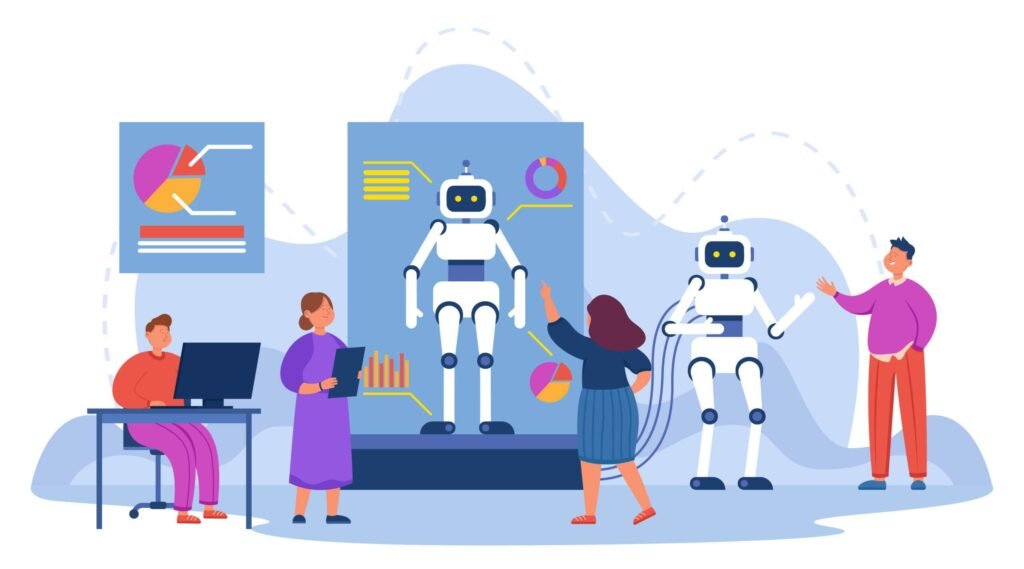
Embracing a Forward-Thinking Mindset
To fully leverage AI for data analytics, businesses must embrace a forward-thinking mindset.
This involves staying open to new ideas, experimenting with innovative technologies, and continuously seeking ways to improve. By fostering a culture of innovation, businesses can stay ahead of the curve and capitalize on emerging opportunities.
Building Strategic Partnerships
Building strategic partnerships with AI vendors, research institutions, and industry experts can provide businesses with valuable insights and support.
These partnerships can help businesses stay informed about the latest AI developments, access cutting-edge technology, and receive expert guidance on AI implementation. Collaborating with external partners can also lead to joint innovations and shared success.
Investing in Talent and Technology
Investing in talent and technology is crucial for successful AI integration. Businesses should prioritize hiring skilled data scientists, engineers, and analysts, as well as providing ongoing training and development opportunities.
Additionally, investing in advanced AI tools and infrastructure ensures that businesses have the resources they need to succeed. Continuous investment in talent development keeps the workforce ready for future AI advancements.
Staying Agile and Adaptable
The AI landscape is constantly evolving, and businesses must stay agile and adaptable to keep up. This involves regularly reviewing and updating AI strategies, being willing to pivot when needed, and embracing a flexible approach to innovation. By staying agile, businesses can quickly respond to changes and take advantage of new opportunities.
Maintaining an agile approach helps businesses navigate the uncertainties of AI implementation effectively.
Wrapping it up
AI tools for advanced data analytics are transforming the way businesses operate, providing deep insights, automating tasks, and enhancing decision-making. To fully leverage these tools, businesses must prioritize data quality, ethical practices, and continuous innovation. By choosing the right AI tools, building a skilled team, and fostering a culture of innovation, companies can gain a competitive edge.
As AI technology continues to evolve, staying informed and adaptable will be crucial for success. Embracing AI in data analytics will lead to better decisions, optimized operations, and improved customer experiences, positioning businesses for long-term success in a data-driven future.
READ NEXT: