Predictive analytics is a branch of advanced analytics that uses current and historical data, statistical algorithms, and machine learning techniques to predict future outcomes. This powerful tool is rapidly transforming the landscape of various industries, with sales being one of its most influential domains.
Understanding Predictive Analytics in the Context of Sales
In the business world, sales have always been a number’s game, but with predictive analytics, the game is being played more strategically than ever before.
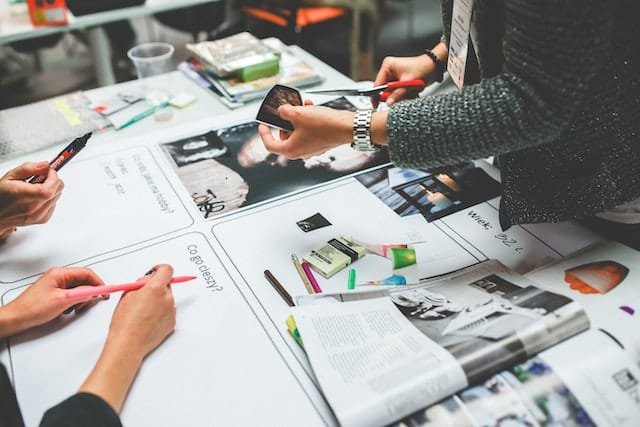
What Makes Predictive Analytics Vital in Sales
Predictive analytics has an increasingly important role in sales forecasting. It enables businesses to use historical data, statistical algorithms, and machine learning techniques to predict future outcomes. This provides valuable insights and assists companies in making well-informed decisions about future sales strategies. But how exactly does predictive analytics influence sales forecasting? Let’s delve deeper.
Role of Predictive Analytics in Sales Forecasting
The Predictive Shift: From Guesswork to Data-Driven Forecasts
Sales forecasts have traditionally been based on intuition, gut feelings, and past experiences. But these methods are often susceptible to human error, bias, and unforeseen external factors. Predictive analytics revolutionizes this approach by relying on data and statistics to forecast future sales trends.
Predictive analytics extracts information from existing data sets to determine patterns and predict future outcomes and trends. In the context of sales, predictive analytics uses historical sales data, customer behavior data, and market trends to predict future sales performance. This data-driven approach increases the accuracy of sales forecasts, allowing for improved business planning and strategy.
Dynamic Adjustments: Predictive Analytics for Real-Time Forecasting
Predictive analytics not only improves the accuracy of sales forecasts, but it also enables real-time forecasting. Traditional forecasting methods often involve static, one-time predictions. However, predictive analytics allows for dynamic adjustments based on real-time data.
Sales patterns can change rapidly due to various factors, such as market trends, competitive actions, and changes in customer behavior. Predictive analytics can adjust forecasts in real-time to reflect these changes, providing businesses with up-to-date insights for decision-making.
Influencing Strategic Decisions: Predictive Analytics for Informed Planning
Predictive analytics is not just about forecasting sales; it is also a tool for strategic decision-making. By predicting future sales trends, businesses can make informed decisions about various aspects of their operations.
For instance, a business can plan its production schedule, inventory management, and staffing needs based on sales forecasts. If the predictive analytics model forecasts high sales in the upcoming quarter, the business can increase production, stock up on inventory, and hire additional staff to meet the anticipated demand.
Additionally, predictive analytics can also guide sales and marketing strategies. Businesses can identify potential sales opportunities, target profitable customer segments, and plan marketing campaigns based on predictive insights.
Risk Management: Predictive Analytics for Minimizing Uncertainty
Uncertainty is a constant in business, particularly when it comes to sales forecasting. However, predictive analytics can help minimize this uncertainty.
Predictive analytics models consider a range of factors that influence sales, including historical sales data, market trends, seasonality, and more. By considering these factors, predictive analytics can forecast a range of potential sales outcomes, providing businesses with a comprehensive view of potential future scenarios.
This allows businesses to prepare for various scenarios and implement contingency plans, thereby minimizing the risks associated with sales forecasting.
Differentiating Traditional Forecasting and Predictive Sales Forecasting
Sales forecasting is a fundamental aspect of business operations. However, traditional forecasting methods have evolved, giving way to more sophisticated predictive sales forecasting techniques. To appreciate the shift, it is necessary to understand the distinct characteristics of both approaches.
Characteristics of Traditional Forecasting
Traditional forecasting largely depends on human intuition and historical data. Let’s further break it down:
Historical Data: Traditional forecasting heavily relies on historical sales data as a primary indicator of future performance. For instance, if sales have been increasing by 10% every year, it would forecast the same for the coming years.
Qualitative Methods: Often, sales teams use their judgement to create forecasts, factoring in their understanding of the market and the customer base. This might involve using methods like sales force composite, where the sales team collectively estimates the sales for a future period.
Static Models: Traditional forecasting models are generally static, meaning they lack the flexibility to adapt to changing trends and patterns effectively. Once a forecast is established, there’s little room for adjustment.
Characteristics of Predictive Sales Forecasting
Predictive sales forecasting, on the other hand, employs advanced data analytics to forecast future sales. Here’s what sets it apart:
Use of Diverse Data Sets: Unlike traditional methods that primarily use historical sales data, predictive analytics leverages a variety of data sets. These can include economic indicators, customer behavior patterns, market trends, and competitor data, which provide a more comprehensive basis for making predictions.
Predictive Models: Predictive forecasting uses statistical and machine learning algorithms to identify patterns and relationships in the data. These models are capable of capturing complex, non-linear relationships that are often overlooked by traditional methods.
Dynamic and Adaptive: Predictive models can learn and adapt to new data. As additional data becomes available, the models update themselves, ensuring they continually reflect the most recent trends and patterns.
Quantitative Approach: Predictive sales forecasting is a quantitative technique that minimizes human bias. Predictions are based on hard data, ensuring a higher degree of accuracy and reliability.
In essence, while traditional forecasting relies primarily on historical sales data and human judgement, predictive sales forecasting leverages advanced algorithms and a broader range of data to make forecasts. Predictive sales forecasting is typically more accurate and adaptable, making it a powerful tool for businesses operating in fast-paced and volatile markets.
The shift from traditional forecasting to predictive sales forecasting represents a significant advancement in the field of sales management. Businesses that harness the power of predictive analytics are better positioned to anticipate market changes, optimize resource allocation, and ultimately, achieve their sales goals.
Principles and Processes of Predictive Analytics in Sales Forecasting
Predictive analytics in sales forecasting doesn’t simply provide sales teams with data; it also offers actionable insights, making it an invaluable tool for sales strategy and decision-making. Let’s dive deeper into the principles and processes that make this possible.
The Principles Behind Predictive Analytics for Sales
Predictive analytics is revolutionizing the way businesses forecast sales. It involves the application of data, statistical algorithms, and machine learning techniques to predict future outcomes, providing organizations with a strategic edge in a competitive market. However, the effectiveness of predictive analytics in sales forecasting relies on a set of core principles. Let’s break down these principles to understand how predictive analytics drives sales forecasting.
Embracing a Data-Driven Culture
Predictive analytics is built on the foundation of data. Organizations must not only collect and store data but must also foster a culture that values data-driven decision making. A data-driven culture emphasizes the importance of relying on facts and statistics for strategic decisions, including sales forecasting.
This culture extends beyond the data analytics team to all departments, including sales, marketing, finance, and operations. All stakeholders need to understand the value of data and how it can guide their decisions and actions. A data-driven culture sets the stage for effective utilization of predictive analytics in sales forecasting.
Quality over Quantity: Ensuring Data Quality
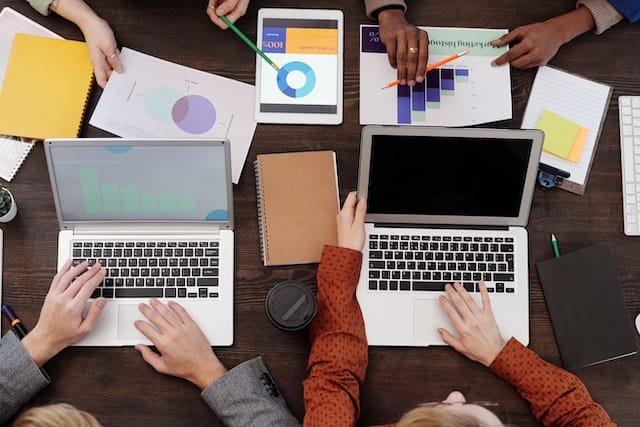
While having a large amount of data is important, the quality of data is paramount for predictive analytics. High-quality data is accurate, complete, consistent, timely, and relevant. Predictive analytics models built on high-quality data are more likely to produce accurate forecasts.
Ensuring data quality involves data cleaning and data validation processes. Data cleaning removes errors and inconsistencies, while data validation ensures the data is accurate and fit for use. A commitment to data quality is an essential principle in predictive analytics for sales forecasting.
Leveraging Advanced Analytics Techniques
Predictive analytics goes beyond basic data analysis to include advanced analytics techniques. These techniques include machine learning, data mining, and statistical modeling, all of which help identify patterns and relationships in data that might be missed with basic analysis.
Machine learning algorithms can learn from data and improve over time, enhancing the accuracy of sales forecasts. Data mining techniques can discover hidden patterns and relationships, providing deeper insights into sales trends. Statistical modeling can identify the variables that have the greatest impact on sales, allowing businesses to focus their efforts on these areas.
Continuous Learning and Adaptation
The business environment is dynamic, with changes in market conditions, customer behavior, and competition. Predictive analytics models need to reflect this dynamism by continuously learning and adapting.
This principle involves regularly updating the predictive analytics models with new data and adjusting the models based on changes in the business environment. This continuous learning and adaptation ensure that the sales forecasts remain accurate and relevant, even in a changing business landscape.
Actionable Insights: Turning Forecasts into Strategy
Predictive analytics is not just about producing accurate sales forecasts; it’s also about turning these forecasts into actionable strategies. This principle emphasizes the importance of using predictive insights to guide strategic decisions, such as sales and marketing strategies, inventory management, and resource allocation.
The goal is not just to predict future sales but to use these predictions to drive business growth and profitability. This principle underlines the strategic value of predictive analytics in sales forecasting.
Detailed Breakdown of the Predictive Analytics Process for Sales Forecasting
The use of predictive analytics in sales forecasting is a systematic process that involves several critical stages. Each stage is essential for the development of a reliable and effective sales forecast. This section provides a detailed breakdown of this process.
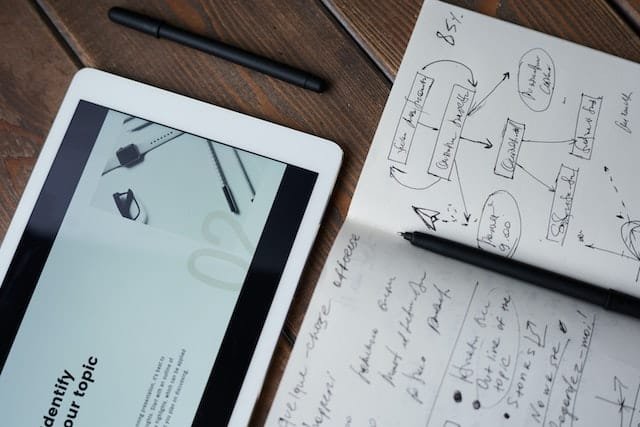
Data Collection
Predictive analytics starts with data collection. It involves gathering historical sales data and other relevant information, such as product details, customer demographics, economic indicators, and market trends. The data may come from various sources, including transaction records, customer relationship management (CRM) systems, and external databases.
This stage requires careful planning to determine what data to collect and how to collect it. The data should be relevant to the sales forecasting task, and it should be collected in a format that can be easily processed and analyzed.
Data Preprocessing
Once the data is collected, it undergoes preprocessing to prepare it for analysis. Preprocessing may involve cleaning the data to remove errors and inconsistencies, transforming the data into a suitable format for analysis, and selecting the most relevant features for forecasting.
Data cleaning is particularly important as it ensures the quality of the data, which directly affects the accuracy of the forecast. Feature selection helps to reduce the complexity of the predictive model and improve its performance.
Model Development
The next step in the predictive analytics process is to develop a predictive model. This involves choosing an appropriate statistical or machine learning algorithm and training it on the preprocessed data. The choice of algorithm depends on the nature of the sales forecasting task and the characteristics of the data.
During training, the algorithm learns patterns and relationships in the data that can be used for forecasting. It may also involve tuning the model’s parameters to optimize its performance. This is often an iterative process, with the model being trained and tested multiple times until it achieves satisfactory results.
Model Validation
After the model is developed, it needs to be validated to assess its forecasting performance. This involves testing the model on a separate set of data, not used in training, and comparing the model’s forecasts with the actual outcomes.
Model validation provides a measure of the model’s predictive accuracy and helps to identify any potential issues, such as overfitting. Overfitting occurs when the model is too complex and fits the training data too closely, causing it to perform poorly on new data.
Forecast Generation
Once the model is validated and fine-tuned, it can be used to generate sales forecasts. The model takes in current and future data, such as upcoming marketing campaigns or seasonal trends, and outputs a forecast of future sales.
The forecasts may be in the form of point predictions, which provide a single value for each future time point, or interval predictions, which provide a range of possible values. Interval predictions can give a sense of the uncertainty associated with the forecast, which is useful for risk management.
Model Updating
The predictive analytics process doesn’t end with the generation of forecasts. As new data becomes available, the predictive model needs to be updated to reflect this data. This ensures that the model stays current and continues to provide accurate forecasts.
Updating the model may involve retraining it with the new data or adjusting its parameters based on the new information. This step is crucial for maintaining the effectiveness of the predictive analytics process in a dynamic business environment.
Real-World Examples of How Predictive Analytics Impacts Sales Forecasting
Having understood the principles and processes involved in predictive analytics for sales forecasting, let’s now delve into real-world examples. These case studies highlight how some of the world’s leading corporations are effectively using predictive analytics in sales forecasting.
Coca-Cola
Coca-Cola, one of the largest beverage companies in the world, is no stranger to the power of predictive analytics. They have leveraged this technology to analyze patterns in sales data and predict future outcomes.
For instance, Coca-Cola has harnessed predictive analytics to determine the demand for their products across different regions and during different seasons. They have used these insights to manage their supply chain more efficiently, ensuring that they have the right products in the right quantities at the right locations.
Moreover, the beverage giant uses predictive analytics to determine the potential impact of price changes on sales volumes, helping them make informed pricing decisions.
The results have been impressive, with Coca-Cola experiencing improvements in sales forecasting accuracy and supply chain efficiency, leading to reduced costs and increased profits.
Amazon
Amazon, the e-commerce behemoth, has a reputation for pushing the boundaries of technology, and their use of predictive analytics in sales forecasting is no exception.
Amazon uses predictive analytics to forecast demand for the millions of products it sells. These forecasts inform various aspects of Amazon’s operations, including inventory management, warehouse management, and shipping.
One notable example is Amazon’s “anticipatory shipping” patent, a system designed to predict what customers will buy before they even make a purchase. The system uses predictive analytics to analyze a variety of factors, including previous purchases, product searches, and even the time a user spends on a page.
Using these predictions, Amazon can pre-emptively move products to distribution centers closer to customers who are likely to purchase them, thereby reducing delivery times and enhancing customer satisfaction.
Oracle Corporation
Oracle, the multinational computer technology corporation, is another key player leveraging predictive analytics for sales forecasting.
Oracle’s predictive analytics capabilities are baked into their comprehensive suite of cloud applications, designed to provide businesses with the tools they need to forecast sales accurately.
For instance, Oracle’s predictive analytics solutions leverage AI and machine learning to analyze historical sales data and provide insights into future sales trends. This capability allows Oracle’s customers to predict customer behavior, optimize sales strategies, and forecast revenue with higher accuracy.
As a result, Oracle has been able to help its customers improve their sales forecasting, leading to better business decisions and increased revenue growth.
In these examples, each company used predictive analytics in unique ways, tailored to their specific needs and goals. However, the common thread is the use of predictive analytics to enhance sales forecasting, drive operational efficiency, and ultimately increase profitability.
The Role of Data in Predictive Analytics for Sales Forecasting
As we turn our attention from real-world applications to the backbone of predictive analytics, it’s important to highlight the role data plays. Data is the lifeblood of any predictive analysis process, and its quality significantly impacts the accuracy of sales forecasts.
Understanding the Importance of Quality Data
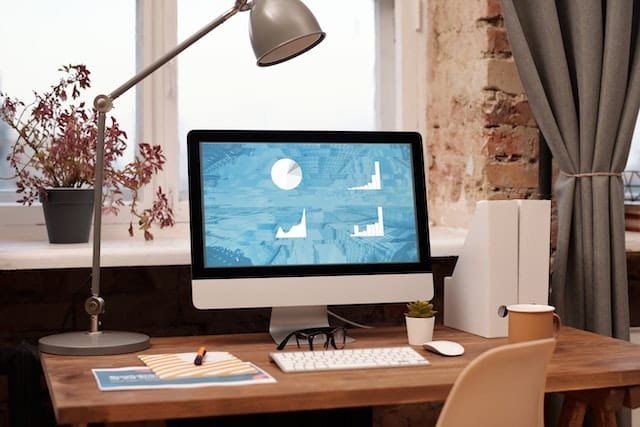
In the realm of predictive analytics, the quality of the input data is a significant determinant of the effectiveness of the sales forecast. This is because the predictions are directly influenced by the data fed into the predictive models. In this section, we will delve into the importance of quality data and its role in shaping successful sales forecasts.
Influence on Model Accuracy
Quality data is paramount as it is directly proportional to the accuracy of the predictive models. The integrity of the data utilized during the training process determines the ability of the model to identify patterns and make reliable predictions. Poor quality data can mislead the model, leading to inaccurate and unreliable forecasts, which in turn can result in poor business decisions and financial losses.
Enabling Relevant Feature Selection
Quality data enhances the feature selection process, a critical phase of model development. Features are the variables or attributes in the data that the model uses to make predictions. Quality data allows for the identification of the most relevant features that significantly impact sales. Irrelevant or noisy features can be eliminated, reducing the complexity of the model and improving its predictive performance.
Compliance and Ethical Considerations
Using quality data is not just a matter of improving predictive accuracy. It’s also about compliance with data regulations and ethical considerations. Companies must ensure that the data they collect is legal, ethical, and respectful of customer privacy. Violations can lead to hefty fines, damaged reputations, and loss of customer trust. Therefore, data quality also encompasses the legitimacy and ethical collection of the data.
Facilitating Efficient Data Preprocessing
Data preprocessing is often the most time-consuming part of the predictive analytics process. It involves cleaning the data, handling missing values, and transforming variables, among other tasks. When the quality of the data is high, less time and resources need to be spent on preprocessing. This means that the model can be developed and deployed more quickly, providing businesses with timely forecasts.
Supporting Confidence in Decision Making
Finally, quality data bolsters confidence in decision-making. When business leaders know that their sales forecasts are based on high-quality data, they can make strategic decisions with greater certainty. This confidence can lead to more proactive and ambitious strategies, contributing to business growth and competitive advantage.
How to Collect, Clean, and Prepare Data for Predictive Analysis
For predictive analytics to be effective in sales forecasting, it is essential to start with high-quality, well-organized data. A major part of predictive analytics involves the collection, cleaning, and preparation of data. This section will provide a step-by-step guide on how to carry out these processes.
Identification of Data Sources
Predictive analysis is heavily reliant on the quality and quantity of data at its disposal. Therefore, the first step involves identifying the right data sources. These sources can be both internal and external. Internal data comes from within the organization and can include transactional data, customer interaction data from CRM systems, and sales records. External data, on the other hand, refers to data outside the organization such as market trends, competitor data, and economic indicators.
Data Collection
Once the data sources are identified, the next step is the collection of the data. Depending on the sources, this can be done through various means like direct extraction from databases, data scraping, using APIs, or through data partnerships. It’s critical to collect data that is relevant, diverse, and substantial enough to create meaningful models.
Data Cleaning
The collected data then needs to be cleaned, a process that involves the removal of duplicates, correction of errors, and dealing with missing values. This step is crucial because unclean data can lead to inaccurate models and misleading forecasts. Data cleaning can be time-consuming but is worth the effort as the quality of data affects the final output significantly.
Data Transformation
Once cleaned, the data may need to be transformed to make it suitable for analysis. This may involve operations such as normalization (scaling data to a small, specified range), conversion of categorical data into numerical data, or even the creation of derived attributes (new attributes created from existing ones to highlight certain trends).
Data Integration
If data has been collected from multiple sources, it’s necessary to integrate this data into a unified view. This involves resolving issues like data conflicts due to different formats, redundancies, or discrepancies in the data collected from different sources.
Feature Selection
Lastly, before feeding the data to a predictive model, it’s essential to conduct feature selection. This step involves selecting the most relevant attributes or features for your predictive model to focus on. Reducing the number of random variables considered can help enhance the model’s performance by simplifying the model’s structure and reducing overfitting.
Challenges and Solutions in Implementing Predictive Analytics for Sales Forecasting
Predictive analytics for sales forecasting holds immense promise in helping businesses achieve greater accuracy in their sales projections. However, several challenges can arise when implementing this technology. Here, we delve into some of these challenges and discuss possible solutions.
Challenge 1:
Data Quality and Integrity
Data is the bedrock of any predictive analytics project. However, maintaining data quality and integrity can be a significant challenge. Sales data can come from multiple sources and can often contain errors or discrepancies.
Solution
The solution to this challenge involves rigorous data management practices. It includes data cleaning, which is the process of detecting and correcting errors, and data validation, which ensures data is accurate and suitable for use. It also involves integrating data from different sources to provide a complete and accurate view of sales data.
Challenge2:
Selection of Appropriate Predictive Models
There’s no one-size-fits-all predictive model for sales forecasting. The effectiveness of a predictive model can vary based on the nature of the business, the market conditions, and the quality and quantity of available data.
Solution
The solution to this challenge is a thorough understanding of various predictive models and their applicability to different situations. Businesses should explore different types of predictive models, such as regression models, time-series models, and machine learning models, and understand their strengths and limitations. Businesses should also conduct iterative testing to determine the most effective model.
Challenge 3:
Skill Gap
Predictive analytics for sales forecasting requires a certain level of statistical and analytical skills. However, there can be a significant skill gap in many organizations, making it difficult to implement and maintain predictive analytics effectively.
Solution
To address this challenge, organizations can invest in training and development to build the necessary skills in-house. They can also consider partnering with external analytics service providers who can offer the required expertise and guidance. Furthermore, the advent of user-friendly predictive analytics tools has made it easier for non-technical users to conduct sophisticated predictive analysis.
Challenge 4:
Change Management
Implementing predictive analytics can represent a significant change in the way an organization conducts its sales forecasting. This can result in resistance from employees, especially those accustomed to traditional methods of sales forecasting.
Solution
Addressing this challenge involves a robust change management strategy. It starts with communicating the benefits of predictive analytics to all stakeholders. Training sessions can be conducted to help employees understand how predictive analytics works and how to interpret the results. It is also important to provide ongoing support to employees as they adapt to the new system.
Challenge 5:
Privacy and Security Concerns
With predictive analytics involving large amounts of data, often including sensitive customer information, privacy and security concerns can arise.
Solution
To mitigate these concerns, businesses should ensure they are complying with all relevant data protection regulations. They should also implement robust security measures to protect data from unauthorized access and cyber threats. Clear policies on data usage and access should be established and communicated to all stakeholders.
In conclusion, while challenges in implementing predictive analytics for sales forecasting are substantial, they are not insurmountable. By understanding these challenges and proactively addressing them, businesses can effectively leverage predictive analytics to improve their sales forecasting accuracy and efficiency.
Predictive analytics may have its challenges, but they are not insurmountable. With the right strategies in place, businesses can leverage predictive analytics to enhance their sales forecasting and gain a competitive advantage. But what does the future hold for predictive analytics in the realm of sales forecasting? Let’s turn our attention to this exciting prospect.
The Future of Predictive Analytics in Sales Forecasting
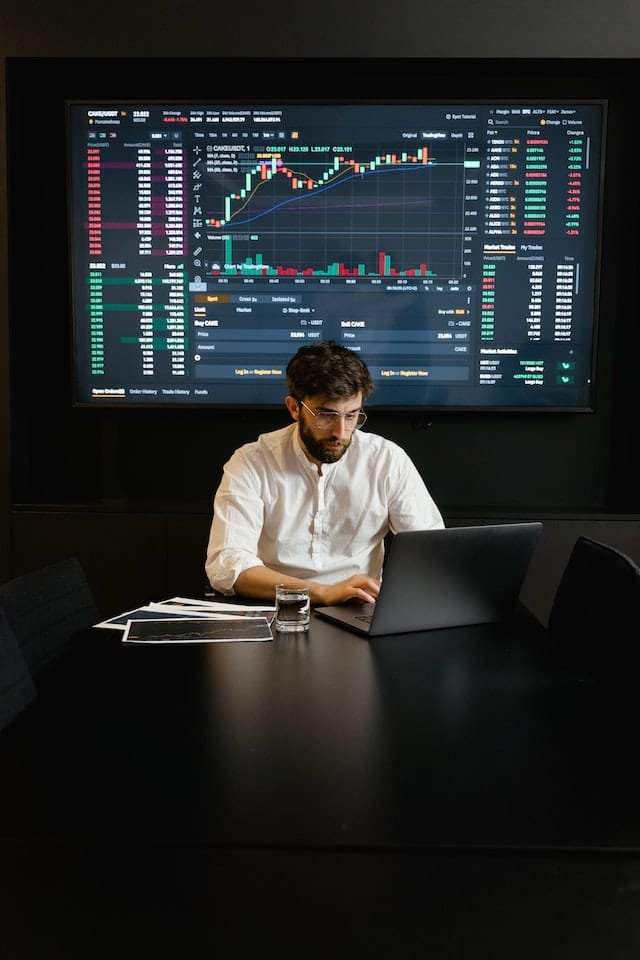
Predictive analytics in sales forecasting has come a long way, and its journey is far from over. As technology continues to evolve, so does the potential for predictive analytics in shaping the future of sales forecasting. This section will explore what that future might look like, by considering emerging trends, advancements in technology, and their potential influence on sales forecasting.
Integration of Advanced Machine Learning Techniques
As the field of machine learning continues to grow and advance, these techniques are becoming more integrated into predictive analytics. For instance, Deep Learning, an advanced form of Artificial Intelligence (AI) that imitates the human brain’s working mechanism, is expected to have a profound impact on sales forecasting. It can help uncover complex patterns and correlations in data that traditional algorithms might miss, increasing the accuracy of predictions.
Moreover, Reinforcement Learning, a type of machine learning where an agent learns to make decisions by interacting with its environment, holds promise for dynamic sales environments. It can adapt to changing conditions, making it especially useful in unpredictable markets.
Rise of Automated Predictive Analytics
The past decade has seen an impressive rise in the field of automated predictive analytics, particularly as it pertains to sales forecasting. The advancement in technologies such as artificial intelligence (AI) and machine learning (ML) have served as catalysts in this rise, allowing businesses to streamline their processes, reduce human error, and improve overall accuracy in their predictions.
Automation Meets Predictive Analytics
The fusion of automation and predictive analytics has been transformative. Automated predictive analytics involves the use of software that uses machine learning to automatically identify patterns and trends in datasets, then use these findings to predict future outcomes. This makes the process faster, more efficient, and often more accurate than traditional methods of predictive analytics.
Efficiency of Automated Predictive Analytics
One of the major advantages of automated predictive analytics is the incredible efficiency it brings to the table. Automated systems are capable of analyzing vast amounts of data at an exponentially faster rate than humans could manage. They are also capable of operating continuously, which allows for real-time data analysis and forecasting, a factor that has become increasingly important in today’s fast-paced business environment.
Accuracy and Consistency in Predictions
Automated predictive analytics systems also offer a higher level of consistency and accuracy in their predictions. Since these systems are based on machine learning algorithms, they have the ability to learn from past mistakes, improve upon them, and adapt to new information. This ability to learn and adapt ensures that these systems become more accurate and reliable over time.
Reducing Human Error
Another significant benefit of automated predictive analytics is the reduction of human error. Human analysis can often lead to mistakes due to bias, miscalculations, or simple oversights. Automated systems, on the other hand, offer an objective analysis of the data, significantly reducing the possibility of errors and improving the reliability of the results.
Integration with Other Technologies
Automated predictive analytics also integrate well with other technologies. For example, they can be combined with AI-powered CRM systems, enabling businesses to leverage customer data for more accurate sales forecasts. They can also be incorporated into ERP systems to help businesses streamline their operations and improve their efficiency.
While the rise of automated predictive analytics has been significant, it’s also worth noting that it’s not a replacement for human insight. Rather, it should be viewed as a tool that can enhance human capabilities. The insights provided by these automated systems can inform decision-making, but they should be complemented by human experience and intuition.
Automated predictive analytics is a powerful tool in sales forecasting. Its ability to analyze large volumes of data quickly and accurately, learn from past trends, and adapt to new information makes it a valuable asset for businesses looking to gain a competitive edge. As technology continues to advance, the role of automated predictive analytics in sales forecasting is likely to grow even more.
Emphasis on Real-time Forecasting
In the modern business world, where the market dynamics are in constant flux, traditional static sales forecasts can become obsolete very quickly. This is where the concept of real-time forecasting comes into play. This section provides a deep dive into the emphasis on real-time forecasting in predictive analytics and how it revolutionizes sales forecasting.
The Need for Real-time Forecasting
In today’s fast-paced business environment, companies need to react quickly to changes in customer behavior, market trends, and competitive dynamics. A sales forecast that was accurate a month or even a week ago may no longer reflect the current reality. Real-time forecasting addresses this issue by providing up-to-date forecasts based on the most recent data.
Real-time forecasting enables businesses to adjust their sales strategies and operations on the fly, helping them to seize new opportunities and mitigate risks. This is particularly valuable in industries with high volatility or seasonality, where sales can fluctuate significantly in a short period.
How Predictive Analytics Facilitates Real-time Forecasting
Predictive analytics plays a crucial role in facilitating real-time forecasting. With the help of advanced data processing and machine learning algorithms, predictive analytics can automatically analyze the latest data and update the sales forecasts in real time.
The key to real-time forecasting with predictive analytics is the ability to process data in real time. This requires robust data infrastructure and efficient algorithms that can handle large volumes of data and deliver fast results. Predictive analytics platforms often include features for real-time data streaming, in-memory computing, and parallel processing to support real-time forecasting.
The Benefits of Real-time Forecasting
Real-time forecasting offers several significant benefits for businesses. Firstly, it provides timely insights into sales trends, allowing businesses to make proactive decisions instead of reacting to outdated information. This can result in more effective sales strategies and higher sales performance.
Secondly, real-time forecasting enhances the accuracy of sales forecasts by taking into account the latest information. This can reduce the risk of overstocking or understocking, leading to improved inventory management and cost savings.
Finally, real-time forecasting facilitates better customer service by enabling businesses to anticipate customer demand and adjust their product offerings and service levels accordingly. This can enhance customer satisfaction and loyalty, leading to increased sales and customer retention.
Overcoming the Challenges of Real-time Forecasting
While real-time forecasting offers significant benefits, it also poses some challenges. These include the need for high-quality, real-time data, the computational demands of real-time data processing and analysis, and the complexity of managing and maintaining real-time forecasting systems.
However, these challenges can be overcome with the right approach. Ensuring access to reliable real-time data sources, investing in powerful computing resources, and leveraging advanced predictive analytics tools and techniques can enable successful real-time forecasting.
Utilization of Unstructured Data
As we delve into the advanced world of predictive analytics, one can no longer overlook the potential and benefits of unstructured data. Constituting around 80-90% of all digital data, unstructured data represents a goldmine of insights waiting to be harnessed.
In the context of sales forecasting, this data type takes on significant roles and applications.
Defining Unstructured Data
Unstructured data refers to information that is not organized or easily interpreted by traditional databases or data models. It includes formats like text, social media posts, emails, documents, video, audio, web pages, and more. In the business context, this could mean customer reviews, social media mentions, call logs, emails, or video testimonials.
Importance of Unstructured Data in Sales Forecasting
The significance of unstructured data in sales forecasting lies in its potential to provide deeper, more nuanced insights into customer behavior.
While structured data offers a clear picture of “what” is happening — i.e., sales numbers, customer demographics, transaction details — unstructured data can provide insights into the “why” behind these events. It can reveal customer sentiments, preferences, trends, and more, which are invaluable in making informed forecasts.
Processing and Analysis of Unstructured Data
Processing unstructured data involves several steps, including data mining, natural language processing (NLP), and machine learning (ML).
Data mining is used to extract useful information from large datasets, while NLP is employed to understand and analyze human language from text data. ML algorithms, particularly deep learning models, can handle data types such as images and videos.
These advanced analytics techniques can transform unstructured data into structured insights. They can identify patterns and trends that may not be evident in structured data, thereby adding depth and accuracy to sales forecasting.
Integrating Unstructured Data into Sales Forecasting
To integrate unstructured data into sales forecasting, organizations first need to determine which unstructured data sources are most relevant to their forecasting efforts. Next, they need to establish procedures for collecting and storing this data, ensuring it can be accessed and processed efficiently.
Then, using the techniques mentioned above, the unstructured data must be analyzed and converted into actionable insights. These insights should be combined with the insights derived from structured data to create a comprehensive sales forecast.
Challenges in Utilizing Unstructured Data
Despite its potential, unstructured data is not without its challenges. These include difficulty in data collection and storage, data privacy and security concerns, and the complexity of analyzing and interpreting unstructured data. However, with the advancement in big data technologies and the growing emphasis on data governance, these challenges are increasingly being addressed.
In conclusion, the utilization of unstructured data in sales forecasting is an evolving field that offers significant opportunities for businesses. By harnessing the insights hidden in unstructured data, organizations can enhance their forecasting accuracy and make more informed business decisions.
Impact of Explainable AI
The proliferation of artificial intelligence (AI) in predictive analytics has brought about considerable transformations in sales forecasting. A critical development in this arena is the advent of Explainable AI (XAI). XAI is a subset of AI that aims to make the decision-making process of AI models transparent, understandable, and interpretable to humans. The emergence of XAI has profound implications for predictive analytics in sales forecasting, and this section delves into these impacts.
Enhancing Trust in AI Models
Explainable AI fosters a trust environment between humans and AI models, which is crucial in the adoption and execution of AI-driven predictive analytics. Sales professionals often express skepticism about adopting AI models due to the ‘black box’ nature of these algorithms, making it hard to understand how they arrive at specific forecasts. XAI demystifies these models, offering clear insights into their decision-making processes, fostering trust, and encouraging wider acceptance.
Streamlining Regulatory Compliance
In industries where regulatory compliance is paramount, explainability becomes crucial. Sales forecasting models that use AI must comply with a host of privacy regulations, and in the case of a dispute, they must be able to justify their predictions. XAI provides a means to understand how the model uses data to arrive at a sales forecast, streamlining the compliance process.
Enabling Model Refinement
A significant advantage of XAI in predictive analytics is the ability to refine and improve models based on insights gleaned from the explainability process. When AI models can clearly indicate areas of weakness or bias in their forecasts, data scientists can modify these areas, leading to more accurate and robust predictive models.
Facilitating Effective Decision Making
Lastly, XAI’s ability to interpret AI-driven predictions enhances decision-making within sales teams. When sales professionals understand how a forecast is derived, they can make informed decisions about sales strategies. This deeper understanding aids in aligning AI-driven predictions with human intuition, resulting in more effective decision-making.
Wrapping Up
Predictive analytics has dramatically altered the landscape of sales forecasting, delivering precision, flexibility, and actionable insights into the hands of decision-makers. It has transitioned from being an esoteric concept to a vital cog in the sales machinery of numerous enterprises globally.
With a bird’s-eye view of the organization’s past sales performance, potential bottlenecks, and customer behavior patterns, predictive analytics lends itself to more informed decision-making. As businesses pivot towards a data-driven approach, this trend is only set to become more pronounced in the years to come.
And as Albert Einstein once said, “I never think of the future. It comes soon enough.” But with predictive analytics, businesses can not only think of the future, but they can also see it, plan for it, and conquer it.
Read Next:
- 3Ps in Marketing – The New Marketing Mix
- 25 Ways to Get More Views and Engagement on Your Instagram Reels (Fast!)
- 8 Content Amplification Tips to Maximize Lead Generation
- Lead Generation for B2B – In 12 Steps (Use this Strategy Now!)
- Skyrocket Your Email Outreach ROI! Strategies (+Experiments) By 19 Experts
- How You Can SkyRocket Your PPC Ad Campaign: 5 Fundamental Principles (Infographic)
- 70 Mistakes Startups Make And Tips On How You Can Avoid Them
Comments are closed.