In today’s fast-paced business environment, managing risks is more critical than ever. With the advent of artificial intelligence (AI) and predictive analytics, businesses now have powerful tools at their disposal to anticipate potential risks and make informed decisions to mitigate them. This article delves deep into how AI in predictive analytics can help businesses identify, assess, and manage risks effectively. By the end, you’ll have actionable strategies to implement AI-driven risk management in your organization.
Understanding AI in Predictive Analytics
What is Predictive Analytics?
Predictive analytics is the practice of using data, statistical algorithms, and machine learning techniques to identify the likelihood of future outcomes based on historical data. This approach allows businesses to make proactive decisions by anticipating future trends and events. For startup founders, mastering predictive analytics can provide a significant competitive advantage by enabling them to mitigate risks before they impact the business.
How AI Enhances Predictive Analytics
AI enhances predictive analytics by automating the analysis of large datasets and uncovering patterns and correlations that would be impossible to detect manually. Here’s how AI can significantly elevate the capabilities of predictive analytics for startups:
Scalability: AI can handle and process vast amounts of data at incredible speeds. For startups, this means being able to analyze data from various sources—such as customer interactions, market trends, and social media—without the need for extensive manual input. This scalability ensures that startups can make data-driven decisions quickly and efficiently.
Accuracy: Machine learning algorithms can learn from data and improve their predictions over time. By continuously feeding new data into AI models, startups can refine their predictive accuracy, leading to more reliable forecasts. This is particularly valuable in dynamic industries where conditions can change rapidly.
Pattern Recognition: AI excels at identifying patterns and correlations that may not be apparent to human analysts. For example, an AI model can analyze customer behavior data to identify subtle indicators that predict churn. By recognizing these patterns early, startups can implement retention strategies proactively.
Real-Time Insights: AI-driven predictive analytics can provide real-time insights, allowing startups to respond swiftly to emerging risks and opportunities. This agility is crucial in competitive markets where the ability to act quickly can be the difference between success and failure.
Strategic Implementation for Startup Founders
For startup founders, implementing AI-driven predictive analytics involves several strategic steps that ensure the technology delivers maximum value. Here are some actionable strategies to consider:
Identify Key Metrics: Determine the most critical metrics that impact your business. These could include customer acquisition costs, churn rates, revenue growth, and operational efficiency. Focusing on these key performance indicators (KPIs) will help you gather relevant data and build effective predictive models.
Invest in Data Infrastructure: A robust data infrastructure is essential for predictive analytics. Startups should invest in scalable data storage solutions, data integration platforms, and data processing tools. Cloud-based solutions offer flexibility and scalability, making them ideal for startups with evolving data needs.
Collaborate with Experts: Building and deploying AI models require specialized skills. Collaborate with data scientists, machine learning engineers, and domain experts to develop and refine your predictive models. If in-house expertise is limited, consider partnering with AI consultants or hiring freelance experts.
Develop a Data-Driven Culture: Encourage a culture where decisions are based on data and insights. Provide training and resources to help your team understand the value of predictive analytics and how to interpret AI-generated insights. This cultural shift ensures that predictive analytics is embraced and utilized effectively across your organization.
Focus on Actionable Insights: Predictive analytics is valuable only if it leads to actionable insights. Ensure that the output from your predictive models is presented in a way that is easy to understand and act upon. Develop user-friendly dashboards and reporting tools that translate complex data into clear, actionable recommendations for your team.
Overcoming Data Challenges
For AI-driven predictive analytics to be effective, startups must address several data-related challenges:
Data Quality: The accuracy of predictive models depends heavily on the quality of the data. Ensure that your data is clean, accurate, and comprehensive. Implement data governance practices to maintain high standards of data quality, including regular audits, data cleaning processes, and strict data management protocols.
Data Integration: Integrating data from various sources can be challenging but is crucial for comprehensive predictive analytics. Use data integration tools to combine data from internal systems, third-party sources, and social media. A unified data repository ensures that your AI models have access to all relevant information.
Data Privacy and Security: Handling sensitive data requires strict adherence to privacy regulations and security standards. Implement robust data security measures, such as encryption, access controls, and regular security audits. Ensure compliance with regulations like GDPR and CCPA to protect customer data and build trust.
Leveraging AI for Competitive Advantage
For startup founders, leveraging AI-driven predictive analytics can provide a significant competitive advantage. Here’s how:
Anticipating Market Trends: AI can analyze market data to predict emerging trends, enabling startups to stay ahead of the competition. For example, AI can identify shifts in consumer preferences, allowing businesses to adapt their products and marketing strategies proactively.
Optimizing Operations: Predictive analytics can identify inefficiencies in business operations and suggest improvements. For instance, AI can optimize inventory management by predicting demand fluctuations, reducing both excess stock and stockouts. This leads to cost savings and improved customer satisfaction.
Enhancing Customer Experience: AI can analyze customer data to provide personalized experiences that drive engagement and loyalty. By predicting customer needs and preferences, startups can tailor their offerings and communication strategies to meet individual customer expectations.
Mitigating Risks: AI-driven predictive analytics helps startups identify potential risks early and develop strategies to mitigate them. Whether it’s predicting equipment failures, detecting fraudulent activities, or anticipating market downturns, AI provides the insights needed to take proactive measures.
Real-World Examples
To understand the practical applications of AI-driven predictive analytics, consider how leading companies leverage this technology:
Netflix: Netflix uses predictive analytics to recommend content to its users. By analyzing viewing habits and preferences, Netflix can predict which shows and movies individual users are likely to enjoy, enhancing the user experience and reducing churn.
Walmart: Walmart uses AI to optimize its supply chain operations. Predictive models analyze sales data, weather patterns, and market trends to forecast demand and manage inventory levels effectively. This ensures that products are available when and where customers need them, improving sales and customer satisfaction.
UPS: UPS leverages predictive analytics to optimize its delivery routes. By analyzing data from GPS devices, traffic patterns, and delivery schedules, AI can predict the most efficient routes for drivers, reducing fuel consumption and improving delivery times.
Actionable Steps for Implementation
For startup founders ready to implement AI-driven predictive analytics, here are some actionable steps:
Start with a Pilot Project: Begin with a small-scale pilot project to test the effectiveness of predictive analytics in a specific area, such as customer retention or inventory management. Use the insights gained from the pilot to refine your approach and scale up to other areas.
Invest in Training: Ensure that your team has the skills needed to leverage predictive analytics effectively. Provide training on data analysis, machine learning, and the use of AI tools. Encourage continuous learning to keep up with advancements in technology.
Measure and Iterate: Continuously measure the performance of your predictive models and make necessary adjustments. Use key performance indicators (KPIs) to track the success of your AI initiatives and iterate based on feedback and new data.
Partner with Technology Providers: Work with AI technology providers to access the latest tools and expertise. Cloud-based AI platforms offer a range of services that can accelerate your implementation and provide scalable solutions for your growing data needs.
Benefits of AI in Predictive Risk Management
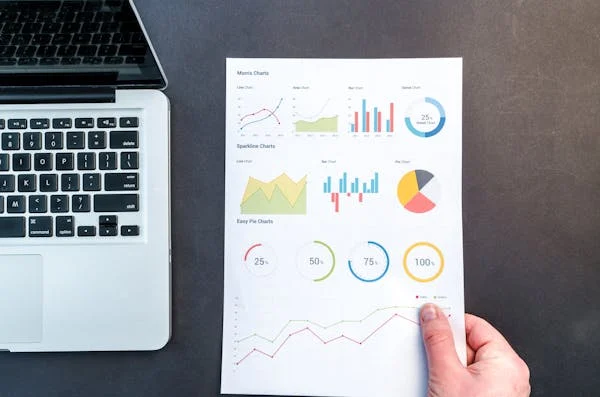
Proactive Risk Identification
One of the most significant benefits of AI in predictive risk management is the ability to identify risks proactively. By analyzing historical data and real-time information, AI can forecast potential risks and provide early warnings. This allows businesses to address issues before they escalate, reducing the impact on operations.
For instance, AI can predict equipment failures in manufacturing by analyzing sensor data and historical maintenance records. This enables maintenance teams to perform preventive maintenance, avoiding costly breakdowns and downtime.
Enhanced Decision-Making
AI-driven predictive analytics provides actionable insights that enhance decision-making. By offering a clear view of potential risks and their impacts, AI helps business leaders make informed decisions quickly. This is particularly valuable in dynamic environments where timely decisions are crucial.
For example, in financial services, AI can analyze market trends and economic indicators to predict market volatility. This allows investment managers to adjust their portfolios proactively, mitigating potential losses.
Cost Savings
By identifying risks early and taking preventive measures, businesses can save significant costs. AI-driven predictive analytics helps avoid expensive disruptions, reduce operational inefficiencies, and minimize losses.
In supply chain management, for example, AI can predict delays caused by factors such as weather conditions, political instability, or supplier issues. By anticipating these disruptions, companies can develop contingency plans, re-route shipments, or find alternative suppliers, saving time and money.
Improved Compliance
Compliance with regulations is a critical aspect of risk management. AI can help businesses ensure compliance by monitoring data and identifying potential compliance risks. This is particularly important in highly regulated industries such as finance, healthcare, and manufacturing.
For example, AI can analyze transactions in real-time to detect suspicious activities and potential money laundering, helping financial institutions comply with regulatory requirements. Similarly, in healthcare, AI can ensure that patient data handling complies with privacy regulations.
Implementing AI-Driven Predictive Risk Management
Assessing Your Data Needs
Before implementing AI-driven predictive analytics, it’s essential to assess your data needs. Determine what types of data are most relevant to your risk management objectives. This could include operational data, financial data, customer data, and external data such as market trends and social media activity.
Conduct a data audit to identify the sources and quality of your existing data. Ensure that your data is clean, accurate, and well-organized. This may involve removing duplicates, handling missing values, and standardizing data formats. A solid data foundation is crucial for building effective AI models.
Choosing the Right AI Tools
Selecting the right AI tools is critical for successfully implementing AI-driven predictive analytics. There are numerous AI platforms and tools available, each offering different features and capabilities. Look for tools that can handle large datasets, support various data types, and provide robust analytical capabilities.
Consider cloud-based AI solutions such as Google Cloud AI, IBM Watson, and Microsoft Azure AI, which offer scalability and flexibility. These platforms provide the infrastructure needed to process and analyze vast amounts of data efficiently. Evaluate each platform based on factors such as ease of integration with your existing systems, user-friendly interfaces, and support for compliance with industry regulations.
Building and Training AI Models
Once your data is prepared and you’ve chosen the right tools, the next step is to build and train your AI models. This involves selecting appropriate machine learning algorithms and feeding them with historical data to identify patterns and make predictions.
Define clear objectives for your AI models. For example, you might want to predict equipment failures, identify potential fraud, or forecast supply chain disruptions. Use a portion of your data to train the model and another portion to test its accuracy. Continuously refine and improve the model to ensure it remains effective and relevant.
Collaboration with data scientists and risk management professionals is crucial during this phase. Data scientists can provide expertise in selecting and tuning machine learning algorithms, while risk management professionals can offer insights into the practical applications and relevance of the predictions.
Integrating AI into Risk Management Processes
After building and training your AI models, the next step is to integrate them into your risk management processes. This involves embedding AI insights into your daily operations, making them easily accessible to risk managers and decision-makers.
Develop user-friendly dashboards and reporting tools that present AI-generated insights in a clear and actionable manner. Ensure that risk managers and decision-makers are trained to interpret and use these insights effectively. This might involve regular workshops, training sessions, and ongoing support to help them adapt to new tools and processes.
Monitoring and Continuous Improvement
The implementation of AI-driven predictive analytics is an ongoing process. Continuous monitoring and improvement are essential to ensure that your AI models remain accurate and relevant.
Establish key performance indicators (KPIs) to track the success of your AI initiatives. Common KPIs might include risk mitigation effectiveness, cost savings, and compliance rates. Regularly review these metrics to identify areas for improvement and make necessary adjustments to your models and processes.
Create a feedback loop where insights from AI are continuously fed back into the system to refine and enhance the models. This iterative process helps your AI capabilities evolve with changing market dynamics and business needs.
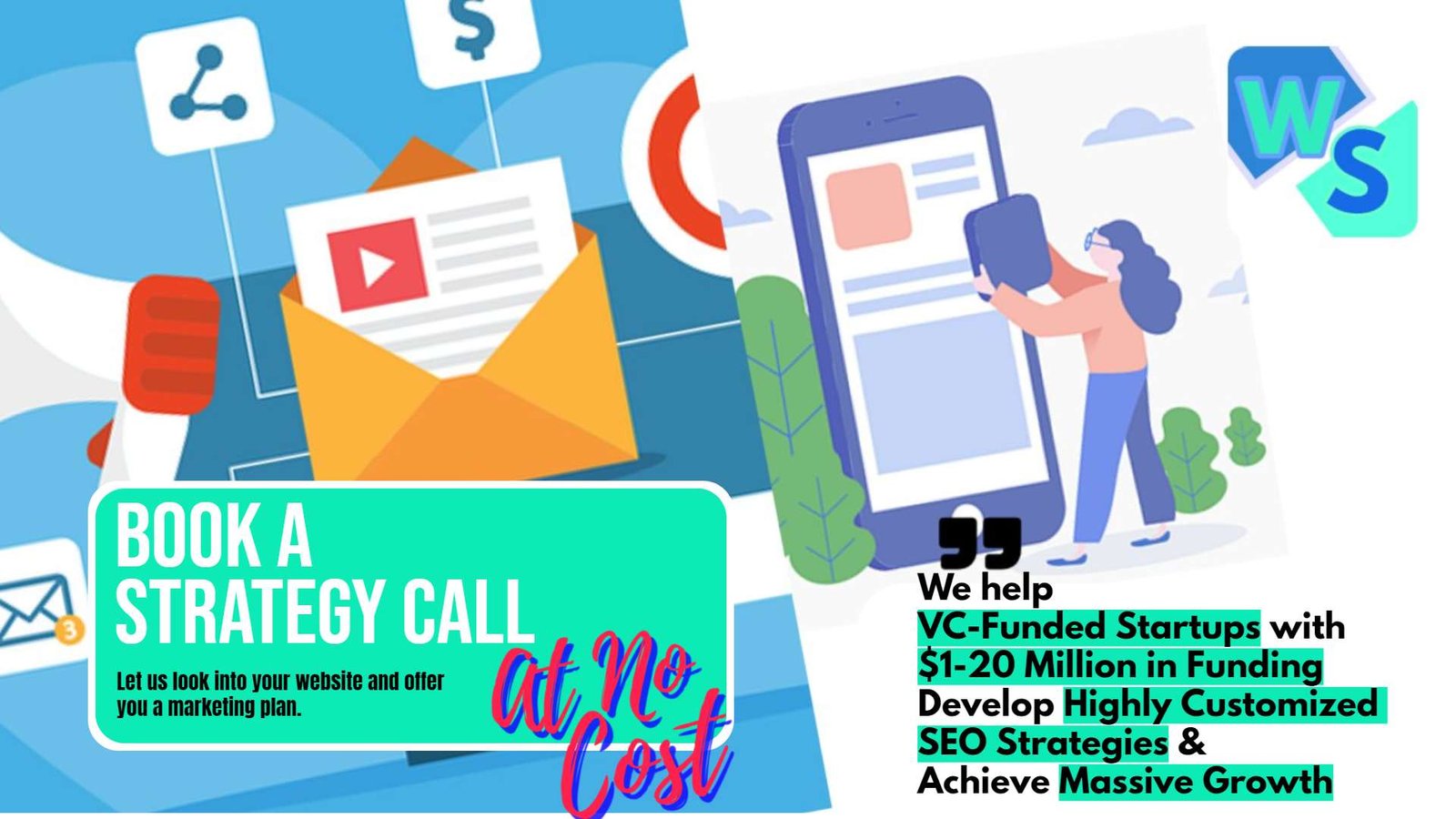
Related: Check out our free tools:
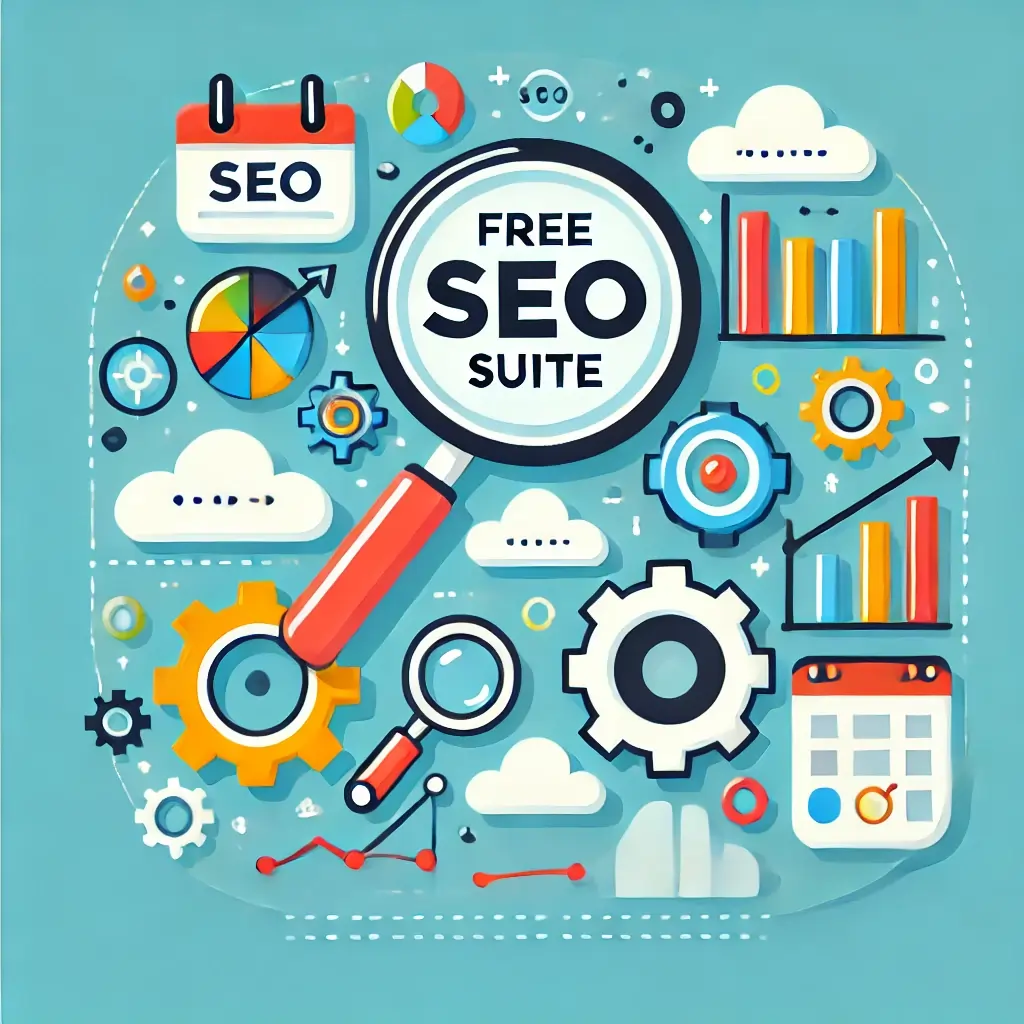
Real-World Applications of AI in Predictive Risk Management
Financial Services: Fraud Detection
In the financial services sector, fraud detection is a critical component of risk management. AI-driven predictive analytics can analyze transaction data in real-time to identify suspicious activities that may indicate fraud. By leveraging machine learning algorithms, financial institutions can detect patterns and anomalies that are often missed by traditional methods.
For instance, AI can monitor thousands of transactions per second, identifying unusual spending patterns, sudden large transactions, or activities that deviate from a user’s typical behavior.
Once a potential fraud is detected, the system can automatically alert risk managers or even take immediate action, such as freezing the account or flagging the transaction for further investigation. This proactive approach significantly reduces the risk of financial losses and enhances customer trust.
Manufacturing: Predictive Maintenance
In the manufacturing industry, equipment failure can lead to significant downtime and financial losses. Predictive maintenance, powered by AI, helps manufacturers anticipate when equipment is likely to fail and schedule maintenance before issues arise. This approach not only prevents unexpected breakdowns but also extends the lifespan of machinery.
For example, AI algorithms can analyze data from sensors embedded in manufacturing equipment to monitor parameters such as temperature, vibration, and pressure. By identifying patterns that precede equipment failure, AI can predict when maintenance is needed. This allows maintenance teams to perform necessary repairs during scheduled downtimes, minimizing disruption to production and reducing maintenance costs.
Healthcare: Patient Risk Assessment
In healthcare, predictive analytics plays a crucial role in assessing patient risks and improving outcomes. AI can analyze patient data, including medical history, genetic information, and lifestyle factors, to predict the likelihood of various health conditions. This enables healthcare providers to develop personalized treatment plans and take preventive measures.
For instance, AI can help identify patients at high risk of developing chronic diseases such as diabetes or heart disease. By analyzing data from electronic health records (EHRs), wearable devices, and patient surveys, AI can provide early warnings and suggest interventions. This proactive approach not only improves patient outcomes but also reduces healthcare costs by preventing complications and hospitalizations.
Retail: Inventory Management
In the retail industry, managing inventory effectively is essential for meeting customer demand and minimizing costs. AI-driven predictive analytics can forecast demand for different products, helping retailers optimize their inventory levels and reduce the risk of overstocking or stockouts.
For example, AI can analyze historical sales data, seasonal trends, and external factors such as weather and economic conditions to predict future demand. Retailers can use these insights to adjust their inventory levels, ensuring that popular products are always available while minimizing excess stock. This not only improves customer satisfaction but also enhances operational efficiency and profitability.
Energy: Predicting Supply and Demand
In the energy sector, balancing supply and demand is a complex challenge. AI-driven predictive analytics can help energy companies forecast demand more accurately and optimize their supply strategies. This is particularly important in the context of renewable energy, where supply can be variable.
For instance, AI can analyze data from weather forecasts, historical energy consumption patterns, and market trends to predict energy demand. This allows energy providers to adjust their production schedules and manage their resources more effectively.
Additionally, AI can help identify potential disruptions in the supply chain, such as equipment failures or geopolitical events, allowing companies to take preventive measures and ensure a reliable energy supply.
Logistics: Route Optimization
In the logistics industry, efficient route planning is crucial for reducing costs and ensuring timely deliveries. AI-driven predictive analytics can optimize delivery routes by analyzing data such as traffic patterns, weather conditions, and delivery volumes.
For example, AI can predict traffic congestion and suggest alternative routes to avoid delays. It can also analyze historical delivery data to identify the most efficient routes for different times of the day. By optimizing routes, logistics companies can reduce fuel consumption, improve delivery times, and enhance customer satisfaction.
Overcoming Challenges in AI-Driven Predictive Analytics
Ensuring Data Privacy and Security
One of the main challenges in implementing AI-driven predictive analytics is ensuring data privacy and security. Businesses must handle large volumes of sensitive data, including customer information and proprietary business data. Ensuring compliance with data protection regulations like GDPR and CCPA is crucial.
Implement robust data security measures, such as encryption, access controls, and regular security audits. Ensure that your AI tools and platforms comply with relevant regulations and have built-in security features. Being transparent with customers about how their data is used and protected can also help build trust and mitigate privacy concerns.
Addressing Bias in AI Models
AI models can sometimes exhibit biases that reflect the biases present in the training data. This can lead to unfair or discriminatory outcomes, particularly in areas such as hiring, lending, and law enforcement. Addressing bias in AI models is essential for ensuring fairness and ethical use of AI.
Implement bias detection and mitigation techniques during the model development process. This includes analyzing the training data for potential biases, using techniques such as fairness constraints to reduce bias in the models, and regularly auditing the models to ensure they remain fair over time. Involving diverse teams in the development and review process can also help identify and address potential biases.
Managing Model Interpretability
Advanced AI models, such as deep learning neural networks, can be highly accurate but often lack interpretability. This can make it difficult for business users to understand how the models arrive at their predictions and make it challenging to trust and act on the insights.
Focus on developing interpretable models where possible, and use techniques such as LIME (Local Interpretable Model-agnostic Explanations) or SHAP (SHapley Additive exPlanations) to explain complex model predictions. Providing clear, interpretable insights helps build trust and enables business users to make informed decisions based on the AI-generated predictions.
Ensuring Seamless Integration
Integrating AI tools into existing business workflows can be challenging, especially for businesses with established processes and systems. Ensuring seamless integration and minimal disruption is essential for successful adoption.
Work closely with your IT team or a dedicated vendor to ensure smooth integration. Test the integrations thoroughly to make sure data is being accurately captured and analyzed. Develop user-friendly interfaces that allow business users to access and act on AI-generated insights easily. Provide ongoing support and training to help your team adapt to new tools and processes.
Future Trends in AI-Driven Predictive Analytics
Real-Time Analytics
As technology advances, real-time analytics will become increasingly important. Businesses will be able to analyze data as it is generated, providing immediate insights and enabling rapid decision-making. This capability is particularly valuable in dynamic environments where timely responses are crucial.
For instance, in finance, real-time analytics can help identify market trends and trading opportunities as they emerge. In manufacturing, real-time monitoring of equipment can detect issues early and prevent costly downtime. By leveraging real-time analytics, businesses can stay agile and responsive to changing conditions.
Advanced Personalization
Personalization is becoming increasingly sophisticated thanks to AI. The future will see even more advanced levels of personalization, where AI not only tailors marketing messages but also customizes the entire customer journey based on individual preferences and behaviors.
For example, AI can analyze a customer’s browsing history, purchase behavior, and even social media activity to create a unique shopping experience. This might include personalized product recommendations, customized landing pages, and targeted promotions. For businesses, this means higher engagement, improved customer satisfaction, and increased sales.
Integration with IoT
The integration of AI with the Internet of Things (IoT) is set to revolutionize predictive analytics. IoT devices generate vast amounts of data that can be analyzed by AI to provide deeper insights into customer behavior and operational efficiency.
For example, in the retail sector, IoT sensors can track foot traffic patterns within a store. AI can analyze this data to optimize store layouts, product placements, and staffing levels. In manufacturing, IoT devices can monitor machinery performance in real-time, and AI can predict maintenance needs before failures occur, reducing downtime and improving productivity.
Ethical AI and Transparency
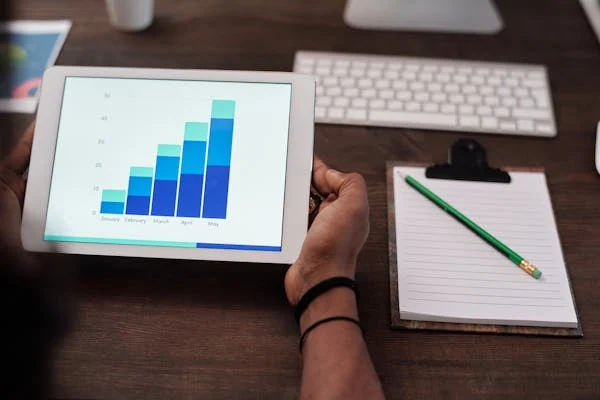
As AI becomes more pervasive, there will be a greater focus on ethical AI and transparency. Consumers and regulators are increasingly concerned about how data is used and the potential biases in AI models. Businesses will need to ensure their AI practices are transparent, fair, and ethical.
Implementing ethical AI involves regular audits of AI models to detect and mitigate biases, ensuring transparency about how data is collected and used, and complying with all relevant regulations. By prioritizing ethical AI, businesses can build trust with customers and stakeholders, which is essential for long-term success.
Predictive Maintenance and Operational Efficiency
Predictive maintenance, driven by AI, is transforming industries by improving operational efficiency and reducing costs. AI analyzes data from machinery and equipment to predict when maintenance is needed, preventing unexpected breakdowns and extending the lifespan of assets.
For instance, in the logistics industry, AI can analyze data from vehicle sensors to predict maintenance needs, schedule repairs proactively, and minimize downtime. This leads to more efficient fleet management, reduced operational costs, and improved service reliability. Businesses adopting predictive maintenance can achieve significant cost savings and operational efficiencies.
Conclusion
AI-driven predictive analytics offers startup founders a powerful tool to anticipate risks, optimize operations, and drive informed decision-making.
By leveraging AI, businesses can transform vast amounts of data into actionable insights, enabling proactive strategies that mitigate risks before they impact the bottom line. The ability to predict future trends and potential issues empowers startups to stay ahead in a competitive market, ensuring sustained growth and success.
Read Next:
- Facebook Marketing: Essential Statistics for 2024
- Harnessing Data-Driven Marketing: Key Statistics for 2024
- Applying Bayesian Statistics to Marketing: Key Insights for 2024
- Key Marketing Statistics to Drive Your Strategy in 2024
- Omni-Channel Marketing Statistics for 2024
Comments are closed.