User experience (UX) research is at the heart of great design. It’s how designers and teams understand what users want, what frustrates them, and what makes them stick around. But traditional UX research can be time-consuming and resource-heavy. That’s where artificial intelligence (AI) steps in to change the game.
AI doesn’t just speed up UX research—it transforms it. By processing vast amounts of data, identifying patterns, and offering predictive insights, AI helps teams make smarter, user-focused design decisions faster. But how do you actually integrate AI into your UX research process?
In this article, we’ll explore how AI can supercharge your UX research, the tools that make it possible, and the steps to ensure it aligns with your design goals.
Step 1: Understanding the Role of AI in UX Research
At its core, AI is about automating and enhancing processes that are repetitive, time-intensive, or prone to human error. In UX research, AI can analyze user behavior, predict preferences, and even simulate user interactions, saving teams countless hours.
What AI Brings to UX Research
For example, instead of manually reviewing hundreds of session recordings to spot patterns, an AI tool can identify common bottlenecks in navigation or highlight where users drop off during a task.
AI doesn’t replace the human touch in UX—it amplifies it. While AI excels at crunching data, it’s still up to designers and researchers to interpret the results and create experiences that resonate emotionally.
Key Areas Where AI Can Help
AI adds value in several areas of UX research, including:
- Data Analysis: Processing large datasets to find patterns and trends.
- Behavior Prediction: Anticipating user actions based on historical data.
- Automated Testing: Running usability tests or A/B tests with minimal manual input.
- Voice of Customer Insights: Analyzing feedback from surveys, reviews, and support tickets to understand user sentiment.
These capabilities allow teams to focus on strategy and creativity rather than being bogged down by manual tasks.
Step 2: Using AI for Data Collection and Analysis
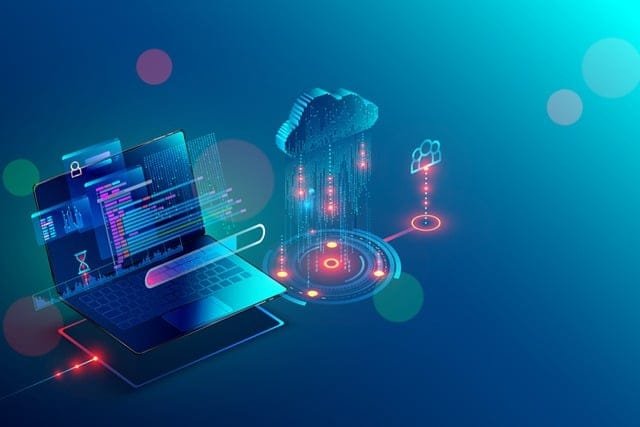
AI tools excel at collecting and analyzing user behavior data from websites, apps, and other digital platforms. Heatmaps, click paths, and session recordings can now be processed at scale to reveal patterns.
Gathering Behavioral Data
For instance, an AI-powered tool like Hotjar or Crazy Egg can analyze thousands of user sessions to identify where users click, scroll, or hesitate. It doesn’t just tell you what’s happening—it highlights why certain areas of your design might be causing confusion.
Analyzing Text Feedback
Feedback forms, customer reviews, and support tickets are goldmines of user insights, but sifting through them manually can be overwhelming. AI-powered natural language processing (NLP) tools, like MonkeyLearn or IBM Watson, can analyze text data to extract key themes, sentiments, and user pain points.
For example, an AI tool could scan hundreds of user reviews and identify that “slow load times” are a recurring complaint, giving you actionable insights in minutes.
Segmenting Your Audience
Understanding different user segments is crucial for personalized design. AI can analyze user demographics, behaviors, and preferences to create detailed personas. Tools like Mixpanel or Amplitude use machine learning to group users based on shared characteristics or actions, making it easier to tailor your design to specific needs.
Step 3: Enhancing Usability Testing with AI
Traditional usability testing often involves recruiting participants, setting up sessions, and manually analyzing results—a time-consuming process. AI tools can automate much of this. Platforms like UserTesting now use AI to analyze test session videos, flagging moments where users experience frustration or confusion.
Automating Usability Testing
For example, AI might identify instances where users hover over a button but don’t click, signaling hesitation or uncertainty about its purpose.
Simulating User Behavior
AI can simulate user interactions with your design, providing insights even before real users engage with it. Predictive tools like EyeQuant analyze your interface to predict where users’ attention will go, helping you optimize layouts and calls to action.
This approach allows you to test and refine your design faster, reducing the number of iterations needed during development.
Speeding Up Iteration Cycles
With AI providing instant feedback on usability, you can quickly iterate on designs without waiting weeks for user testing results. For instance, an AI tool might suggest changes to a confusing navigation menu in real-time, allowing you to implement and test a revised version immediately.
Step 4: Improving Personalization with AI
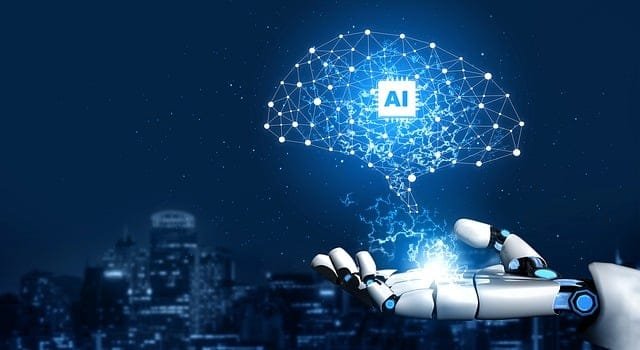
Personalization is no longer a “nice-to-have”—it’s an expectation. AI-driven UX research enables you to create experiences tailored to individual users based on their preferences, behavior, and past interactions.
Creating Tailored User Experiences
For example, Netflix uses AI to recommend shows based on viewing history, while e-commerce platforms suggest products users are likely to buy. These personalized experiences drive engagement and conversions.
Predicting User Needs
AI doesn’t just react to user behavior—it anticipates it. Predictive analytics can identify what users are likely to do next and help you design proactive solutions. For instance, if AI detects that users often abandon carts at the shipping page, you can redesign that page to address potential barriers, like unclear delivery times.
Balancing Personalization and Privacy
While personalization is powerful, it’s essential to respect user privacy. Use AI responsibly by being transparent about data collection and offering users control over their data. Building trust is just as important as creating tailored experiences.
Step 5: Streamlining A/B Testing with AI
A/B testing is a staple of UX research, but it can be slow and labor-intensive. AI speeds up the process by identifying which variations are most likely to succeed based on historical data. Platforms like Optimizely and VWO now use AI to optimize A/B testing by automatically adjusting traffic allocation to the better-performing variation.
Running Smarter Tests
For instance, if one variation starts outperforming the other early in the test, AI can shift more users to that version to maximize results faster.
Multivariate Testing Made Simple
Multivariate testing, which evaluates multiple design elements simultaneously, can be complex. AI simplifies this by analyzing the interactions between variables and determining the best combination. This approach is especially useful for optimizing complex interfaces with many interdependent elements.
Real-Time Adjustments
AI-powered testing tools can make real-time adjustments to your interface based on user behavior. For example, if a variation isn’t performing well, the tool might automatically replace it with a better option, ensuring you’re always delivering an optimized experience.
Step 6: Extracting Insights from User Sentiment
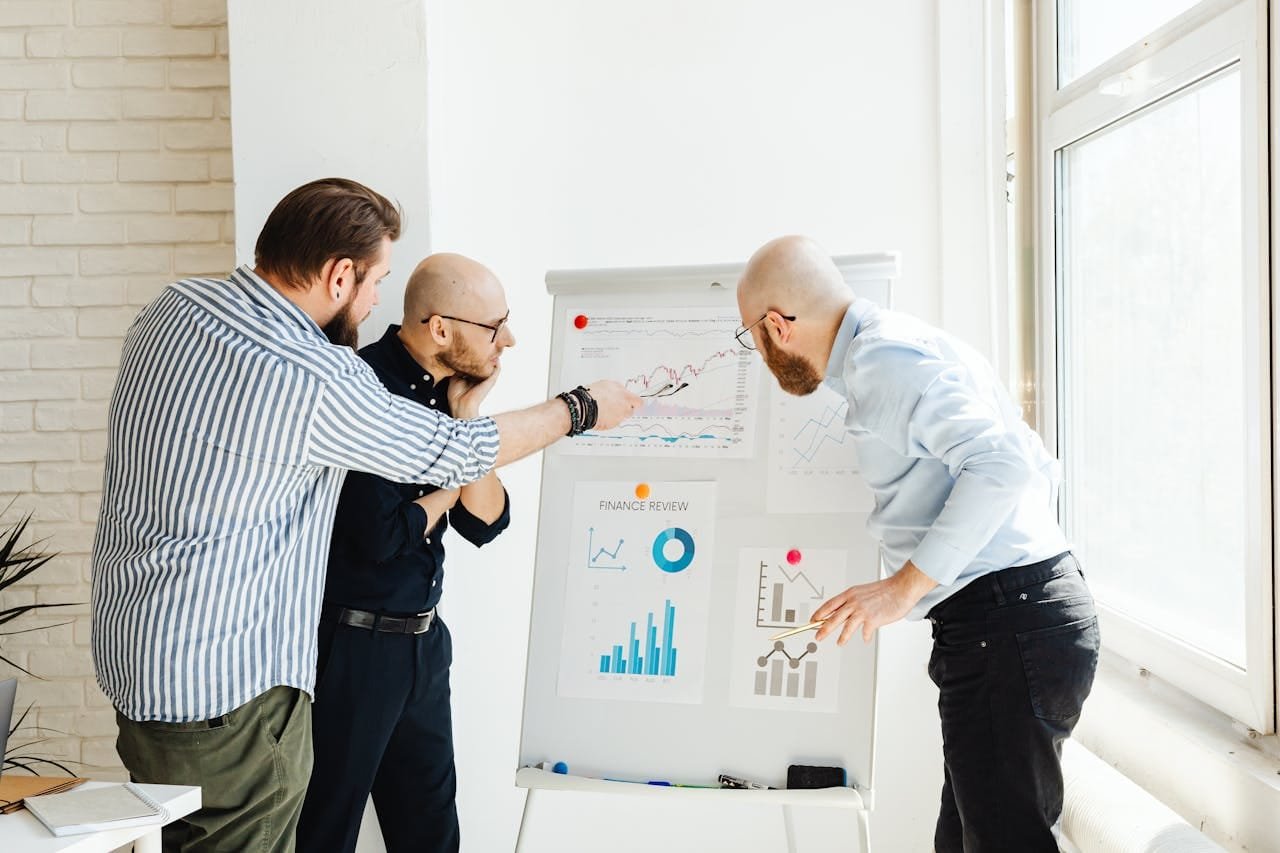
Understanding how users feel about your product is just as important as knowing what they do. AI-powered sentiment analysis tools can analyze user comments, reviews, and social media mentions to gauge overall sentiment.
Analyzing Sentiment in Real Time
For example, if users frequently describe your app as “frustrating,” AI can flag this as a concern and highlight the contexts in which the term appears.
Turning Sentiment into Actionable Feedback
Sentiment analysis isn’t just about tracking emotions—it’s about identifying patterns and opportunities for improvement. For instance, if sentiment analysis reveals that users love your app’s speed but dislike its navigation, you know where to focus your design efforts.
Tracking Changes Over Time
AI can track how sentiment evolves as you roll out updates or new features. This helps you measure the impact of your changes and ensures you’re consistently moving in the right direction.
Step 7: Addressing Bias in AI-Powered UX Research
AI is only as good as the data it’s trained on, and biased data can lead to skewed insights. For example, if your training data overrepresents certain demographics, your AI might make recommendations that don’t work for everyone.
Recognizing the Risk of Bias
Recognizing these risks is the first step in addressing them. Be mindful of where your data comes from and strive for diversity in your datasets.
Validating AI Insights
AI insights should always be validated by human researchers. If an AI tool suggests a design change, test it with real users to ensure it works as intended. Combining AI-driven insights with human intuition ensures a balanced approach.
Iterating on AI Models
AI models need regular updates to remain effective. As user behavior evolves, retrain your AI tools with fresh data to ensure they continue to provide accurate and relevant insights.
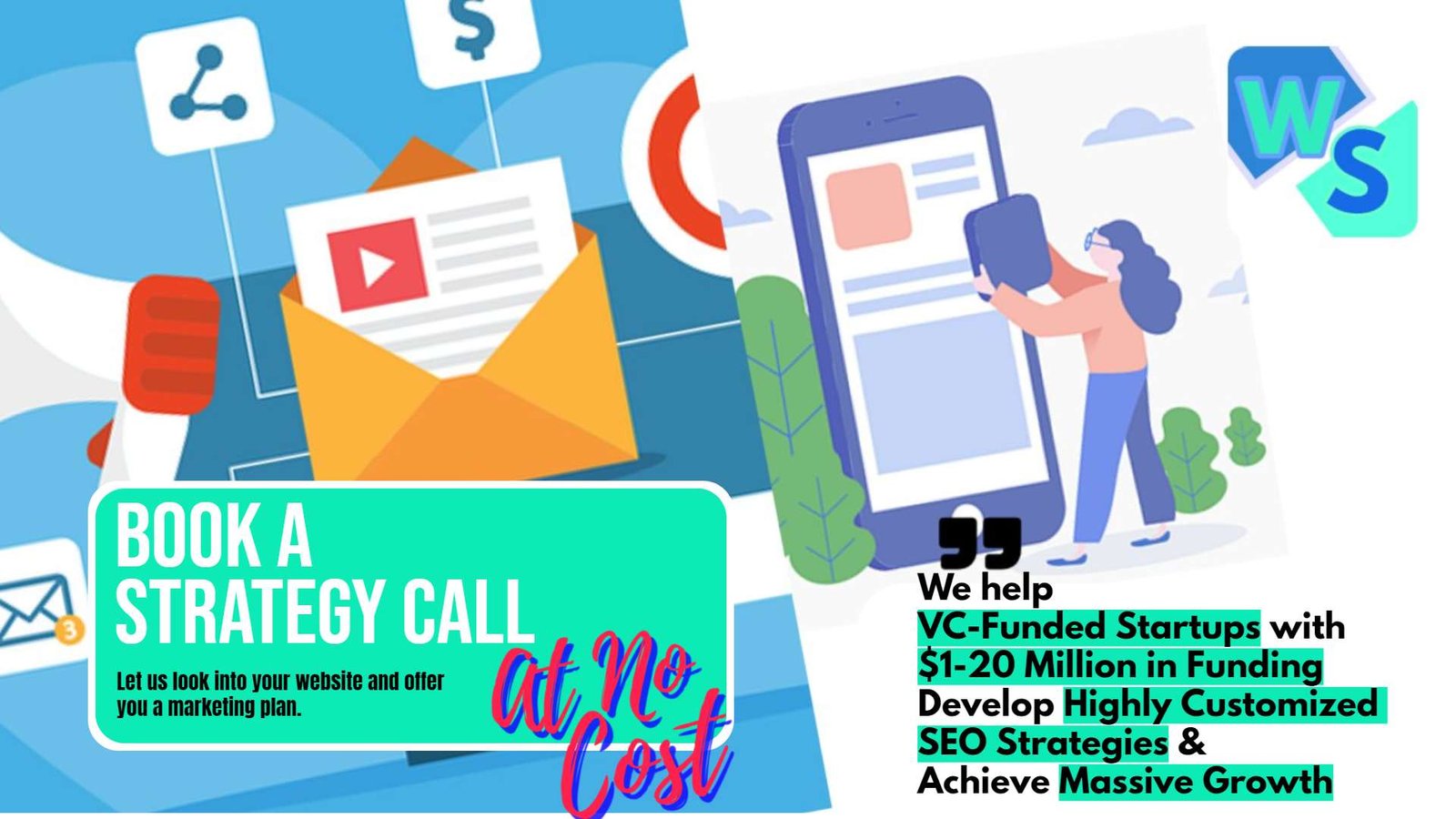
Related: Check out our free tools:
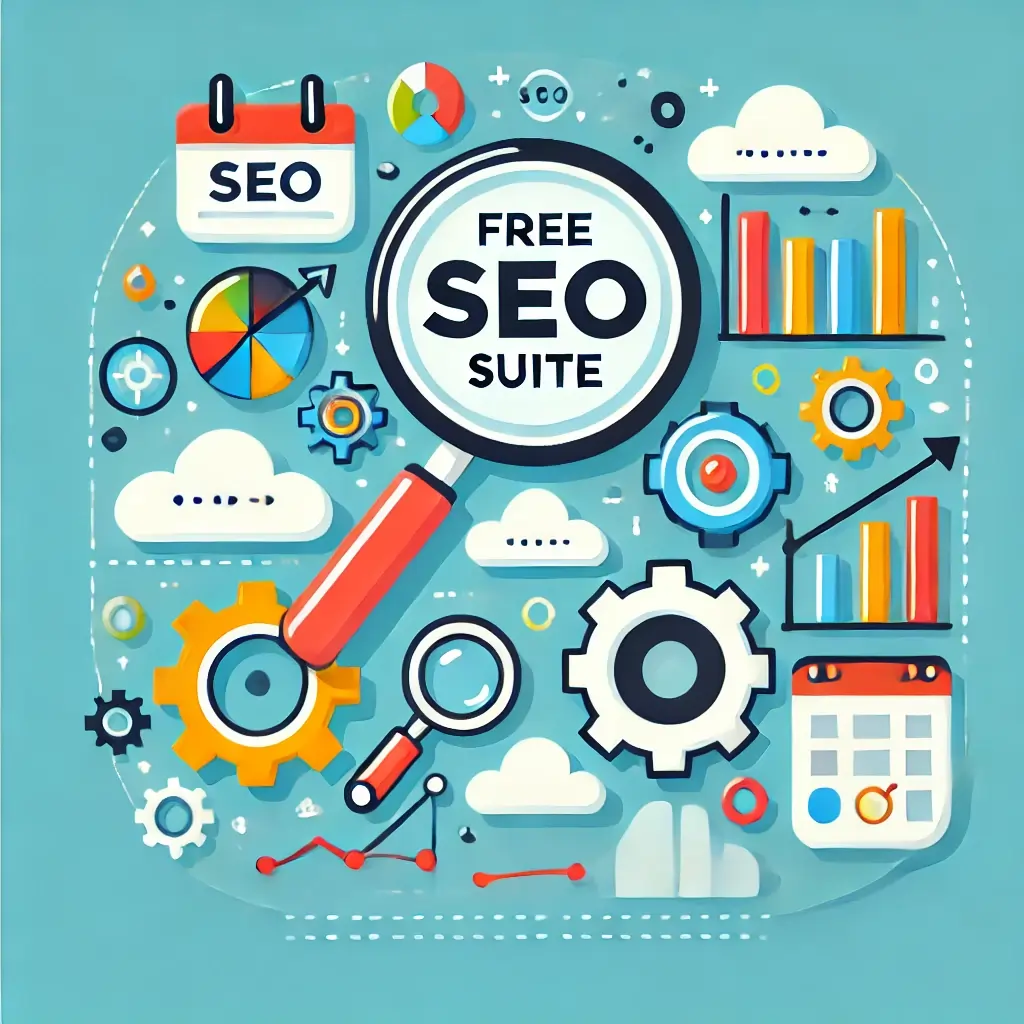
Step 8: Integrating AI into Your UX Workflow
Choosing the Right Tools
Not all AI tools are created equal. Choose platforms that align with your goals and integrate seamlessly into your existing workflow. For example, if you’re focused on user feedback, tools like Qualtrics with AI-driven insights might be ideal. For usability testing, consider platforms like Maze or Lookback with AI capabilities.
Training Your Team
AI tools are only as effective as the people using them. Invest in training your team to understand how AI works, interpret its insights, and integrate them into the design process. This ensures you’re leveraging AI to its full potential.
Creating a Feedback Loop
Integrate AI insights into a continuous feedback loop. Use the insights to refine your designs, then collect new data to measure the impact of those changes. This iterative approach ensures your designs are always improving.
Step 9: Overcoming Challenges in AI-Driven UX Research
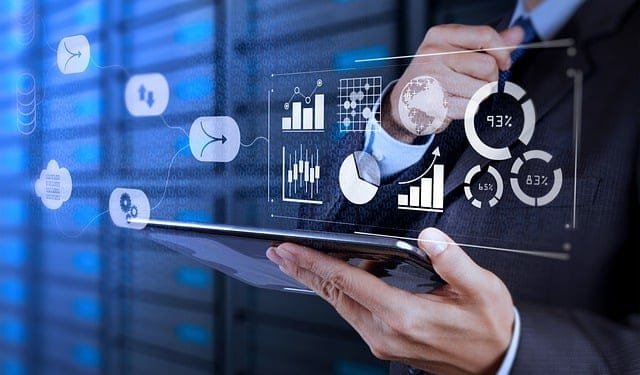
AI excels at processing large amounts of data, but it doesn’t fully replace the human touch in UX research. A common challenge is striking the right balance between automation and manual intervention. For instance, while AI can flag potential usability issues, it might not understand the emotional nuances behind user frustrations.
Balancing Automation with Human Oversight
To overcome this, use AI as a tool to enhance your insights, not dictate them. Pair AI-generated insights with qualitative methods like interviews and focus groups to ensure a well-rounded understanding of your users.
Interpreting Complex Outputs
AI tools often present results in technical or abstract formats, such as heatmaps, clustering diagrams, or predictive scores. For teams unfamiliar with these outputs, interpreting the data can be a challenge.
To tackle this, invest in tools with intuitive dashboards and visualizations. Train your team to understand AI outputs and how to convert them into actionable design decisions. The more accessible the insights, the easier it is to integrate them into your workflow.
Addressing Ethical Concerns
As AI processes sensitive user data, ethical considerations become paramount. Users may worry about how their data is collected, stored, and used. Transparency is key—inform users about your data practices and offer them control over their information.
For example, include clear privacy policies and give users the option to opt out of data collection. By prioritizing ethical AI practices, you build trust while leveraging AI responsibly.
Step 10: Measuring the Impact of AI on UX Design
AI’s impact on UX research should be measurable. Set clear benchmarks to evaluate how AI tools improve your workflow. For example, measure whether incorporating AI shortens research cycles, increases the volume of actionable insights, or boosts user engagement metrics.
Tracking Performance Improvements
Track metrics such as:
- Reduced time to identify usability issues.
- Increased conversion rates after implementing AI-driven design changes.
- Improved user satisfaction scores based on post-launch feedback.
These tangible results demonstrate the value AI adds to your process.
Comparing Before and After
One way to measure AI’s effectiveness is to compare outcomes before and after integrating AI into your workflow. For example, if manual usability testing previously identified 10 issues per test cycle, but AI now flags 25 issues with the same effort, the improvement is clear.
Additionally, analyze how quickly your team can act on insights. Faster iterations often translate to better user experiences and higher ROI.
Scaling Success Across Teams
Once you see positive results, share the success with other teams in your organization. For instance, if AI improves UX research for a specific app, explore how it can benefit your marketing, product development, or customer support teams. This cross-functional approach maximizes the value of your AI investments.
Step 11: Staying Ahead of AI Trends in UX Research
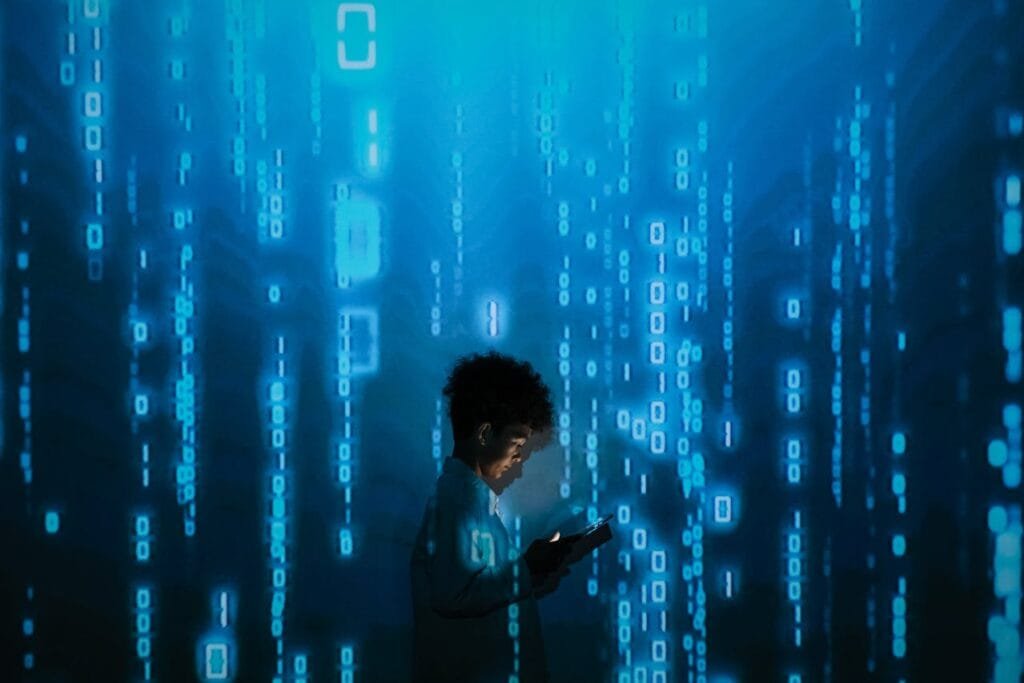
AI technology is advancing rapidly, and new tools are constantly emerging. Stay informed about the latest developments in AI-powered UX research tools. Subscribe to industry newsletters, attend webinars, and follow thought leaders in UX and AI to remain ahead of the curve.
Keep Up with Evolving AI Tools
For example, emerging tools might offer advanced sentiment analysis or real-time eye-tracking insights that weren’t available before. Experimenting with these tools can give you a competitive edge.
Explore Emerging Technologies
AI in UX research isn’t limited to data analysis. Technologies like augmented reality (AR), virtual reality (VR), and conversational AI are creating new opportunities for user testing and design exploration.
For instance, AI-powered AR tools can simulate how users interact with physical products in real-world environments, providing insights that traditional testing methods can’t. Similarly, conversational AI can facilitate user interviews or gather feedback through chatbots.
Foster a Culture of Experimentation
Encourage your team to experiment with AI tools and explore new ways to enhance your UX research process. Create a culture that values learning and innovation, where team members feel comfortable testing emerging technologies and sharing their findings.
Step 12: Building a Long-Term Strategy for AI-Driven UX Research
For AI to have a lasting impact, it needs to be fully integrated into your UX design framework. Build AI-driven research into every stage of your process, from discovery and ideation to testing and iteration.
Integrate AI into Your Design Framework
For example, use AI tools to analyze user needs during the discovery phase, predict behaviors during prototyping, and optimize designs based on testing results. Consistency in applying AI ensures its benefits compound over time.
Collaborate Across Disciplines
AI-driven UX research works best when it’s part of a collaborative effort. Involve designers, developers, data scientists, and product managers in the process. Each team brings unique perspectives that enhance the application of AI insights.
For instance, while data scientists can refine predictive models, designers can ensure those insights translate into user-friendly interfaces. Collaboration ensures that AI isn’t siloed but contributes meaningfully across teams.
Plan for Continuous Improvement
AI tools are not a one-and-done solution. To get the most value, plan for ongoing refinement and scaling. Regularly evaluate the performance of your AI tools, update them with new data, and explore additional features as your needs evolve.
For example, as your user base grows, your AI tools should adapt to analyze larger datasets, identify emerging trends, and uncover new opportunities.
Step 13: Case Studies of AI-Driven UX Research Success
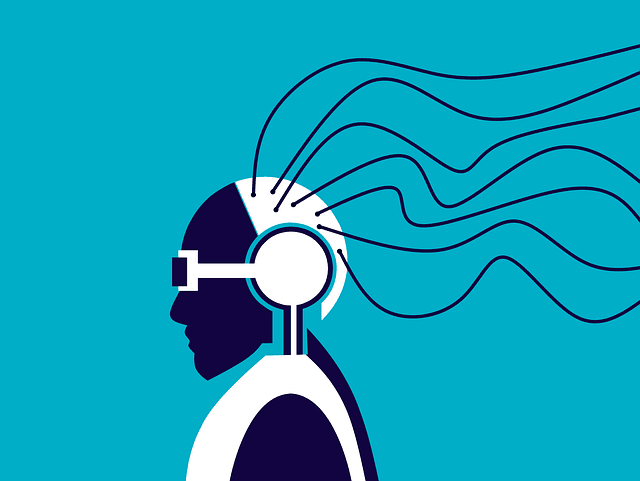
Airbnb is a prime example of a company using AI to refine its UX and personalize the user experience. By analyzing user preferences, search patterns, and booking behaviors, Airbnb’s AI-powered recommendation engine suggests properties and experiences tailored to individual users.
Airbnb: Leveraging AI for Personalization
Their AI tools don’t just stop at personalization—they also enhance usability testing. By simulating user interactions, Airbnb can identify design friction points before they become a problem for actual users. This approach ensures that every feature rollout aligns with user expectations, driving higher satisfaction and engagement.
Google: Automating Accessibility Testing
Google uses AI extensively to ensure accessibility across its products. With tools like Lighthouse, an automated testing tool powered by machine learning, Google identifies potential accessibility issues in web designs. This enables their teams to fix problems like poor contrast, missing alt text, or confusing navigation paths early in the development process.
By embedding AI into their workflow, Google ensures that their products are inclusive and functional for all users, setting a high standard for UX excellence.
Spotify: Predicting User Preferences
Spotify’s AI-driven UX research focuses on creating personalized music recommendations through its Discover Weekly and Daily Mix features. By analyzing billions of data points, including listening habits, skips, and playlist creations, Spotify’s AI anticipates what users want to hear next.
This data-driven approach not only enhances the user experience but also informs the design of Spotify’s interface. For example, they use AI insights to decide which playlists should appear on the home screen, making the app more intuitive and engaging.
Step 14: Practical Tips for Getting Started with AI in UX Research
If you’re new to AI, it’s important not to overwhelm your team or processes. Start by integrating AI tools into a single aspect of your UX research, such as data analysis or usability testing. Once you’re comfortable, gradually expand its role to include other areas like behavior prediction or sentiment analysis.
Start Small and Build Gradually
For example, you could begin with a tool like Hotjar for heatmaps and session recordings, then layer in NLP tools for analyzing feedback once you’ve mastered the basics.
Focus on Your Objectives
AI is a tool, not a solution in itself. Before implementing any AI-driven process, be clear about your goals. Are you looking to improve task completion rates? Reduce bounce rates? Identify navigation issues? Clear objectives help you choose the right tools and measure success effectively.
Collaborate and Share Insights
The real power of AI lies in its ability to uncover patterns and trends that may not be immediately obvious. Share AI-driven insights across teams to ensure they’re leveraged effectively. For instance, if AI identifies a navigation bottleneck, both the design and development teams should collaborate on a solution.
Final Thoughts: Smarter UX with AI
Leveraging AI in UX research isn’t about replacing human creativity—it’s about empowering teams with smarter tools and deeper insights. By automating repetitive tasks, analyzing data at scale, and predicting user needs, AI allows you to focus on what matters most: creating experiences that delight and engage your users.
As AI continues to evolve, its role in UX research will only grow. Stay curious, stay adaptable, and embrace the possibilities AI brings to your workflow. With the right tools and mindset, you can design better, faster, and more effectively than ever before. The future of UX research is here—make it work for you.
READ NEXT:
- Are Vanity Metrics Killing Your Marketing Efficiency? Here’s What to Track Instead
- Pinpointing Digital Marketing ROI: Why Your Metrics Aren’t Telling the Full Story
- Unlocking Real ROI in Digital Marketing: The Hidden Costs Draining Your Budget
- How Misaligned Marketing Funnels Are Blocking Your ROI Potential
- Best Digital Marketing Agency In Santa Ana, California
- Best Digital Marketing Agency In San Francisco, California