Understanding why customers leave your business is crucial for growth and sustainability. This is where predictive churn analysis comes into play. By leveraging AI-powered tools, businesses can anticipate which customers are likely to churn and take proactive measures to retain them. This guide will walk you through the process of using AI for predictive churn analysis, helping you reduce customer churn and increase loyalty.
Understanding Predictive Churn Analysis
What is Predictive Churn Analysis?
Predictive churn analysis is more than just identifying which customers are likely to leave; it’s about understanding the underlying reasons for their departure and leveraging these insights to create effective retention strategies.
For startup founders, this means taking a proactive approach to customer retention by using data and AI to anticipate and address issues before they lead to churn. This strategic advantage can significantly impact your startup’s growth and sustainability.
Why Use AI for Churn Analysis?
AI enhances the accuracy and depth of churn analysis by processing vast amounts of data quickly and identifying patterns that might be missed by human analysis. For startups, AI can transform how you approach customer retention, providing a scalable and efficient way to handle data-driven insights.
Traditional churn analysis methods often rely on historical data and basic statistical methods, which can be time-consuming and less accurate. AI-powered tools, however, can analyze diverse data sources in real-time, including customer interactions, transaction history, social media behavior, and even external factors like market trends. This comprehensive approach ensures that you have a holistic view of your customers and their behaviors.
For startup founders, this means being able to swiftly adapt to changes in customer behavior and market conditions. By leveraging AI, you can develop dynamic retention strategies that evolve with your customers’ needs and preferences, keeping your startup agile and responsive.
Key Benefits of Predictive Churn Analysis with AI
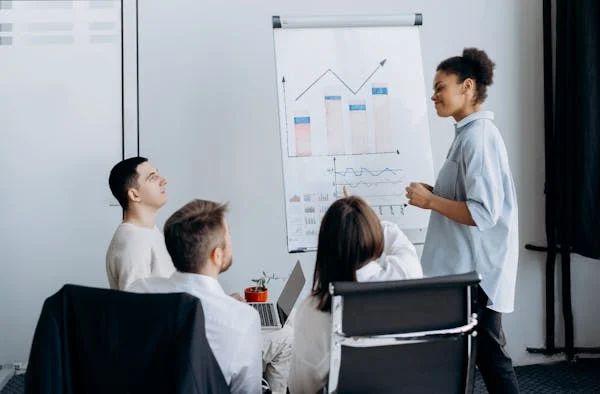
The benefits of using AI for predictive churn analysis are multifaceted, providing both immediate and long-term advantages for your startup.
One of the primary benefits is the ability to create highly personalized retention strategies. AI allows you to segment your customer base into distinct groups based on their behavior and preferences. This segmentation enables you to tailor your retention efforts to each group, ensuring that your strategies resonate with their specific needs.
Another significant benefit is improved resource allocation. By identifying which customers are at the highest risk of churning, you can focus your retention efforts and resources on these individuals. This targeted approach maximizes the efficiency of your retention campaigns and ensures that you are investing in the areas that will yield the highest returns.
For startups, the ability to predict churn also means better financial planning and stability. Predictive churn analysis provides insights into potential revenue losses, allowing you to develop contingency plans and mitigate the impact on your bottom line. This foresight is invaluable for maintaining investor confidence and securing future funding.
Additionally, AI-powered churn analysis can enhance your overall customer experience. By understanding the factors that contribute to churn, you can proactively address common pain points and improve your products and services. This continuous improvement loop not only reduces churn but also increases customer satisfaction and loyalty.
Strategic Implementation for Startup Founders
Implementing predictive churn analysis requires a strategic approach, especially for startups that may have limited resources and expertise.
Begin by establishing clear objectives for your churn analysis efforts. Understand what you want to achieve—whether it’s reducing churn rates, increasing customer lifetime value, or improving overall customer satisfaction. Clear objectives will guide your data collection and analysis processes, ensuring that your efforts are aligned with your business goals.
Next, invest in the right AI tools and technologies. Choose platforms that are scalable and can integrate seamlessly with your existing systems. Consider cloud-based solutions that offer flexibility and reduce the need for extensive infrastructure. Look for tools that provide user-friendly interfaces and robust analytical capabilities, enabling your team to easily interpret and act on the insights generated.
Data quality is paramount for effective churn analysis. Develop a data governance strategy that includes regular data cleaning and validation processes. Ensure that your data is comprehensive and covers all relevant aspects of customer behavior. This includes transactional data, interaction data, and any other data points that can provide insights into customer satisfaction and engagement.
Training and ongoing support are crucial for the successful implementation of AI-powered churn analysis. Provide your team with the necessary training to understand and utilize the AI tools effectively. Encourage a culture of continuous learning and adaptation, where feedback and insights are regularly used to refine your models and strategies.
Lastly, measure the impact of your predictive churn analysis efforts. Develop key performance indicators (KPIs) to track the effectiveness of your retention strategies. Regularly review these metrics and adjust your approaches based on the insights gained. This iterative process ensures that your churn analysis efforts remain relevant and effective, driving continuous improvement and growth.
Preparing for Predictive Churn Analysis
Collecting the Right Data
The foundation of any predictive churn analysis is data. You need to collect and organize relevant data to build an effective model. Start with basic customer information, such as demographics and contact details.
Then, gather data on customer interactions, including purchase history, website visits, and customer service interactions. Social media activity and feedback can also provide valuable insights.
Ensure that your data is clean and accurate. Incomplete or incorrect data can lead to inaccurate predictions. Regularly update your data to reflect the most recent customer behavior.
Choosing the Right AI Tools
Selecting the right AI tools is crucial for effective predictive churn analysis. There are various AI platforms available, each offering different features and capabilities. Look for tools that can handle large datasets and provide robust analytical capabilities. Popular options include Google AI, IBM Watson, and Microsoft Azure AI.
Consider the ease of integration with your existing systems. The AI tool you choose should seamlessly integrate with your CRM, marketing platforms, and other business systems. This will ensure that you can easily access and analyze the data you need.
Data Preparation and Cleaning
Before you can start building your predictive model, you need to prepare and clean your data. This involves removing duplicates, handling missing values, and standardizing data formats. Data cleaning is a critical step, as it ensures that your model is built on accurate and reliable data.
Develop a data governance framework to maintain data quality over time. This includes establishing procedures for data collection, storage, and management. Regularly review and update your data to ensure it remains relevant and accurate.
Building Your Predictive Model
Once your data is prepared, you can start building your predictive model. This involves selecting the appropriate machine learning algorithms and training your model with historical data. Use a portion of your data for training and another portion for testing to evaluate the model’s performance.
Continuously monitor and refine your model to improve its accuracy. As you collect more data, update your model to ensure it reflects the most recent customer behavior.
Building and Training Your Predictive Model
Selecting the Right Algorithms
The choice of algorithm is critical in building an effective predictive churn model. Different algorithms are suited to different types of data and business requirements. Some commonly used algorithms for churn prediction include logistic regression, decision trees, random forests, and neural networks.
Logistic regression is straightforward and interpretable, making it a good starting point. Decision trees and random forests can handle complex interactions in the data, while neural networks can capture intricate patterns but require more data and computational power.
Consider starting with a simple model and gradually experimenting with more complex algorithms as you gain confidence and understanding of your data. Use cross-validation techniques to evaluate the performance of different algorithms and choose the one that provides the best predictive accuracy.
Training Your Model
Training your predictive model involves feeding it with historical data so it can learn the patterns associated with customer churn. Divide your dataset into a training set and a testing set. The training set is used to build the model, while the testing set is used to evaluate its performance.
During the training process, the algorithm identifies relationships between the input features (such as customer demographics, purchase history, and engagement metrics) and the target variable (churn or no churn). The model adjusts its parameters to minimize the difference between its predictions and the actual outcomes in the training data.
After training the model, validate its performance using the testing set. Evaluate metrics such as accuracy, precision, recall, and the F1 score to assess how well the model predicts churn. Continuously refine and improve your model based on these evaluations.
Feature Engineering and Selection
Feature engineering involves creating new variables (features) that can help improve the predictive power of your model. For instance, you can create features that capture customer behavior trends over time, such as the average purchase frequency or the number of support tickets submitted in the last six months. Feature selection, on the other hand, involves identifying the most important variables that contribute to predicting churn.
Use techniques like correlation analysis and feature importance scores to identify which features have the most significant impact on churn. Removing irrelevant or redundant features can simplify the model and improve its performance. Experiment with different combinations of features to find the optimal set for your predictive model.
Implementing Your Predictive Model
Once your model is trained and validated, it’s time to implement it into your business processes. Integrate the model with your CRM system, marketing platforms, and other relevant tools. This integration will allow you to automatically flag at-risk customers and take proactive measures to retain them.
Develop a real-time dashboard to monitor churn predictions and key metrics. This will enable your team to track the model’s performance and make data-driven decisions. Ensure that your team is trained on how to interpret and act on the insights generated by the model.
Acting on Predictive Churn Insights
Personalized Retention Strategies
The primary goal of predictive churn analysis is to retain customers before they leave. Use the insights generated by your model to develop personalized retention strategies. For example, if the model predicts that a customer is likely to churn due to a lack of engagement, you can reach out with targeted offers, personalized content, or special discounts to re-engage them.
Segment your at-risk customers based on the factors contributing to their potential churn. Create customized retention campaigns for each segment, addressing their specific needs and concerns. Personalization is key to making your retention efforts more effective.
Enhancing Customer Experience
Predictive churn analysis can also help you identify areas where the customer experience can be improved. Analyze the common pain points and issues that lead to churn and take steps to address them. This could involve improving product features, enhancing customer support, or streamlining the user journey.
By proactively addressing these issues, you can enhance the overall customer experience and reduce the likelihood of churn. Regularly collect feedback from your customers to understand their needs and expectations better. Use this feedback to make continuous improvements to your products and services.
Proactive Communication
Effective communication is essential for retaining customers. Use the insights from your predictive model to reach out to at-risk customers with timely and relevant messages. This could include check-in emails, satisfaction surveys, or personalized offers based on their behavior and preferences.
Ensure that your communication is consistent and value-driven. Show your customers that you care about their experience and are committed to meeting their needs. Building strong relationships through proactive communication can significantly reduce churn rates.
Monitoring and Iteration
Predictive churn analysis is not a one-time task. Continuously monitor the performance of your model and the effectiveness of your retention strategies. Collect data on the outcomes of your interventions and use this information to refine your approach.
Regularly update your predictive model with new data to ensure it remains accurate and relevant. As your business and customer base evolve, so will the factors contributing to churn. Stay agile and be ready to adapt your strategies based on new insights and trends.
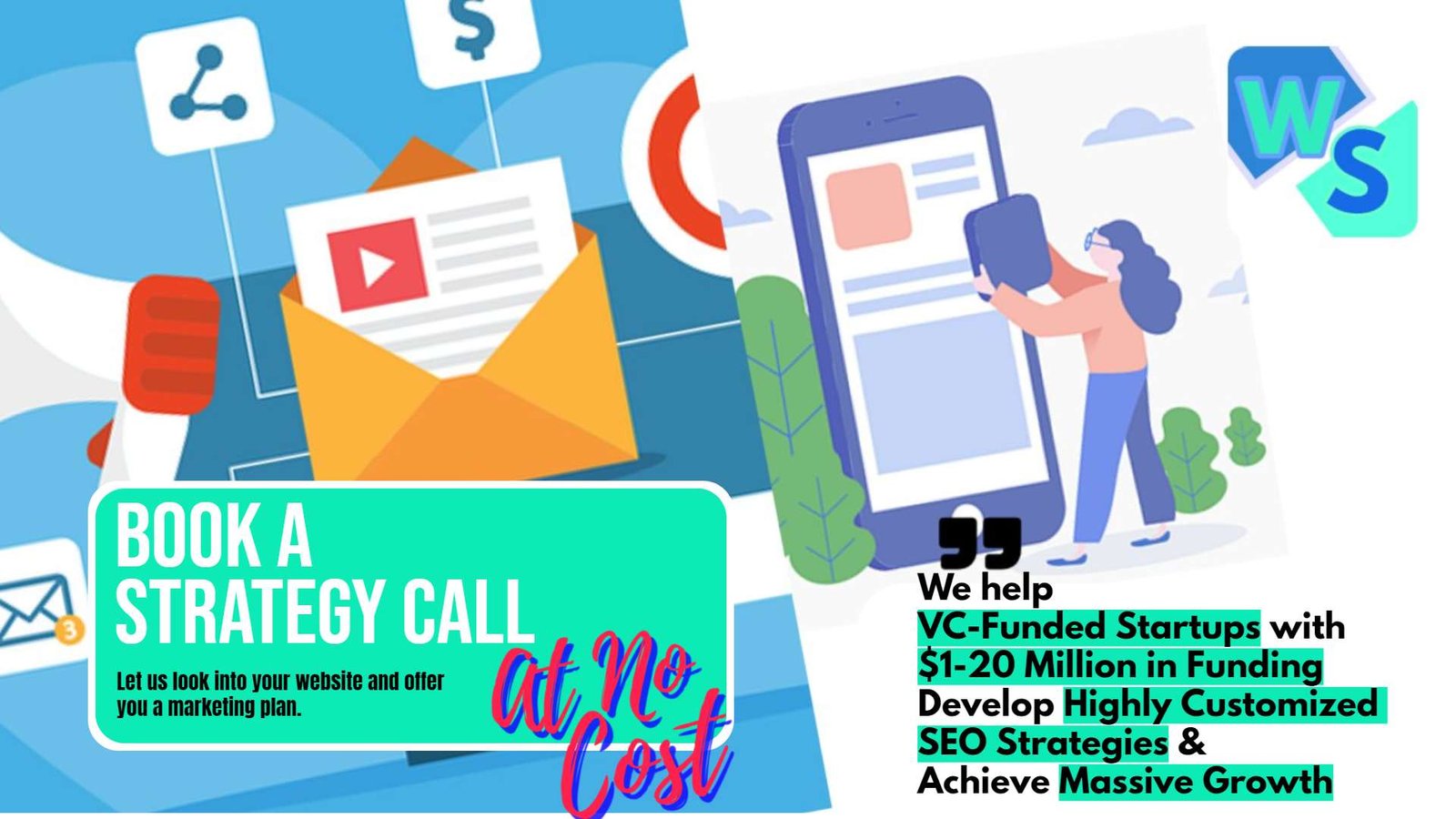
Related: Check out our free tools:
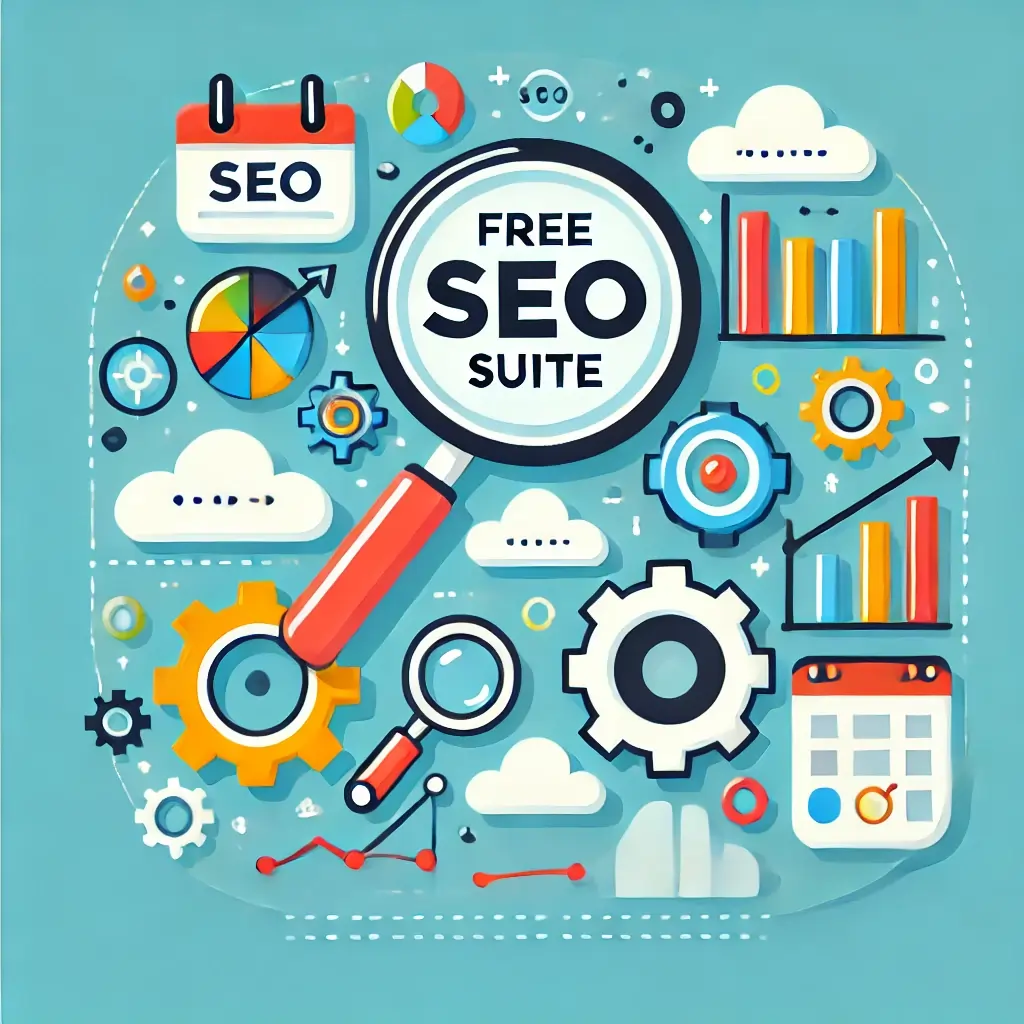
Case Studies: Successful Predictive Churn Analysis
Netflix: Keeping Viewers Engaged
Netflix is renowned for its use of AI and predictive analytics to retain subscribers. By analyzing viewing habits, search queries, and interaction data, Netflix can predict when a user is likely to cancel their subscription. The company uses these insights to recommend content that aligns with the user’s preferences, ensuring they remain engaged with the platform.
For instance, if a user frequently watches a particular genre, Netflix’s algorithms will prioritize showing new releases and recommendations in that genre. This personalized approach not only enhances user satisfaction but also reduces the likelihood of churn.
Spotify: Tailoring the Listening Experience
Spotify leverages AI to analyze user behavior, such as listening history, playlist creation, and interaction with the app. By identifying patterns that indicate a user might stop using the service, Spotify can take proactive steps to retain them.
One of Spotify’s key strategies is its personalized playlists, like Discover Weekly and Release Radar, which introduce users to new music tailored to their tastes. Additionally, Spotify sends notifications about new releases from favorite artists or personalized concert recommendations. These personalized touchpoints keep users engaged and reduce churn.
Salesforce: Enhancing Customer Relationships
Salesforce uses predictive churn analysis to help businesses understand customer health and retention risks. By integrating AI into its CRM platform, Salesforce provides businesses with insights into customer behavior and engagement levels.
The platform’s AI, known as Einstein, analyzes various data points such as customer interactions, product usage, and support ticket history. It then predicts which customers are at risk of churning and suggests actions to improve their satisfaction. Businesses can use these insights to tailor their customer engagement strategies, improving retention and building stronger customer relationships.
Common Challenges in Predictive Churn Analysis
Data Quality and Integration
One of the primary challenges in predictive churn analysis is ensuring data quality and integration. Inaccurate, incomplete, or outdated data can lead to incorrect predictions and ineffective retention strategies. Businesses need to invest in robust data collection and management processes to maintain data integrity.
Integrating data from multiple sources can also be challenging, especially for businesses with disparate systems. Ensuring seamless data integration is crucial for building a comprehensive view of customer behavior.
Managing Model Complexity
As predictive models become more complex, managing and interpreting them can be challenging. Advanced models like neural networks can provide highly accurate predictions, but they often act as black boxes, making it difficult to understand how they arrive at their conclusions.
To address this, businesses should balance model complexity with interpretability. Simple models like decision trees and logistic regression are easier to interpret, while more complex models require additional tools and techniques to explain their predictions.
Keeping Up with Changing Customer Behavior
Customer behavior is dynamic and can change rapidly due to various factors such as market trends, economic conditions, and competitive actions. Predictive churn models need to be continuously updated with new data to remain accurate and relevant.
Businesses should establish processes for regularly updating and validating their models. This ensures that the models adapt to changing customer behavior and continue to provide reliable predictions.
The Future of Predictive Churn Analysis
Advances in AI and Machine Learning
The future of predictive churn analysis is set to be transformed by ongoing advances in AI and machine learning. These technologies will continue to evolve, offering more sophisticated tools and techniques for understanding and predicting customer behavior. For startup founders, staying abreast of these developments is critical.
Advanced AI models such as deep learning and reinforcement learning will allow for even more precise and nuanced churn predictions. These models can analyze vast datasets with multiple variables, capturing complex relationships and subtle patterns that simpler models might miss.
Startups should consider investing in AI research and development to leverage these cutting-edge technologies. Collaborate with academic institutions, AI research firms, or hire in-house data scientists to explore how advanced AI can be integrated into your churn analysis strategies. By being early adopters, startups can gain a significant competitive advantage.
Integration with Real-Time Data
The ability to integrate real-time data into predictive churn models will revolutionize how businesses respond to potential churn. Real-time data allows for immediate detection of behavioral changes and timely interventions.
For example, if a customer suddenly reduces their usage of a service or starts engaging with competitors more, real-time analytics can trigger immediate retention strategies, such as targeted offers or personalized messages.
Startup founders should prioritize building infrastructure that supports real-time data collection and analysis. This involves setting up systems that can continuously capture and process data from various touchpoints, including online interactions, mobile app usage, and social media activity. Ensuring that your AI tools can handle and analyze real-time data will make your churn predictions more accurate and actionable.
Personalized and Contextual Interventions
As AI becomes more sophisticated, the future of churn analysis will focus on delivering personalized and contextual interventions. This means not only predicting who is likely to churn but also understanding why and how to address the underlying issues. Personalized interventions can include customized product recommendations, tailored customer service interactions, or exclusive loyalty rewards.
For startup founders, developing a deep understanding of individual customer journeys is crucial. Utilize AI to create detailed customer profiles that encompass all aspects of their interactions with your business.
Use these profiles to design personalized retention strategies that address specific pain points and preferences. By offering highly relevant solutions, startups can significantly enhance customer satisfaction and reduce churn rates.
Ethical AI and Data Privacy
As predictive churn analysis becomes more prevalent, ethical considerations and data privacy will become increasingly important. Consumers are becoming more aware of how their data is used and expect businesses to handle it responsibly. Ensuring transparency and building trust with your customers will be essential.
Startup founders must prioritize ethical AI practices and robust data privacy measures. This includes obtaining explicit consent for data collection, being transparent about how data is used, and implementing strong data security protocols.
Regularly review and audit your AI models to ensure they are fair, unbiased, and compliant with relevant regulations. By committing to ethical AI, startups can build trust with their customers and differentiate themselves in the market.
Cross-Functional Collaboration
The future of predictive churn analysis will see greater cross-functional collaboration within organizations. Effective churn reduction strategies require input and cooperation from various departments, including marketing, sales, customer service, and product development. AI-driven insights should be shared across teams to ensure a cohesive approach to customer retention.
Startup founders should foster a culture of collaboration and data sharing within their organizations. Establish cross-functional teams that regularly review churn data and develop integrated retention strategies. Encourage open communication and continuous feedback to refine and improve your approaches. By breaking down silos and promoting teamwork, startups can create a more unified and effective response to customer churn.
Leveraging External Data Sources
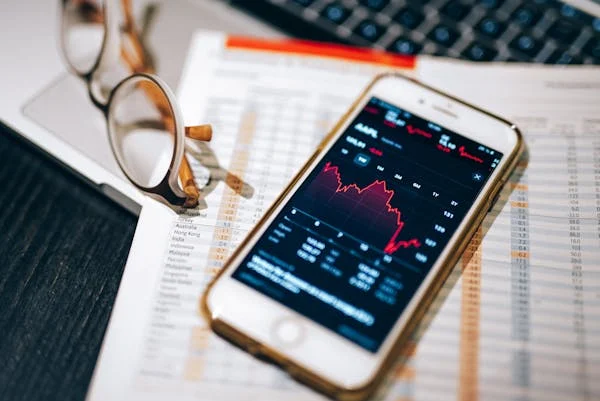
Incorporating external data sources will enhance the accuracy and depth of predictive churn analysis. External data, such as market trends, economic indicators, and competitive activity, can provide valuable context and improve your understanding of customer behavior. For example, economic downturns or shifts in industry trends might impact customer loyalty and spending habits.
Startup founders should explore partnerships with data providers and consider subscribing to relevant data feeds. Integrate this external data with your internal datasets to create more comprehensive and accurate churn models. By understanding the broader context in which your customers operate, you can develop more effective retention strategies.
Continuous Learning and Adaptation
The future of predictive churn analysis will require continuous learning and adaptation. As customer behavior evolves and new technologies emerge, your churn models and retention strategies must adapt accordingly. Staying ahead of the curve will involve regular updates to your models, ongoing training for your team, and a commitment to innovation.
Startup founders should create a culture of continuous improvement within their organizations. Encourage your team to stay updated with the latest trends and advancements in AI and predictive analytics.
Invest in ongoing training and professional development to ensure your team has the skills and knowledge to leverage new technologies. Regularly review and refine your predictive models and retention strategies to keep them relevant and effective.
Conclusion
Predictive churn analysis with AI-powered tools is a game-changer for businesses, particularly for startups looking to establish a strong foothold in their markets. By leveraging AI, startups can anticipate customer behavior, identify at-risk customers, and implement effective retention strategies before churn happens. This proactive approach can significantly enhance customer satisfaction, increase loyalty, and drive long-term growth.
Read Next:
- The Link Between Social Media and Eating Disorders: Eye-Opening Statistics
- Social Media and Mental Health: Crucial Statistics for 2024
- Inbound Marketing Success: Key Statistics and Best Practices
- How Photographers Can Market Their Services
- Innovative Ways to Use QR Codes in Marketing
Comments are closed.