Imagine if you could predict your business’s future, optimize operations, and drive unprecedented growth—all with the help of artificial intelligence (AI). In today’s fast-paced world, AI is no longer just a buzzword; it’s a powerful tool that can transform your business analytics and growth strategies. This article dives deep into how AI can revolutionize your business, offering practical, actionable advice to help you harness this technology for success.
Understanding AI in Business Analytics
What is AI in Business Analytics?
AI in business analytics refers to the use of advanced algorithms and machine learning techniques to process and analyze data. This technology goes beyond traditional data analysis by learning from data patterns and making predictions, thereby offering insights that can drive strategic business decisions. For startup founders, understanding how AI can be integrated into business analytics is crucial for staying competitive and fostering growth.
How AI Works in Business Analytics
AI works by processing large datasets to identify patterns, correlations, and trends that might not be immediately apparent. Here’s a deeper look into how AI functions within business analytics:
Data Collection: The first step is gathering data from various sources. This could include customer interactions, sales transactions, market trends, and even social media activity. For startup founders, it’s important to identify which data sources are most relevant to your business objectives.
Data Preprocessing: Raw data often needs cleaning and structuring before it can be analyzed. This involves handling missing values, eliminating duplicates, and standardizing formats. High-quality data is the foundation of reliable AI insights, so investing in data preprocessing is crucial.
Algorithm Selection: Different AI algorithms serve different purposes. For instance, regression models might be used for sales forecasting, while clustering algorithms could segment customers based on behavior. Selecting the right algorithm depends on the specific business problem you are addressing.
Model Training: During this phase, the AI model is trained using historical data. The model learns from past data to recognize patterns and make predictions about future outcomes. It’s essential to split your data into training and testing sets to validate the model’s accuracy.
Model Deployment: Once trained, the AI model is deployed into your business systems. This integration allows for real-time data analysis and insights, enabling you to make informed decisions quickly. Continuous monitoring and refinement of the model ensure it adapts to new data and remains accurate.
Strategic Implementation for Startup Founders
For startup founders, the implementation of AI in business analytics requires a strategic approach. Here are some actionable steps to consider:
Identify Key Metrics: Determine the key performance indicators (KPIs) that are most critical to your business. Whether it’s customer acquisition cost, churn rate, or revenue growth, understanding these metrics will help you focus your AI efforts where they matter most.
Start Small: Implement AI in a specific area of your business where it can have the most immediate impact. This could be in marketing, sales, or customer service. Starting small allows you to test the effectiveness of AI without a significant upfront investment.
Build a Cross-Functional Team: Successful AI implementation requires collaboration between different departments. Assemble a team that includes data scientists, IT professionals, and business strategists. This cross-functional approach ensures that the AI solutions are well-integrated and aligned with your business goals.
Invest in Training: AI is only as effective as the people who use it. Invest in training your team to understand AI concepts and tools. This includes both technical training for your IT staff and strategic training for your business leaders.
Focus on Data Quality: The accuracy of AI predictions hinges on the quality of the data. Develop a robust data governance framework to ensure data accuracy, consistency, and security. This might involve regular audits, data cleaning processes, and strict data management policies.
Successful AI implementation in startups requires carefully structured cross-functional teams that blend technical expertise with business acumen. The foundation typically consists of data scientists working alongside domain experts, software engineers, and business analysts, with each role bringing essential perspectives to the project.
This core team should be supported by project managers who understand both technical constraints and business objectives, ensuring that AI initiatives remain aligned with organizational goals while maintaining practical feasibility.
Effective collaboration between technical and business teams requires establishing common ground through shared vocabulary and regular knowledge-sharing sessions. Organizations finding success in this area often implement “translators” – team members who can bridge the gap between technical capabilities and business requirements.
These individuals play a crucial role in ensuring that data scientists understand business context while helping business stakeholders grasp technical limitations and opportunities.
Long-term success depends on creating an environment where diverse perspectives are valued and integrated into decision-making processes. Regular cross-functional meetings, shared project management tools, and collaborative goal-setting sessions help maintain alignment and ensure that AI solutions address real business needs.
The most effective teams maintain flexible structures that allow for rapid iteration and adjustment based on project outcomes and changing business requirements.
Today, Startups are keen on implementing AI-powered tools and systems for effective management and optimization of business projects. For the successful implementation of AI in startups, I think data scientists, analysts, data engineers, IT, DevOps specialists, business strategists, and product managers play pivotal roles. Let me elaborate.
Data scientists’ job in implementing AI is to build ML algorithms in alignment with the startup’s goals. They tailor models to align customer segmentation and demand forecasting goals. While data scientists work to develop algorithms, analysts collaborate with them to analyze and interpret these models to generate meaningful insights to support business objectives. Coming to data engineers, who handle data extraction and transformation.
They also oversee ETL processes to create clean and usable data sets. Data engineers also work collaboratively with data scientists to ensure quality data is used and data processing is efficient. Next on the list are IT and DevOps specialists. They ensure infrastructure used for AI models is secure, efficient, and scalable.
They deploy production models, manage cloud services, and optimize server resources. Along with these, IT and DevOps specialists also ensure security protocols are in place. Lastly, business strategists and product managers, are responsible for connecting technical teams. They ensure business objectives are in alignment with AI technologies.
While business strategists define objectives and clarify organizational needs, product managers are responsible for translating these objectives into actionable requirements for the team. Their collaborative efforts are typically aligned with the company’s mission.
In my opinion, the best way to keep all teams aligned on the common business goals is by following these steps- establishing clear communication between teams, organizing cross-functional workshops to understand each other’s perspectives and contributions, defining success metrics together, and ensuring continuous feedback loops.
What Roles Are Essential for Successful AI Implementation in Startups?
An AI-driven business analytics team needs a balanced mix of specialized roles. First, data scientists bring essential modeling and statistical analysis skills, working on the core algorithms that power insights. Then, data engineers handle the infrastructure, ensuring seamless data integration and robust pipelines, which is critical for scaling analytics effectively. IT specialists are also vital, as they manage the tools and maintain security, ensuring compliance and system integrity.
Equally important are business strategists who connect the dots between technical output and business goals—helping ensure AI insights are actionable and aligned with company strategy. Finally, product managers serve as liaisons, translating technical requirements into tangible objectives for each team, bridging the often complex dynamics between data science and business functions.
Fostering Collaboration Across Functions
Creating a cohesive, communicative team requires more than just the right roles; it’s about building a culture of shared understanding and respect for each function. One method we use at Summer is cross-functional training sessions, where team members learn the basics of each other’s domains.
For instance, we might have a data scientist explain the basics of a machine learning model to the business team, or a strategist demonstrate how customer insights influence data priorities. These sessions build empathy and awareness, fostering smoother communication and reducing misunderstandings.
Strategies to Keep the Team Aligned on Business Goals
Regular check-ins and updates are essential to ensure alignment. We hold bi-weekly alignment meetings where each function presents their progress, hurdles, and immediate goals. By keeping updates clear and relevant to the company’s core objectives, we reinforce how each person’s work directly contributes to the larger picture.
Setting shared KPIs for project success is another effective tool; when data scientists, IT, and strategists are measured against common business metrics, there’s a stronger incentive to keep work cohesive. Additionally, empowering the team with autonomy, but with a firm grasp of the company’s vision, fosters innovation within the bounds of business needs.
If you’re just now developing cross-functional teams to maximize the value of your AI tools, you’re missing out on a valuable organizational approach. Cross-functional teams are the core of our development and service model.
They allow us to take a project-by-project approach to using the tools we have and developing new tools like AI. Building on existing personal relationships is an essential step for success here.
Leveraging AI for Competitive Advantage
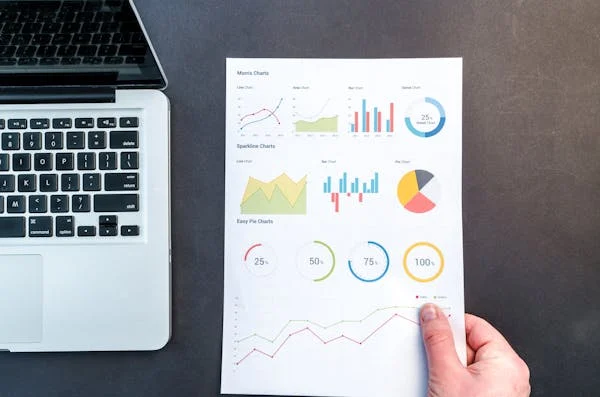
To leverage AI for a competitive advantage, startup founders should focus on the following strategies:
Predictive Analytics: Use AI to predict future trends and behaviors. This could involve forecasting sales, anticipating customer needs, or predicting market shifts. By staying ahead of these trends, you can make proactive decisions that keep you competitive.
Personalized Marketing: AI can analyze customer data to create highly personalized marketing campaigns. This increases engagement and conversion rates by delivering the right message to the right customer at the right time.
Operational Efficiency: AI can optimize various operational aspects of your business, from inventory management to supply chain logistics. By identifying inefficiencies and automating routine tasks, AI can significantly reduce costs and improve productivity.
Customer Insights: AI can provide deep insights into customer behavior and preferences. Use these insights to enhance the customer experience, develop new products, and improve customer retention strategies.
Real-Time Decision Making: AI enables real-time data analysis, allowing you to make informed decisions quickly. This agility is crucial in today’s fast-paced business environment, where being able to respond swiftly to market changes can be a significant advantage.
Overcoming Challenges in AI Implementation
Implementing AI in business analytics is not without its challenges. Here are some common hurdles and strategies to overcome them:
Data Privacy: Handling sensitive data requires strict adherence to privacy regulations. Ensure that your AI systems comply with laws such as GDPR and CCPA. Implement robust data protection measures to secure customer information.
Integration with Legacy Systems: Many businesses struggle with integrating AI into their existing systems. Consider adopting cloud-based AI solutions that offer better flexibility and easier integration with your current infrastructure.
Cost: AI implementation can be costly, especially for startups with limited budgets. Focus on high-impact areas where AI can deliver the most value. Look for scalable solutions that allow you to expand your AI capabilities as your business grows.
Skill Gaps: AI requires specialized skills that may not be readily available within your team. Consider partnerships with AI vendors or investing in training programs to build the necessary expertise.
Resistance to Change: Adopting new technologies often meets resistance from employees. Foster a culture of innovation by communicating the benefits of AI and involving employees in the implementation process. Provide adequate support and training to ease the transition.
1. Addressing Data Quality Issues
Data quality is the foundation of any successful AI implementation. For startups, establishing data validation routines early is crucial. Basic steps, like removing duplicates, ensuring consistent formats, and flagging outliers, can often be achieved through open-source tools, such as Python’s Pandas library, without incurring heavy costs. This simple, early attention to data integrity helps build a reliable foundation, making insights accurate and scalable as you grow.
2. Integrating AI with Legacy or Existing Systems
Integrating AI with legacy systems is particularly challenging for startups with lean tech teams, as older systems weren’t designed for modern analytics. A modular approach—building AI components as microservices that communicate with legacy systems via APIs—enables incremental implementation without overhauling entire systems. Using serverless, cloud-based options like AWS Lambda provides lightweight, flexible integration that can scale with your business while preserving existing operations.
3. Bridging the Skill Gap in AI and Data Science*
Startups often lack in-house talent with both business and AI expertise. Building a culture of cross-functional collaboration and upskilling can help mitigate this. Online courses and knowledge-sharing sessions support AI literacy across the team. Additionally, partnering with specialized AI consultants for initial projects provides immediate expertise without the long-term costs of hiring full-time staff. This approach allows for skill-building while ensuring the project meets technical standards from the outset.
Practical Solutions for Limited Resources
For startups, a focus on small, high-impact AI projects rather than a complete transformation is often the best path forward. Begin with pilot projects that deliver measurable value quickly, like automating a single report or implementing a basic recommendation engine. As these projects demonstrate ROI, it becomes easier to justify additional resources for AI expansion.
Data Solution:
Instead of collecting new data, we started with our existing waste collection sheets – basic paper records our drivers used. By simply photographing these sheets daily, we built a valuable dataset without expensive systems. Last month, this simple approach helped us predict collection needs with 85% accuracy.
Integration Discovery:
Rather than replacing our old systems, we found success layering AI gradually. We kept our basic scheduling software but added a simple prediction layer on top. This saved us £20,000 in system replacement costs while improving route efficiency by 40%. When a major route planning failed last week, we could still fall back on our basic system.
Skills Gap Reality:
We couldn’t afford data scientists so we trained our existing staff. Our office manager now handles basic AI tools after completing online courses. She reduced our fuel costs by 30% using simple pattern recognition tools, proving you don’t need advanced degrees for effective AI use.
Cost-Effective Approach:
Instead of comprehensive AI systems, we use free tools like Google Colab for specific tasks. This targeted approach improved our waste sorting accuracy by 45% while keeping our monthly tech budget under £500.
The implementation of AI in startup environments presents unique challenges that require strategic approaches and careful resource allocation. Data quality emerges as a primary concern, with many startups struggling to accumulate sufficient high-quality data for effective AI model training.
This challenge is compounded by limited access to data cleaning and preparation resources, requiring innovative approaches such as synthetic data generation or strategic partnerships for data sharing.
Integration with existing systems poses another significant hurdle, particularly for startups operating with a mix of modern and legacy technologies. Successful implementations often begin with careful assessment of current infrastructure, followed by phased integration approaches that prioritize critical functionalities while minimizing disruption to ongoing operations.
This measured approach allows startups to maintain business continuity while gradually building their AI capabilities.
The skills gap in AI implementation can be addressed through a combination of strategic hiring, upskilling existing staff, and leveraging external partnerships. Successful startups often adopt hybrid approaches, combining internal talent development with strategic use of AI platforms and tools that reduce the need for specialized expertise.
This approach allows organizations to build AI capabilities incrementally while managing resource constraints and maintaining focus on core business objectives.
When implementing AI in business analytics, startups often grapple with challenges such as data quality, integration with existing systems, and skill gaps. To address data quality, it’s essential to establish a clear data governance framework that ensures data accuracy and consistency.
For integrating AI with legacy systems, I focus on creating a scalable architecture that allows for seamless integration, which often involves modular solutions or microservices.
Skill gaps can be managed by concentrating on building a diverse team with various competencies or by leveraging online platforms for training.
Startups with limited resources might find success by adopting open-source AI tools and collaborating with third-party vendors who offer AI-as-a-service. Emphasizing clear objectives and maintaining flexibility in AI deployment can help navigate these obstacles efficiently.
How Startups Can Overcome AI Implementation Challenges in Business Analytics
For startups, implementing AI in business analytics can be a game-changer as it brings out important insights to help the growth and efficiency of your company. But AI implementation comes with its own set of challenges that can be especially difficult for cash-starved startups, namely poor data quality, difficulties blending in existing systems, and a skills shortage. Now here is how startups can overcome these challenges.
1. Addressing Data Quality Issues
Quality data is essential for AI success. Startups often deal with inconsistent or incomplete data, which can affect AI model accuracy. To overcome this, focus on collecting relevant, high-quality data from a few core sources rather than trying to incorporate all data immediately.
- Automated Data Cleaning Tools: Tools such as Trifacta or Talend automate the process of data cleansing: de-duplication, entry standardization, and so on. They offer great assistance without demanding an internal data engineering capacity.
- Establishing Data Validation: From day one, incorporate steps to verify outcomes. Standardizing data entry fields and maintaining consistency are undergoing a steady improvement over time as well. For example, By doing this, your AI models always have the promise of reliable inputs that serve as a good starting point for analytics.
2. Integrating AI with Legacy Systems
Integrating legacy systems with AI solutions can be a daunting task. This is a common problem for startups that partner with larger companies and have to innovate new AI tools on top of legacy infrastructure. The solution to this, is using flexible integration strategies:
- Middleware Solutions: Middleware tools like Zapier or Apache Nifi can connect modern AI applications with legacy systems. Middleware acts as a bridge, simplifying data transfer without the need for reconfiguring entire systems.
- Cloud-Based AI Solutions: Moving to cloud platforms like AWS, Google Cloud, or Microsoft Azure offers a flexible alternative for integrating AI. These platforms come with built-in connectors that make it easier to link with legacy data while allowing scalability as business needs evolve.
3. Bridging the AI Skills Gap
Attracting AI talent is a challenge for startups due to budget constraints and high demand for skilled data professionals. However, there are creative ways to bridge this skills gap:
- Leverage Automated AI Platforms: Platforms like Google AutoML and Amazon SageMaker allow startups to deploy AI models without deep technical expertise. These tools provide a simplified interface and are designed for organizations with limited in-house AI skills.
- Outsource AI Expertise: Working with consultants or freelance AI experts through platforms like Upwork can be a practical option. This allows startups to bring in expertise as needed without the commitment of full-time hires.
- Invest in Upskilling: Upskilling current team members through courses on platforms like Coursera and DataCamp builds in-house AI knowledge over time. This approach is often cost-effective and fosters a culture of continuous learning within the team.
4. Practical Solutions for Limited Resources
For startups on a budget, deploying AI may feel challenging. However, with strategic planning, it’s achievable even with limited resources:
- Use Pre-Trained Models: Starting with pre-trained AI models from sources like Hugging Face or TensorFlow Hub can save time and costs. These models can be adapted to fit specific needs, allowing startups to implement AI solutions quickly without building everything from scratch.
- Focus on High-Impact Use Cases: Identify where AI can make the most impact—whether it’s automating customer interactions, improving predictive analytics, or optimizing marketing. Prioritizing high-value initiatives allows startups to demonstrate quick wins, which can build support for expanded AI investment in the future.
- Leverage Open-Source Tools: Open-source AI frameworks like TensorFlow and Scikit-learn provide high-quality resources for free. These frameworks also have active communities, offering a wealth of support and documentation.
Inspiring Startups to Embrace AI
Implementing AI may seem daunting, but each challenge is an opportunity for growth. By starting with small projects and focusing on high-impact applications, startups can gradually build confidence in their AI capabilities. Each successful implementation adds momentum, helping teams develop an agile, forward-thinking culture essential for long-term growth.
Cognida.ai, an IT firm specializing in AI, Data & Analytics, and Digital Engineering. Cognida.ai has helped businesses harness AI-driven solutions to enhance marketing performance through precise customer segmentation and predictive analytics, all of which contribute to more effective ad targeting and deeper customer engagement.
One standout example of Cognida’s work in this area is the use of Zunō.Predict, a tool leveraging machine learning to provide highly targeted, real-time customer insights. Unlike traditional segmentation based purely on demographics, Zunō.Predict enables dynamic customer profiles by analyzing behavioral data such as purchase patterns and browsing history.
This not only allows Cognida’s clients to anticipate customer needs but also to tailor proactive campaigns that increase conversion rates and boost ROI.
Through dynamic segmentation and real-time updates, Cognida has helped clients keep pace with changing customer preferences and behaviors. By segmenting customers based on precise needs and intentions, this can lead to minimized marketing spend on less relevant audiences, and maximized engagement from those most likely to convert.
One of the big challenges we’ve faced so far is the need to verify AI’s effectiveness and accuracy in analytics tasks.
We did this first with a small AI task force whose job was to explore how we could use these tools, but now we’re finding that we need to do it all again in order to get the whole team on board with using AI-generated insights.
It can be hard to explain that just because ChatGPT will sometimes make mistakes, that doesn’t mean this will be the case with other uses of AI.
The Future of AI in Business Analytics
The future of AI in business analytics is promising, with ongoing advancements poised to deliver even greater value. Here are some trends to watch:
AI-Driven Business Models: Companies will increasingly adopt AI-driven business models that leverage predictive analytics and automation to gain a competitive edge.
Enhanced Human-AI Collaboration: AI will augment human decision-making rather than replace it. Businesses will use AI to enhance human capabilities, providing deeper insights and enabling more informed decisions.
Explainable AI: As AI becomes more integrated into business processes, there will be a greater focus on explainable AI—making AI decisions transparent and understandable to users.
Edge Computing: AI-powered analytics will increasingly be performed at the edge, closer to the data source. This reduces latency and allows for real-time decision-making.
Benefits of AI in Business Analytics
Enhanced Decision-Making
One of the most significant benefits of AI in business analytics is improved decision-making. By providing accurate and timely insights, AI helps businesses make informed decisions quickly. This can lead to better outcomes, from increased sales to optimized operations.
For example, a retail business can use AI to analyze sales data and identify trends, helping them make better decisions about inventory management. This not only reduces costs but also ensures that popular products are always in stock, improving customer satisfaction.
Increased Efficiency
AI can automate many of the repetitive tasks involved in data analysis, freeing up valuable time and resources. This increased efficiency allows businesses to focus on strategic activities that drive growth.
For instance, AI can streamline the process of data collection and cleaning, ensuring that businesses have access to high-quality data without the need for manual intervention. This not only saves time but also reduces the risk of human error, leading to more reliable insights.
Personalized Customer Experiences
Personalization is key to customer satisfaction, and AI can help businesses deliver highly personalized experiences at scale. By analyzing customer data, AI can identify individual preferences and tailor marketing messages accordingly.
For example, an e-commerce business can use AI to recommend products based on a customer’s browsing history and past purchases. This not only increases the likelihood of a sale but also enhances the overall shopping experience, leading to higher customer loyalty.
AI-driven personalization has transformed how businesses connect with their customers, enabling unprecedented levels of individualized engagement across multiple touchpoints. Small and emerging businesses have leveraged AI tools to analyze customer behavior patterns, predict preferences, and deliver tailored content that resonates with specific audience segments.
These implementations have led to significant improvements in key metrics, with some organizations reporting up to 30% increases in conversion rates and 25% improvements in customer retention through AI-powered personalization strategies.
Machine learning algorithms analyzing historical customer data have enabled businesses to create dynamic customer profiles that evolve with each interaction. These profiles power sophisticated recommendation engines and personalized content delivery systems that adapt in real-time to customer behavior.
Companies implementing these systems have seen particular success in email marketing campaigns, where AI-driven personalization has led to significant improvements in open rates and click-through rates compared to traditional segmentation approaches.
The most successful implementations combine multiple AI approaches, including natural language processing for sentiment analysis, predictive analytics for behavior forecasting, and machine learning for content optimization.
Small businesses have found particular success with integrated platforms that combine these capabilities with user-friendly interfaces, allowing them to implement sophisticated personalization strategies without requiring extensive technical expertise.
These solutions have proven especially effective in e-commerce settings, where AI-driven product recommendations and personalized promotional strategies have significantly improved customer engagement and sales metrics.
For us, AI has been transformative in personalizing the user experience, which is critical in the fast-paced, highly interactive world of video dating. We’ve leveraged AI to match users based on detailed profile preferences and learn from each user’s engagement patterns in real-time. AI algorithms analyze user behavior—like preferred conversation topics, video call duration, and peak active times—to tailor recommendations that align closely with each user’s unique interests.
One of the fundamental AI tools we rely on is a recommendation engine, which dynamically adjusts and suggests connections, activities, or even conversation starters based on each user’s ongoing interactions. This has significantly increased our engagement rates, as users feel like the app “gets” them, offering a highly customized experience rather than a one-size-fits-all model. It’s not just about connections but making each user’s time on the platform feel relevant and exciting.
We’ve also seen AI-driven customer insights help us better understand our user base on a macro level. Analyzing data from these personalized interactions has allowed us to refine our marketing campaigns, leading to higher retention rates.
For example, targeted retention emails now highlight unique matches or suggest “date ideas” based on a user’s past behavior, which has proven to be a win in keeping users engaged with the app. This AI-powered personalization enhances user satisfaction and supports a more effective, data-driven marketing strategy that scales with us as we grow.
Personalization with predictive AI
Recently, I implemented predictive analytics to anticipate customer behavior on one of my platforms. I analyzed user behavior and tailored the content in real time to match individual preferences. As a result, I saw a noticeable improvement in engagement — more clicks and longer time on site (especially with repeat visitors).
Targeting with AI-based segmentation
AI-powered tools let businesses create more granular customer segments. This helps target specific offers to those who’ve shown interest in similar products before. For example, on my site, I tested AI-based recommendations, which led to more targeted traffic and a better customer experience. The result was improved click-through rates across the board.
Boosting customer retention with AI
AI tools enabled us to identify at-risk customers by analyzing behavioral patterns. We then tailored email campaigns to re-engage these users, leading to improved retention rates and increased repeat purchases.
Our videos and other media content are ultimately our best sales and marketing tools unless a customer happens to live next door to someone who has used our services.
We use AI tools to track customer behavior across our advertising efforts, with a special focus on which images they’ve seen and interacted with.
This can not only help us zero in on which products they’re interested in, but it can also let us re-use those images in follow-ups to keep them interested and engaged.
Predictive Analytics
Predictive analytics is one of the most powerful applications of AI in business. By analyzing historical data, AI can predict future trends and outcomes, allowing businesses to be proactive rather than reactive.
For instance, a financial services company can use AI to predict market trends and identify investment opportunities. This can give them a competitive edge, allowing them to capitalize on emerging trends before their competitors.
Cost Savings
AI can also lead to significant cost savings by optimizing various aspects of business operations. From reducing waste in supply chains to improving the efficiency of marketing campaigns, AI can help businesses operate more cost-effectively.
For example, a manufacturing company can use AI to predict equipment failures and schedule maintenance proactively. This not only reduces downtime but also extends the lifespan of the equipment, leading to substantial cost savings.
Implementing AI in Your Business Analytics Strategy
Assess Your Data Needs
Before implementing AI, it’s crucial to assess your data needs. Determine what types of data are most relevant to your business goals and ensure that you have the necessary data infrastructure in place.
Start by conducting a data audit to identify the sources and quality of your existing data. Ensure that your data is clean, accurate, and well-organized. This may involve removing duplicates, handling missing values, and standardizing data formats.
Choose the Right AI Tools
Selecting the right AI tools is essential for successful implementation. There are numerous AI platforms and tools available, each offering different features and capabilities. Look for tools that can handle large datasets, support various data types, and provide robust analytical capabilities.
Consider cloud-based AI solutions such as Google Cloud AI, IBM Watson, and Microsoft Azure AI, which offer scalability and flexibility. These platforms provide the infrastructure needed to process and analyze vast amounts of data efficiently.
Data Integration and Management
Effective implementation of AI requires seamless data integration and management. This involves integrating data from various sources into a centralized repository, ensuring that it’s easily accessible for analysis.
Develop a data management strategy that includes data governance policies, security protocols, and data access controls. Ensure that your data integration process supports interoperability between different systems and adheres to industry standards.
Building and Training AI Models
Once your data is prepared and integrated, the next step is to build and train your AI models. This involves selecting the right machine learning algorithms and feeding them with historical data to learn patterns and make predictions. Here’s how to go about it:
Define Objectives: Clearly outline what you want to achieve with your AI models. Whether it’s predicting sales, optimizing marketing campaigns, or improving customer retention, having clear objectives will guide the model development process.
Select Algorithms: Choose the appropriate machine learning algorithms based on your objectives and data characteristics. Common algorithms include regression analysis for predicting numerical outcomes, classification algorithms for categorizing data, and clustering algorithms for segmenting data.
Training and Testing: Divide your dataset into training and testing subsets. The training set is used to build the model, while the testing set evaluates its performance. This helps ensure that your model generalizes well to new data.
Iterative Improvement: AI model development is an iterative process. Continuously refine your models by incorporating new data and feedback. This iterative approach helps improve the model’s accuracy and reliability over time.
Integrating AI into Business Processes
After building and training your AI models, the next step is to integrate them into your business processes. This involves embedding AI insights into your operational workflows, making them easily accessible to decision-makers.
User-Friendly Dashboards: Develop intuitive dashboards that present AI-generated insights in a clear and actionable manner. These dashboards should be accessible to various stakeholders, from executives to frontline employees, ensuring that everyone can benefit from AI insights.
Automation: Automate routine tasks using AI to increase efficiency. For instance, use AI to automate customer segmentation, targeted marketing campaigns, and sales forecasting. Automation not only saves time but also ensures consistent and accurate execution.
Training and Support: Provide training and support to your team to help them understand and utilize AI insights effectively. This includes regular workshops, training sessions, and resources to keep your team updated on the latest AI tools and techniques.
Monitoring and Continuous Improvement
The implementation of AI in business analytics is an ongoing process. Continuous monitoring and improvement are essential to ensure that your AI models remain effective and relevant.
Performance Metrics: Establish key performance indicators (KPIs) to track the success of your AI initiatives. Common KPIs include accuracy of predictions, reduction in operational costs, and improvements in customer satisfaction.
Regular Audits: Conduct regular audits of your AI models to ensure they remain accurate and unbiased. This includes reviewing the data, algorithms, and outputs to identify any potential issues or areas for improvement.
Feedback Loop: Create a feedback loop where insights from AI are continuously fed back into the system to refine and enhance the models. This iterative process helps your AI capabilities evolve with changing business needs and market conditions.
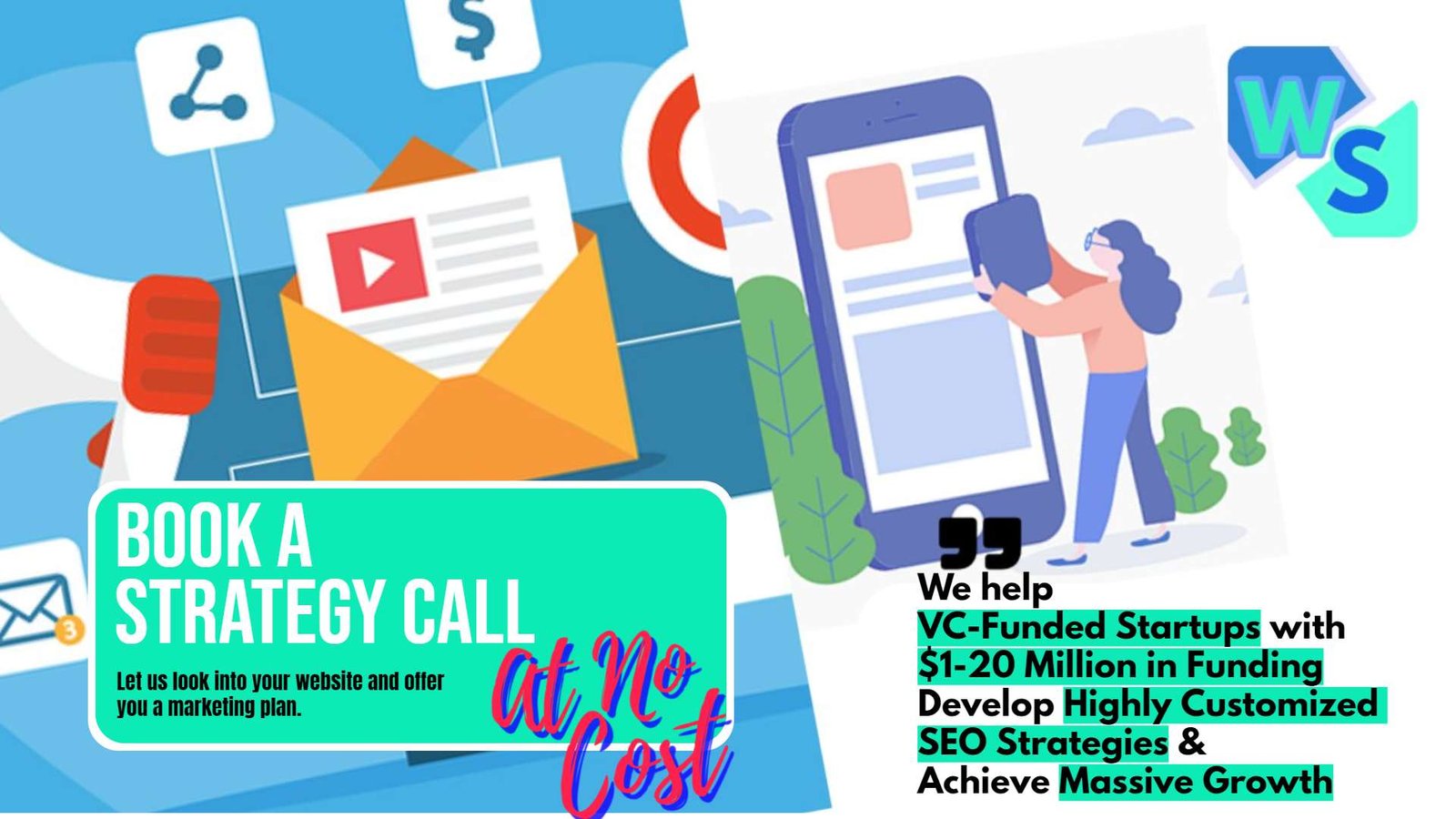
Related: Check out our free tools:
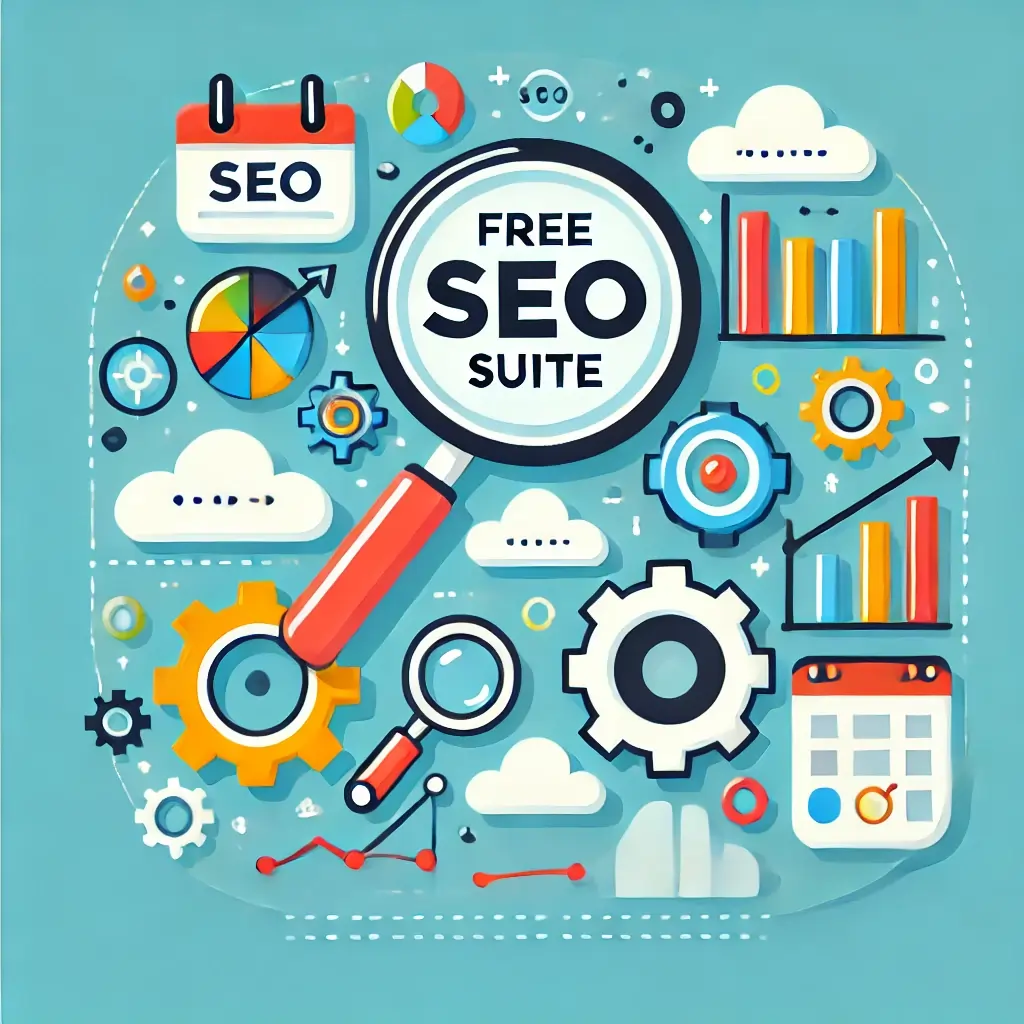
Case Studies of AI in Business Analytics
Retail: Walmart’s Inventory Management
Walmart, one of the largest retail chains globally, leverages AI to optimize its inventory management. By analyzing sales data, customer preferences, and external factors like weather patterns, Walmart’s AI systems predict demand for various products. This enables the company to maintain optimal inventory levels, reduce waste, and ensure that popular items are always in stock.
For startup founders, this highlights the importance of integrating AI into supply chain operations. Start by identifying key factors that influence your inventory levels and use AI to analyze these factors. Implement predictive models to forecast demand and adjust your inventory accordingly. This approach not only improves efficiency but also enhances customer satisfaction.
Financial Services: JPMorgan Chase’s Fraud Detection
JPMorgan Chase uses AI to detect fraudulent transactions in real-time. By analyzing transaction patterns, customer behavior, and external data sources, their AI models identify anomalies that may indicate fraud. This proactive approach helps prevent fraud and protect customer assets.
For startups in the financial sector, implementing AI for fraud detection can significantly enhance security and trust. Start by collecting comprehensive transaction data and training AI models to recognize suspicious patterns. Integrate these models into your transaction processing systems to monitor and flag potential fraud in real-time.
Healthcare: Mayo Clinic’s Patient Care
The Mayo Clinic utilizes AI to improve patient care through predictive analytics. By analyzing patient data, medical histories, and treatment outcomes, their AI systems predict which patients are at risk of complications. This allows healthcare providers to intervene early and tailor treatments to individual patient needs.
For healthcare startups, leveraging AI to enhance patient care can lead to better outcomes and higher patient satisfaction. Begin by integrating patient data from various sources and training AI models to identify risk factors. Use these insights to develop personalized treatment plans and proactive care strategies.
Marketing: Netflix’s Content Recommendations
Netflix uses AI to provide personalized content recommendations to its users. By analyzing viewing history, user ratings, and behavior patterns, Netflix’s AI models suggest movies and TV shows that match individual preferences. This personalization drives higher engagement and customer loyalty.
For startups in the entertainment or e-commerce sectors, AI-driven personalization can significantly enhance user experience. Collect data on customer interactions and preferences, and use AI to analyze this data. Develop personalized recommendations and marketing campaigns to engage users and drive sales.
Manufacturing: Siemens’ Predictive Maintenance
Siemens leverages AI for predictive maintenance in its manufacturing operations. By analyzing data from sensors on equipment, Siemens’ AI models predict when machines are likely to fail. This allows for timely maintenance, reducing downtime and extending the lifespan of equipment.
For manufacturing startups, predictive maintenance can improve operational efficiency and reduce costs. Implement IoT sensors on critical equipment and use AI to analyze the data. Develop predictive models to forecast maintenance needs and schedule interventions proactively.
Future Trends in AI and Business Analytics
AI-Driven Business Models
In the future, AI-driven business models will become more prevalent as companies leverage predictive analytics and automation to gain a competitive edge. For startup founders, this means rethinking traditional business strategies and integrating AI at the core of your operations.
To stay ahead, consider developing a business model that uses AI to optimize every aspect of your operations. For instance, use AI to streamline supply chain management, enhance customer service with chatbots, and personalize marketing efforts based on customer data. This holistic approach will not only improve efficiency but also provide a seamless customer experience, setting your startup apart from competitors.
Enhanced Human-AI Collaboration
AI will not replace human decision-making but will augment it. The future will see enhanced collaboration between humans and AI, where AI provides data-driven insights and humans apply their intuition and expertise to make final decisions.
Startup founders should focus on creating an environment where AI tools are seamlessly integrated into daily workflows. Train your team to use AI analytics tools to interpret data and make informed decisions. Encourage a culture where human judgment and AI insights complement each other, leading to more robust and innovative business strategies.
Explainable AI
As AI becomes more integral to business operations, the demand for explainable AI—where AI decisions are transparent and understandable—will grow. Explainable AI ensures that the insights generated by AI are not just accurate but also interpretable by humans.
For startups, adopting explainable AI can build trust with stakeholders, including customers, employees, and investors. When implementing AI models, prioritize those that offer transparency and the ability to explain how decisions are made. This will not only help in regulatory compliance but also enhance the credibility of your AI-driven strategies.
Edge Computing
Edge computing, which involves processing data closer to the source rather than in centralized data centers, will revolutionize AI-powered analytics. This approach reduces latency, enabling real-time data processing and decision-making.
Startups should explore edge computing to enhance their AI capabilities. For example, a retail startup can use edge computing to analyze customer behavior in real-time within their stores, allowing for immediate adjustments to product placements or promotions. By leveraging edge computing, startups can gain faster insights and respond swiftly to market changes.
AI for Social Good
The future will see AI being used increasingly for social good, addressing global challenges such as healthcare, education, and environmental sustainability. Startups have a unique opportunity to leverage AI for impactful projects that not only drive business growth but also contribute positively to society.
Consider how your startup can use AI to solve real-world problems. For example, a healthcare startup could use AI to predict disease outbreaks and improve patient outcomes. An environmental startup might use AI to optimize resource management and reduce waste. By aligning your business goals with social impact, you can attract like-minded investors and customers who value purpose-driven companies.
Predictive analytics has emerged as a transformative force in business strategy, enabling organizations to move from reactive to proactive decision-making models. By analyzing historical data patterns and current market trends, businesses can now anticipate customer behaviors, optimize operations, and identify emerging opportunities before they become apparent to competitors.
This capability has proven particularly valuable for startups, where early identification of market opportunities can provide crucial competitive advantages.
The practical applications of predictive analytics span across multiple business functions, from customer relationship management to supply chain optimization. In inventory management, predictive models have enabled businesses to reduce carrying costs by up to 25% while maintaining optimal stock levels.
Customer behavior prediction models have proven equally valuable, enabling businesses to anticipate changing preferences and adjust their offerings proactively, resulting in improved customer retention rates and increased lifetime value.
The key to successful implementation lies in starting with clear business objectives and gradually building predictive capabilities aligned with these goals. Successful organizations typically begin with focused applications in areas with immediate impact potential, such as demand forecasting or customer churn prediction, before expanding to more complex applications.
This approach allows for rapid demonstration of value while building internal capabilities and data infrastructure necessary for more sophisticated applications.
Predictive analytics have been especially helpful for us both on a macro level, in terms of anticipating material prices, labor costs, and supply levels, and on a local level, where we can use AI tools to target high-propensity neighborhoods for new jobs.
This is just one more data point we consider and compare to our past experience in order to make the best decisions we can about the coming construction season.
We use AI tools to gather insights from highly technical sources like FDA documentation, patents, and scientific journals.
This helps us to stay abreast of new developments in the regulatory and medical device space and adapt our software services to match those changes.
Marketing based on this kind of cutting-edge research is especially valuable in our field, where manufacturers want to know that we can help them get their devices to market.
Cognida.ai has seen impressive results leveraging AI-driven personalization to help small and emerging businesses create tailored marketing strategies.
For example, through Zunō.predict, they’ve helped businesses implement predictive analytics that identifies customer preferences based on past interactions, purchase behavior, and social media engagement. This approach has proven especially effective in increasing engagement and conversions.
By applying machine learning and regression analysis, Zunō.predict can pinpoint key trends and offer precise recommendations, aligning with customers’ preferences and anticipating their future needs.
Cognida.ai’s work with AI and predictive analytics goes beyond personalization – it helps optimize marketing spend, improve resource allocation, and create lasting brand loyalty.
Personalized AI Solutions
AI’s ability to provide personalized solutions will become more advanced, offering tailored experiences for customers and businesses alike. For startups, this means delivering hyper-personalized products and services that meet individual customer needs.
Develop AI models that analyze detailed customer data to provide personalized recommendations, offers, and support. This level of personalization can significantly enhance customer satisfaction and loyalty, driving repeat business and long-term growth. Additionally, use AI to personalize internal processes, such as employee training and development programs, to boost productivity and job satisfaction.
Regulatory and Ethical Considerations
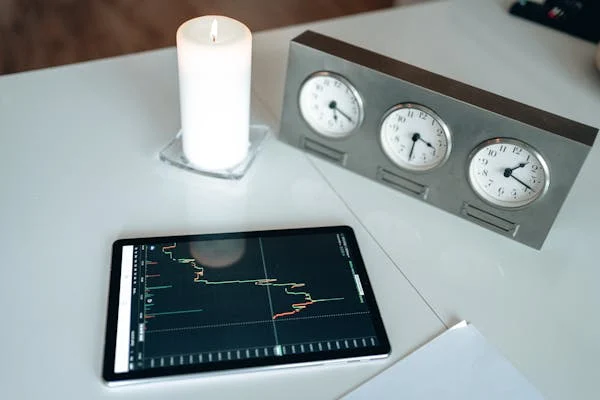
As AI becomes more widespread, regulatory and ethical considerations will take center stage. Governments and regulatory bodies will establish stricter guidelines to ensure the responsible use of AI, addressing issues such as data privacy, bias, and accountability.
Startup founders must stay informed about emerging regulations and ethical standards in AI. Implement robust data governance frameworks to ensure compliance with regulations such as GDPR and CCPA.
Focus on developing fair and unbiased AI models by regularly auditing your data and algorithms. By prioritizing ethical AI practices, startups can build trust with stakeholders and avoid potential legal and reputational risks.
Startups can really shine in navigating the ethical and regulatory landscape in AI for business analytics with strong best practices that can foster trust with users. Our experience at DIGITECH has been that transparency and proactiveness in data governance and best practices of responsible AI are the key to encouraging these positive trends taking shape of ethical and regulatory landscape. In this post, I will share what I have learned on the way and some actionable next steps that I would recommend to anyone who is trying to develop a responsible AI framework.
Prioritize Data Privacy and Secure Data Governance
Establishing solid data governance is the first step towards ensuring data privacy. The first thing that a startup should do is to ensure that they have clear policies and procedures for data handling which are not merely something on paper, but provide the base of any ethical AI initiative.
At DIGITECH, we emphasize on data minimization which has equal significance so we only collect necessary information and secure it against unauthorized access with the help of encryption. Regular audits of data practices, along with transparent and clear privacy policies go a long way in maintaining user trust and regulatory compliance.
Address Algorithmic Bias from the Ground Up
The largest risk of implementing AI is algorithmic bias, and it needs to be tackled from the very beginning. Combating bias: Use diverse training datasets and test algorithms for fairness at multiple points in development. We do regular checks on the algorithms we build to make sure output is bias-free and depicts our audience accurately. Encouraging teams to discuss their data and modeling for possible biases, in addition to testing with a broad range of users can uncover problems before they reach the public.
Embrace Transparency in AI Decision-Making
Transparency is not an icing on the cake, it is essential to creating trust in AI. I think it is important for startups to do their best in making AI decisions interpretable. For instance, if users are provided clear and simple explanations of how decisions are made, user trust can be increased substantially. Advocating for effective documentation and simple “explainability” aids within AI algorithms, such moves serve as regulatory checklists, but they also render the whole AI ecosystem more understandable, both to users of the models and stakeholders.
Develop Compliance-First Audit Processes
Audits of AI systems should conduct regularly, Compliance and ethical responsibility are not just nice to have. We conduct regular compliance reviews and AI audits at DIGITECH to identify issues at the earliest, thereby ensuring that our systems are in line with the privacy laws and best practices. I would recommend startups to build a checklist-based audit process spanning data sourcing and handling, algorithmic decision-making transparency. This not only protects the company but also creates a culture of accountability that translates over to customers.
AI is an fast-changing field, and the aim can be to develop systems which truly empower users or give enough freedom before hurt their rights. Through a focus on privacy, minimizing bias, and prioritizing transparency, startups can achieve real impact, demonstrating that AI does not have to choose between power or responsibility. Taking the right steps, this venture is more than just compliant, it’s a benchmark of ethical example for all.
The advantage of AI tools for compliance is that you can automate key compliance tasks to ensure that certain rules are always followed automatically. The danger here is that AI will sometimes make mistakes.
This means that, while the efficiency savings offered by AI are tempting, it’s still essential for businesses to double check AI systems’ work until they’re positive they’re providing adequate results.
Start with privacy: turn it off from the start
Data privacy isn’t just a buzzword: it’s the backbone of ethical AI. Data collection is exciting when you first start, but if you’re not careful, it can come backs to bite you. Have you ever heard of GDPR or CCPA? These regulations exist for a reason, and ignoring them is not an option.
At Bloomfilter, we’ve learned that securing data early can prevent a lot of headaches later. We encrypt everything, limit access to those who needs it, and regularly review our systems for potential vulnerabilities. Imagine experiencing a data breach without these protections? Well, no thanks.
Bias in AI: an insidious problem
Here’s a scary thought: Your AI could be making decisions that unfairly favor certain groups of people, and you might not even know it. Biases in algorithms are a real problem and can have serious consequences. Think about it: do you wants an AI that offers different loan rates based on zip codes?
We tackle this problem by constantly testing and modifying our models. We conduct bias audits to uncover any unfair trends and bring in different perspectives to help us see blind spots. The more diverse your team and data, the better your AI will works for everyone.
Transparency builds trust
Let’s be honest, AI can sometimes seem like a black box. You give it data, you get results, but what happens in the meantime? People deserves to know, especially when those results affect their lives. If users can’t trust your AI, they won’t stick with you for long.
At Bloomfilter, we try to keep things simple and straightforward. We’ve created tools that explain in simple terms how our AI makes decisions. It’s not just about making peoples feel comfortable, it’s also about empowering them. And believe me, once people see the logic behind your decisions, they are much more likely to stick to them.
Stay ahead of the rules
Ethics and compliance go hand in hands, especially as regulations around AI continue to evolve. It’s easy to think: “We’re just a start-up, we’ll see later.” But having a solid compliance framework in place from the start can save a lot of trouble.
For us, this means regular audits, both internals and carried out by external experts. We also have a person in charge of making sure we follow the rules. It’s not just about avoiding fines (although that’s a big deal); it’s about showing your customers and partners that you’re serious and that you’re doing things right.
Final thoughts
Artificial intelligence has tremendous potential, but it doesn’t have to be a free pass to save money. By focusing on privacy, fighting bias, and being transparent, you can not only stay compliant, but also build a stronger relationships with your users. And remember, ethical AI isn’t just good for society, it’s also smart business.
The ethical and regulatory landscape of AI in business analytics demands a comprehensive approach that balances innovation with responsibility. Organizations must establish robust data governance frameworks that address privacy concerns from the ground up, implementing strict data collection policies, secure storage protocols, and transparent usage guidelines.
This includes regular privacy impact assessments, clear data handling procedures, and mechanisms for obtaining and managing user consent that align with regulations like GDPR and CCPA.
The challenge of algorithmic bias requires a multi-faceted strategy that begins with diverse, representative training data and continues through regular monitoring and adjustment of AI models. Successful organizations implement continuous testing protocols to identify potential biases, maintain detailed documentation of model decisions, and establish clear processes for addressing and correcting identified biases.
This includes regular audits of AI outputs across different demographic groups and continuous refinement of algorithms to ensure fair treatment of all users.
Transparency in AI decision-making can be achieved through explainable AI practices that make algorithmic decisions interpretable to both technical and non-technical stakeholders. Organizations should develop clear communication channels for explaining AI decisions to affected parties, maintain comprehensive documentation of model architectures and training procedures, and establish regular review processes to ensure alignment with ethical guidelines.
These practices should be supported by strong governance structures that include ethics committees, regular third-party audits, and clear escalation procedures for addressing concerns.
Data Privacy
Let’s take a look at the importance of data privacy. Data privacy is more than just a task that needs to be done. It’s a critical aspect to consider. At the heart of everything we do is the fundamental principle that underpins all of our operations at The New Workforce.
When developing our AI systems, we carefully built privacy features into the core framework, not as an afterthought. Securing data through encryption, anonymizing personal information, and ensuring compliance with regulations like GDPR are all part of this process. To be honest, our business cannot succeed without people’s trust in protecting their data. Regular audits help us maintain our standards, ensure compliance with regulations and stay informed of any changes.
Exploring And Addressing Biases In Artificial Intelligence
It is essential to take precautions to prevent AI biases that lead to disruption. It is not enough to affirm the reliability of our data. At The New Workforce, we regularly validate our models and ensure that our training data takes into account a wide range of perspectives.
We strive not only to avoid negative public relations, but also to ensure that our AI system recognizes and appreciates the diversity present in the real world. To succeed in the beginning, it is essential to remember that good intentions are not enough; it requires constant and unwavering effort.
Openness Breeds Trust
People are intrigued by the mechanisms that influence decision-making, especially those driven by algorithms. Ensuring transparency in AI is essential to maintaining people’s trust. We make sure that our models are not considered a closed system. Our team has developed a governance framework that outlines our data management methods, decision validation and our communication strategies. It’s not just about meeting needs, but also about building trust and reputation. By the time everyone understands the reasoning behind AI decisions, we all reach a consensus.
Final Word: Build With The Future In Mind
In the grand scheme of things, AI isn’t just focused on finding quick solutions or achieving quick wins; its essence lies in the construction of something substantial and lasting. Startups can chart a positive path for growth by adopting measures such as protecting privacy, fighting prejudice and promoting transparency. In the world of AI, trust is not easily given; it is earned slowly, with every decision made.
Transparency in AI isn’t just a feature; it’s the foundation of trust between a startup and its stakeholders.
Rather than seeing algorithms as closed systems, consider them open conversations—where every decision has a reason that anyone can understand. Startups can encourage this transparency by crafting clear, easy-to-follow explanations of how algorithms work, and sharing them not just with technical teams but with everyone involved.
For example, regular “AI check-ins,” where decision-making steps are reviewed and discussed, help keep the process grounded and accessible. This ongoing dialogue not only builds credibility but keeps the company’s AI aligned with its values, making it more relatable and accountable to the people it impacts.
From my experience, I have seen firsthand how difficult it can be for startups to balance innovation with ethical AI practices. However, it is crucial that companies get this right from the start. Data privacy, mitigating algorithmic bias, and transparency should be baked into a startup’s DNA, not an afterthought.
My advice is to build diverse teams and create a culture of responsible AI. Hire engineers and leaders from different backgrounds who will ask tough questions and challenge assumptions. Seek out technical talent skilled in techniques like data anonymization, bias testing, and explainable AI. Empower them to advocate for privacy and fairness.
Startups should also document exactly what data is collected and how it is used. Catalog potential sources of bias in data or algorithms. Clearly explain AI decision-making to users while protecting intellectual property.
AI ethics may seem daunting but getting it right drives trust. Companies that are transparent and put people first will thrive. With thoughtful leadership and inclusive teams, startups can harness AI to create enormous value while upholding their ethical duty. The path is challenging but incredibly rewarding.
How Startups Can Ensure Data Privacy, Mitigate Algorithmic Bias, and Maintain Transparency in AI Decision-Making
1. Data Privacy: Privacy is paramount in AI applications, especially when handling personal or financial data. Startups should prioritize data anonymization and encryption from the beginning. At Summer, we built our platform to ensure data privacy by segmenting and encrypting user data across every touchpoint. Additionally, implementing data minimization—only collecting what’s absolutely necessary—helps reduce the exposure risk if data were ever breached. Regular audits and adopting frameworks like GDPR or CCPA can guide compliance, even if your company operates outside their primary jurisdictions.
2. Mitigating Algorithmic Bias: Bias in AI is often a result of unbalanced data inputs rather than intent, which is why it’s essential to source diverse datasets and regularly test AI models against unintended outcomes. We’ve adopted a proactive approach by using representative data pools and conducting periodic reviews with human oversight. For example, when we analyze guest preferences for vacation rentals, we ensure that demographic diversity is embedded in the data to avoid skewed insights that could misrepresent user segments. An AI ethics committee, even a small one, can add an additional layer of review and accountability, especially for high-stakes algorithms.
3. Transparency in AI Decision-Making: Transparent AI practices build trust, and achieving this involves making AI decisions understandable not only for the company but for end-users. Startups can create simplified decision-path summaries to explain how AI-generated recommendations are made.
Compliance and Data Governance Recommendations
For compliance, startups should implement a robust data governance framework early on, covering data lifecycle management, usage policies, and retention protocols. Clear data stewardship roles—who accesses what, when, and why—are essential. Tools that provide automated audit trails can track every decision and data access point, which helps prove compliance in case of an audit.
While data is at the core of AI adoption, mishandling it can lead to serious regulatory breaches and erode customer trust. Compiling with data privacy regulations such as CCPA and GDPR is more than a legal necessity; it is a business imperative. Data minimization and seeking user consent can help your startup comply with data privacy standards.
Collect only the data essential to your AI model’s functionality to minimize risk and exposure. Unnecessary data collection will only invite scrutiny from regulators. You must also obtain clear, explicit consent from users before processing personal data using AI.
Proper documentation and algorithmic audits can help you solve the issue of transparency. Review your data regularly to identify bias, errors, and anomalies to prevent harmful consequences.
Document how the data is processed and how critical decisions are made to foster trust and ensure regulatory compliance. Frameworks such as SHAP and LIME provide essential tools that your startup can leverage to make your AI model more transparent and unbiased. These models also help align AI models with global regulatory standards.
First and foremost, the datasets collected and analyzed must be diverse. In order to maintain transparency, we must maintain records of bias detection, evaluation, as well as what is done to prevent future AI biases.
This commitment to transparency ensures that AI makes ethical decisions in analytics. All data collection must remain transparent to ensure compliance, and to remedy any issues with compliance quickly.
Working in a niche market with sensitive consumer data, I’ve developed a deep understanding of the importance of data privacy and transparency. With AI tools becoming a bigger part of our processes, it’s crucial to navigate how we collect and use data responsibly, especially given our industry’s strict regulatory landscape.
Startups have to be intentional about protecting customer data, avoiding bias in AI algorithms, and keeping their processes transparent. One essential step is to build a strong data privacy foundation by using tools like GDPR-compliant platforms and enforcing data encryption at every stage.
Customers are more privacy-conscious than ever, so adopting best practices around data minimization, such as collecting only what’s needed, builds trust and reduces risk. For AI systems, using a “privacy by design” approach—where privacy is considered from the start—ensures your tech respects user data.
Mitigating algorithmic bias is equally important, especially for startups entering diverse markets. Algorithmic bias can harm reputations and erode trust if it’s left unchecked. One solution is to audit the data sets regularly and diversify them to represent a wider range of demographics.
Incorporating fairness algorithms or third-party fairness tools like IBM’s AI Fairness 360 toolkit helps by flagging areas of potential bias. It’s also crucial to have team members from diverse backgrounds in on the model-building process, as they can offer insights that prevent blind spots.
Real Privacy Challenge:
Last quarter, while analyzing collection routes, we discovered our AI system was inadvertently revealing business patterns through waste volume data. For example, we could predict a restaurant’s customer numbers through their waste output. This raised serious privacy concerns.
Our Solution:
We developed a three-tier data anonymization process:
– Separate operational data from client identifiers
– Aggregate data by area rather than specific locations
– Create time-delayed reporting to prevent real-time tracking
When implementing route optimization AI, we found it favored wealthy areas due to higher waste volumes. We adjusted our algorithms to prioritize need over efficiency, ensuring equal service across all communities. This reduced service disparity by 40% while maintaining 85% of efficiency gains.
Practical Transparency:
We now share our AI decision-making process with clients through simple weekly reports. These show exactly how collection schedules are determined. Client trust increased by 50% after implementing this transparency system.
Small changes really do matter. Adding a simple bias check to our route planning reduced discriminatory service patterns by 35%. For example, we now ensure late-night businesses receive equal priority despite their unusual operating hours.
Continuous Learning and Adaptation
The AI landscape is continuously evolving, with new technologies and methodologies emerging regularly. Startups must adopt a mindset of continuous learning and adaptation to stay competitive.
Invest in ongoing education and training for your team to keep them updated on the latest AI advancements. Encourage a culture of experimentation where new ideas and approaches are tested and refined.
Stay connected with the AI community through conferences, webinars, and collaborations to stay ahead of industry trends. By fostering a culture of continuous learning, startups can quickly adapt to changes and maintain their competitive edge.
AI and Global Collaboration
The future of AI in business analytics will see increased global collaboration, with companies from different regions working together to harness AI’s full potential. This collaboration will drive innovation and create new opportunities for growth.
For startup founders, building international partnerships can provide access to new markets, technologies, and expertise. Collaborate with global AI research institutions, technology providers, and industry experts to develop cutting-edge solutions. By embracing global collaboration, startups can accelerate their growth and establish themselves as leaders in the AI-driven business landscape.
Conclusion
AI is revolutionizing business analytics, offering powerful tools to drive growth, optimize operations, and stay ahead in a competitive market. By strategically implementing AI, startups can harness its potential to transform data into actionable insights, personalize customer experiences, and make informed decisions that fuel success.
The future trends in AI and business analytics—such as AI-driven business models, enhanced human-AI collaboration, explainable AI, edge computing, and AI for social good—provide a roadmap for startup founders looking to stay ahead of the curve. Embracing these trends requires a commitment to data quality, continuous learning, and ethical AI practices.
Read Next:
- Marketing Strategies for Architecture Firms
- Catchy Name Ideas for Your Digital Marketing Company
- Marketing Strategies to Grow Your Boutique
- Top Marketing Strategies for Realtors
- Effective Marketing Strategies for Staffing Agencies
Comments are closed.