Data analytics has changed a lot over the years. With more data available than ever, businesses are looking for ways to make sense of it all. Generative AI, a branch of artificial intelligence, is now playing a big role in this field. It helps in creating new data and finding patterns that were hard to spot before. This article will explore the latest trends in using generative AI for data analytics, providing insights into how it works and how it can benefit businesses.
What is Generative AI?
Generative AI refers to algorithms that can create new data from existing datasets. Unlike traditional AI, which focuses on recognizing patterns and making predictions, generative AI can produce new outputs that resemble the input data.
This is useful in many areas, including image and text generation, but it’s now making waves in data analytics too.
How Does Generative AI Work?
Generative AI uses models like Generative Adversarial Networks (GANs) and Variational Autoencoders (VAEs). These models learn the underlying structure of data and can generate new data points that are similar to the original.
This is particularly useful in scenarios where data is limited or where synthetic data can be used to augment real-world data for better analysis.
Emerging Trends in Generative AI for Data Analytics
Enhanced Data Quality
One of the biggest challenges in data analytics is dealing with poor-quality data. Generative AI helps by creating high-quality synthetic data.
This synthetic data can fill in gaps where data is missing, or where the existing data is noisy or inconsistent. As a result, the overall quality of the dataset improves, leading to more accurate analytics.
Advanced Predictive Analytics
Predictive analytics involves forecasting future trends based on historical data. Generative AI enhances this by generating new scenarios and data points that weren’t previously considered.
This provides a broader range of possibilities, leading to better-informed decisions. Companies can use these insights to anticipate market trends, customer behavior, and more.
Personalization and Customization
In marketing and customer service, personalization is key. Generative AI can analyze customer data to create personalized experiences.
For example, it can generate custom product recommendations, tailor marketing messages, or even create personalized content. This leads to better customer engagement and higher satisfaction rates.
Practical Applications of Generative AI in Data Analytics
Fraud Detection
Financial institutions use data analytics to detect fraudulent activities. Generative AI can enhance these systems by creating synthetic fraudulent data.
This helps in training models to recognize and respond to new types of fraud that haven’t been seen before. The result is a more robust fraud detection system that can adapt to evolving threats.
Healthcare Analytics
In healthcare, data analytics is used for everything from patient care to operational efficiency. Generative AI can create synthetic patient data, which can be used to train models without compromising patient privacy.
This is especially useful in developing new treatments and improving patient outcomes.
Market Research
Businesses rely on market research to understand consumer preferences and trends. Generative AI can simulate different market conditions and consumer behaviors.
This helps businesses to test new products, marketing strategies, and more in a virtual environment before launching them in the real world.
Integrating Generative AI with Traditional Data Analytics Tools
Combining Forces for Better Insights
Generative AI doesn’t work in isolation. It’s most effective when integrated with traditional data analytics tools. By combining the predictive power of traditional analytics with the creative capabilities of generative AI, businesses can gain deeper insights.
For instance, a company might use traditional analytics to identify trends in sales data, then use generative AI to simulate how different market conditions could impact future sales.
Real-Time Data Generation
Another exciting development is the ability to generate data in real-time. Traditional data analytics often relies on historical data, which can quickly become outdated.
Generative AI can create up-to-date data on the fly, helping businesses respond to changes more rapidly. This is particularly valuable in fast-moving industries like finance and retail, where staying ahead of trends is crucial.
Ethical Considerations and Challenges
Data Privacy Concerns
While generative AI offers many benefits, it also raises ethical concerns, especially around data privacy. Synthetic data generation must be handled carefully to ensure that it doesn’t inadvertently expose sensitive information.
Businesses need to implement robust privacy measures and adhere to regulations to protect individuals’ data.
Quality and Reliability of Synthetic Data
Another challenge is ensuring the quality and reliability of synthetic data. While generative AI can create realistic data, it’s not always perfect.
Businesses must validate synthetic data to ensure it accurately represents real-world conditions. This involves continuous testing and refinement of AI models.
Bias in AI Models
AI models can sometimes inherit biases present in the training data. Generative AI is no exception. If the input data is biased, the generated data will be too.
This can lead to skewed analytics and decision-making. It’s important to regularly review and update AI models to minimize bias and ensure fair outcomes.
Future Prospects of Generative AI in Data Analytics
Expanding Applications
The future of generative AI in data analytics looks promising. As technology advances, we can expect to see even more applications across various industries.
From enhancing supply chain management to optimizing energy consumption, the possibilities are vast. Businesses that stay ahead of these trends will be better positioned to leverage AI for competitive advantage.
Improved AI Models
Ongoing research and development are leading to more sophisticated AI models. Future models will be able to generate even more accurate and useful data, further enhancing the capabilities of data analytics.
These improvements will make generative AI an indispensable tool for businesses looking to innovate and stay ahead of the curve.
Democratization of AI Technology
As generative AI technology becomes more accessible, even small businesses will be able to benefit from it. Tools and platforms are emerging that make it easier to implement generative AI without needing extensive technical expertise.
This democratization will enable more businesses to harness the power of AI for data analytics, leveling the playing field.
Success Stories with Generative AI
Retail Industry
A major retail chain implemented generative AI to enhance its inventory management system. By generating synthetic sales data, the company was able to predict stock shortages and optimize its supply chain.
This led to a significant reduction in out-of-stock items and improved customer satisfaction.
Financial Services
A financial services firm used generative AI to improve its risk assessment models. By creating synthetic financial data, the firm was able to simulate various economic scenarios and better understand potential risks.
This allowed them to develop more robust strategies to mitigate those risks, enhancing their overall financial stability.
Healthcare
A healthcare provider used generative AI to create synthetic patient data for training purposes. This enabled the provider to develop more accurate diagnostic tools and improve patient care without compromising patient privacy.
The synthetic data also helped in training new medical professionals, ensuring they had a wealth of realistic scenarios to learn from.
Implementing Generative AI in Your Business
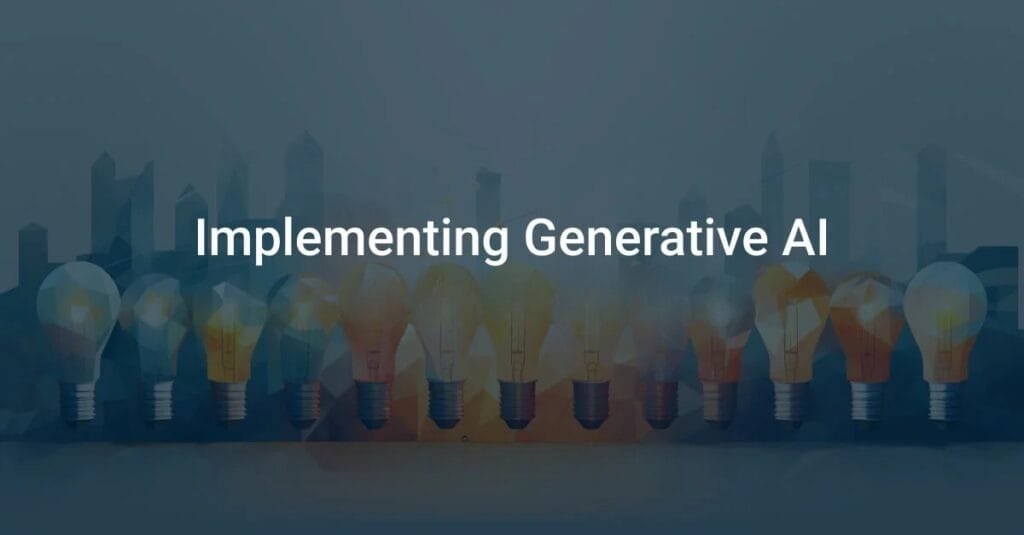
Getting Started with Generative AI
For businesses looking to implement generative AI, the first step is understanding the specific needs and goals. Identify areas where data quality can be improved or where new data generation could provide valuable insights.
This initial assessment will help in defining the scope and objectives of your generative AI projects.
Choosing the Right Tools and Technologies
There are various tools and platforms available for implementing generative AI. When selecting a tool, consider factors such as ease of use, scalability, and integration capabilities with your existing data analytics infrastructure.
Popular options include open-source frameworks like TensorFlow and PyTorch, as well as commercial solutions from companies like IBM and Microsoft.
Building and Training AI Models
Once you’ve selected your tools, the next step is building and training your AI models. This involves collecting and preparing your data, designing the architecture of your models, and training them using your datasets.
It’s important to monitor the training process and make adjustments as needed to ensure your models are accurate and reliable.
Integrating AI with Existing Systems
After your models are trained, the next step is integrating them with your existing data analytics systems. This may involve working with your IT team to ensure seamless data flow and compatibility.
The goal is to create a unified system where generative AI enhances your overall data analytics capabilities.
Monitoring and Maintenance
Implementing generative AI is not a one-time task. It requires ongoing monitoring and maintenance to ensure that your models continue to perform well.
Regularly updating your models with new data, retraining them as needed, and continuously testing their outputs are all critical to maintaining the effectiveness of your generative AI system.
Tips for Maximizing the Benefits of Generative AI
Focus on Quality Data
The quality of your input data has a significant impact on the performance of your generative AI models. Ensure that you are using clean, well-labeled data for training. This will help in producing more accurate and reliable synthetic data.
Start Small and Scale Up
When implementing generative AI, it’s often best to start with a small, manageable project. This allows you to test and refine your models before scaling up to larger, more complex applications.
As you gain experience and confidence, you can expand your use of generative AI to other areas of your business.
Collaborate with Experts
If you’re new to generative AI, consider collaborating with experts in the field. This could involve hiring data scientists, partnering with AI-focused companies, or even attending workshops and training sessions.
Expert guidance can help you avoid common pitfalls and accelerate your progress.
Stay Updated with Trends
The field of generative AI is evolving rapidly. Stay updated with the latest research, trends, and best practices. This will help you continually improve your models and stay ahead of the competition.
Participating in industry conferences, joining AI communities, and subscribing to relevant publications are great ways to stay informed.
The Road Ahead for Generative AI and Data Analytics
The Rise of Autonomous AI Systems
One of the most exciting prospects for the future is the development of autonomous AI systems. These systems can operate independently, making real-time decisions based on the data they generate and analyze.
This could revolutionize industries by enabling more efficient and responsive operations.
Increased Collaboration Between Humans and AI
As generative AI becomes more advanced, we’ll see increased collaboration between humans and AI. AI will handle more complex tasks, freeing up humans to focus on strategic decision-making and creative problem-solving.
This synergy will lead to more innovative solutions and greater business success.
Broader Adoption Across Industries
Generative AI is not limited to a few sectors. Its applications are broad and varied, from manufacturing and logistics to entertainment and education.
As more businesses recognize the benefits of generative AI, we’ll see widespread adoption across many different industries, driving growth and innovation.
Ethical and Regulatory Developments
With the growing use of generative AI, there will be increased focus on ethical and regulatory issues. Ensuring that AI is used responsibly, protecting data privacy, and addressing biases will be critical.
Governments and industry bodies will play a key role in shaping the regulations and standards that guide the use of generative AI.
Industry-Specific Applications of Generative AI in Data Analytics
Manufacturing and Supply Chain Management
In the manufacturing sector, generative AI is making a significant impact. It helps in optimizing supply chain management by generating synthetic data that simulates various supply chain scenarios.
This allows manufacturers to predict potential disruptions and plan accordingly, ensuring smooth operations. Generative AI can also enhance quality control by creating synthetic defect data, which helps in training better inspection models.
Retail and E-commerce
Retailers are leveraging generative AI to better understand consumer behavior and improve customer experiences. By analyzing purchase data, generative AI can create detailed customer profiles and predict future buying trends.
This enables retailers to personalize marketing strategies, optimize inventory management, and improve customer service. E-commerce platforms are using generative AI to generate realistic product descriptions and images, enhancing the online shopping experience.
Finance and Banking
In finance, generative AI is used for risk assessment, fraud detection, and investment forecasting. By generating synthetic financial data, banks can train models to identify unusual patterns that might indicate fraudulent activities.
This improves the accuracy and reliability of fraud detection systems. Generative AI also helps in stress testing financial models by simulating various economic scenarios, enabling better risk management and decision-making.
Healthcare and Pharmaceuticals
Generative AI is revolutionizing healthcare by generating synthetic patient data for research and training purposes. This helps in developing new treatments and improving patient outcomes without compromising privacy.
In pharmaceuticals, generative AI aids in drug discovery by simulating molecular structures and predicting how they will interact with the human body. This accelerates the development of new drugs and reduces costs.
Marketing and Advertising
Marketing and advertising firms use generative AI to create personalized content and advertisements. By analyzing customer data, generative AI can generate customized marketing messages that resonate with individual consumers.
This leads to higher engagement and conversion rates. Additionally, generative AI can simulate different advertising scenarios, helping marketers optimize their campaigns and achieve better results.
Overcoming Challenges in Implementing Generative AI
Ensuring Data Privacy and Security
One of the primary concerns when implementing generative AI is ensuring data privacy and security. Businesses must adopt robust data protection measures and comply with relevant regulations.
This involves using encryption, anonymization, and secure data storage practices. Regular audits and monitoring can help identify and address potential vulnerabilities.
Addressing Bias in AI Models
Bias in AI models can lead to unfair and inaccurate outcomes. It is crucial to regularly review and update AI models to minimize bias.
This involves using diverse and representative training data, testing models for bias, and implementing corrective measures as needed. Transparent reporting and accountability practices can also help in addressing bias.
Validating Synthetic Data
The quality of synthetic data generated by AI models is vital for accurate analytics. Businesses must validate synthetic data to ensure it accurately represents real-world conditions.
This involves comparing synthetic data with actual data, conducting thorough testing, and making adjustments as necessary. Continuous validation and improvement processes are essential for maintaining data quality.
Building In-House Expertise
Implementing generative AI requires specialized knowledge and skills. Businesses should invest in training and upskilling their workforce to build in-house expertise.
This can involve hiring data scientists, providing training programs, and fostering a culture of continuous learning. Collaborating with AI experts and consultants can also provide valuable insights and support.
The Future of Generative AI in Data Analytics
Integration with IoT and Edge Computing
The integration of generative AI with the Internet of Things (IoT) and edge computing is set to drive further advancements in data analytics.
IoT devices generate vast amounts of data in real-time. By integrating generative AI, businesses can analyze this data at the edge, closer to where it is generated. This enables faster decision-making and more efficient operations.
AI-Driven Business Intelligence
Generative AI will play a key role in AI-driven business intelligence. By automating data generation and analysis, businesses can gain deeper insights and make more informed decisions.
AI-driven business intelligence platforms will provide real-time analytics, predictive insights, and automated reporting, enhancing overall business performance.
Personalized AI Assistants
The future will see the rise of personalized AI assistants powered by generative AI. These assistants will analyze individual preferences and behaviors to provide customized recommendations and support.
From personal finance management to health monitoring, AI assistants will enhance various aspects of daily life, providing valuable insights and assistance.
Enhanced Human-AI Collaboration
As generative AI continues to evolve, the collaboration between humans and AI will become more seamless. AI will handle routine and data-intensive tasks, freeing up humans to focus on strategic and creative activities.
This enhanced collaboration will drive innovation, improve productivity, and lead to better business outcomes.
Leveraging Generative AI for Competitive Advantage
Creating a Data-Driven Culture
To fully leverage generative AI, businesses must foster a data-driven culture. This involves encouraging employees at all levels to use data in their decision-making processes.
Training programs and workshops can help staff understand the value of data analytics and how to use AI tools effectively. A data-driven culture promotes innovation and ensures that the insights gained from generative AI are utilized across the organization.
Continuous Learning and Adaptation
Generative AI and data analytics are fields that are constantly evolving. Businesses need to stay updated with the latest advancements and continuously adapt their strategies.
This can be achieved through regular training, attending industry conferences, and engaging with AI research communities. Continuous learning ensures that businesses can quickly adopt new technologies and methodologies, maintaining their competitive edge.
Collaborating with AI Startups and Innovators
Collaboration is key to staying ahead in the rapidly changing AI landscape. Partnering with AI startups and innovators can provide businesses with access to cutting-edge technologies and expertise.
These collaborations can lead to the development of innovative solutions that address specific business challenges. Additionally, working with AI thought leaders can inspire new ideas and approaches to data analytics.
Enhancing Customer Experiences with Generative AI
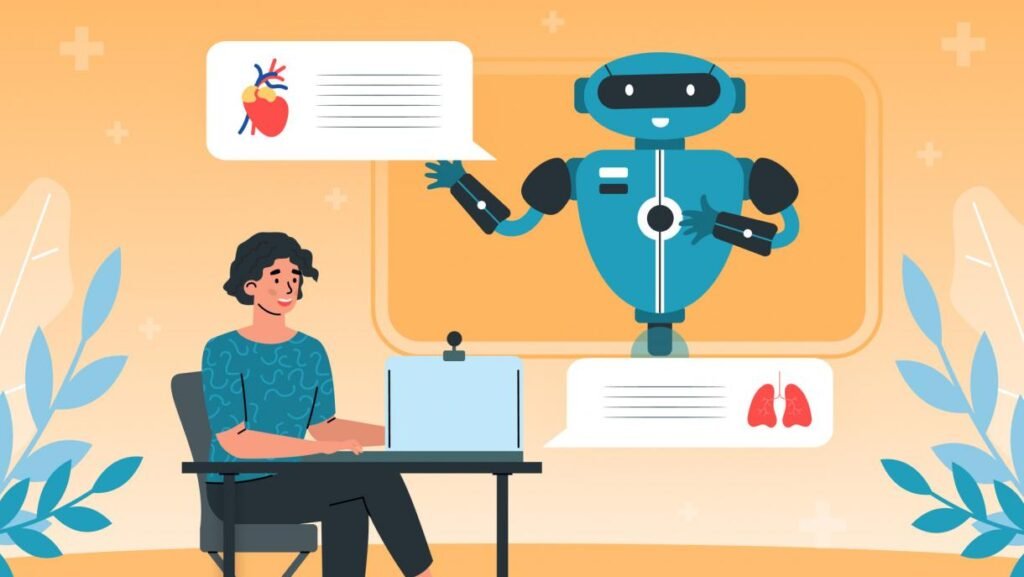
Personalized Customer Interactions
Generative AI enables businesses to create highly personalized customer interactions. By analyzing customer data, AI can generate personalized recommendations, tailor marketing messages, and customize products and services.
This level of personalization enhances customer satisfaction and loyalty, leading to increased sales and long-term business success.
Improving Customer Support
AI-powered chatbots and virtual assistants are transforming customer support. Generative AI can create more natural and human-like interactions, improving the customer experience.
These AI systems can handle a wide range of customer inquiries, providing quick and accurate responses. By automating routine support tasks, businesses can free up human agents to handle more complex issues, enhancing overall support efficiency.
Predicting Customer Needs
Generative AI can help businesses predict customer needs and preferences. By analyzing past behavior and generating new data, AI can identify trends and patterns that indicate future actions.
This allows businesses to proactively address customer needs, offering solutions before issues arise. Predictive insights from generative AI can also guide product development and marketing strategies, ensuring they align with customer expectations.
Ethical AI Practices and Governance
Establishing AI Ethics Committees
To ensure responsible use of generative AI, businesses should establish AI ethics committees.
These committees can oversee the development and deployment of AI systems, ensuring they adhere to ethical standards and regulations. By involving diverse stakeholders, including ethicists, legal experts, and community representatives, businesses can address potential ethical concerns and promote transparency.
Developing Ethical AI Guidelines
Businesses should develop clear ethical guidelines for the use of generative AI. These guidelines should cover data privacy, bias mitigation, and transparency.
By outlining best practices and standards, businesses can ensure their AI systems are used responsibly. Regular reviews and updates to these guidelines will help address emerging ethical issues and maintain public trust.
Promoting Transparency and Accountability
Transparency is crucial in building trust in AI systems. Businesses should be open about how their generative AI models work, the data they use, and the decisions they make.
Providing explanations for AI-generated insights and maintaining records of AI processes can enhance accountability. This transparency helps stakeholders understand and trust AI systems, fostering a positive relationship with technology.
Measuring the Impact of Generative AI
Key Performance Indicators (KPIs)
To measure the impact of generative AI, businesses should define key performance indicators (KPIs).
These KPIs can include improvements in data quality, accuracy of predictions, customer satisfaction, and operational efficiency. By tracking these metrics, businesses can assess the effectiveness of their AI initiatives and make data-driven decisions for continuous improvement.
Regular Audits and Assessments
Regular audits and assessments of generative AI systems are essential for maintaining their performance and reliability.
These audits can identify potential issues, such as biases or data quality problems, and provide insights for improvement. By conducting thorough assessments, businesses can ensure their AI systems remain effective and aligned with their goals.
Feedback Loops for Continuous Improvement
Feedback loops are crucial for the continuous improvement of generative AI systems. By collecting feedback from users and stakeholders, businesses can identify areas for enhancement.
This feedback can inform model updates, data refinement, and process improvements, ensuring the AI system evolves to meet changing needs and expectations.
Preparing for the Future of Generative AI
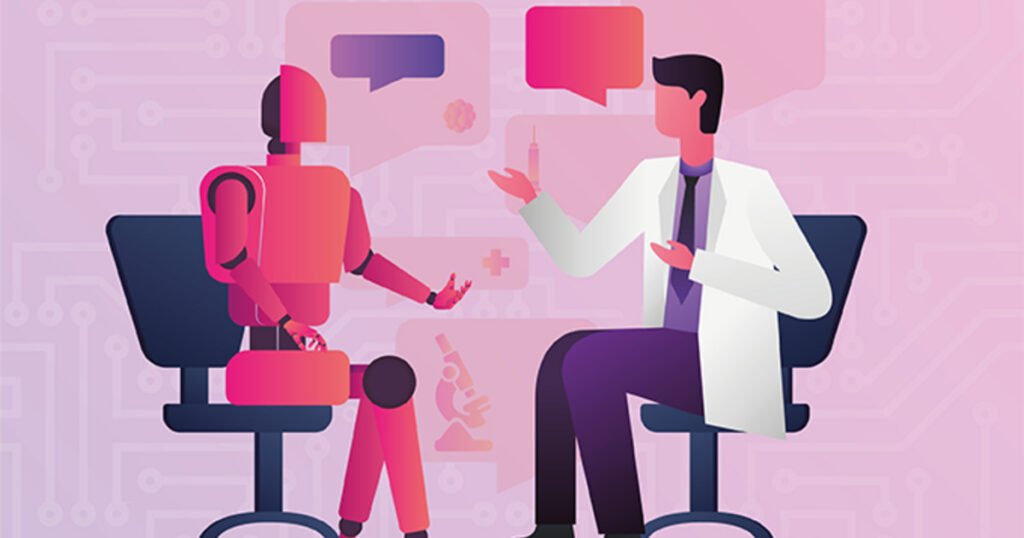
Investing in Research and Development
Investing in research and development is key to staying at the forefront of generative AI. Businesses should allocate resources to explore new AI technologies, develop innovative applications, and enhance existing systems.
R&D investments drive technological advancements and ensure businesses remain competitive in the AI-driven future.
Building Strategic AI Roadmaps
Developing strategic AI roadmaps helps businesses plan for the future.
These roadmaps should outline short-term and long-term AI goals, the steps needed to achieve them, and the resources required. By having a clear plan, businesses can align their AI initiatives with their overall strategy, ensuring cohesive and effective implementation.
Fostering an Innovation-Driven Mindset
An innovation-driven mindset is essential for leveraging the full potential of generative AI. Businesses should encourage creativity, experimentation, and risk-taking among their teams.
Creating an environment where new ideas are welcomed and explored can lead to groundbreaking innovations and drive business growth.
Wrapping it up
Generative AI is transforming data analytics by creating high-quality synthetic data, enhancing predictive capabilities, and personalizing customer interactions. While it presents challenges such as ensuring data privacy and mitigating bias, its benefits are substantial.
Fostering a data-driven culture, investing in research and development, and promoting ethical AI practices, businesses can leverage generative AI for competitive advantage. Embracing these emerging trends will enable businesses to thrive in the data-driven future, making informed decisions and enhancing customer experiences.
READ NEXT:
- Effective Marketing Strategies for Medical Practices
- Effective Marketing Strategies for Lash Technicians
- How to Market Your Pressure Washing Business
- Marketing Strategies for Financial Services
- How to Engage Your Community Through Marketing
Comments are closed.