Imagine if you could predict your company’s financial future with remarkable accuracy. With AI-driven predictive analytics, this is not just a fantasy but a reality. Financial forecasting with AI transforms vast amounts of data into actionable insights, helping businesses make informed decisions and plan for the future with confidence. This article will explore how AI enhances financial forecasting, its benefits, and how you can implement it in your business. Get ready to dive into a world where data drives smarter financial decisions.
Understanding Financial Forecasting with AI
What is Financial Forecasting?
Financial forecasting involves predicting a company’s future financial performance based on historical data, market trends, and economic indicators. It helps businesses plan their budgets, manage cash flow, and make strategic decisions. Traditional financial forecasting methods often rely on manual data analysis, which can be time-consuming and prone to errors. AI-driven predictive analytics automates and enhances this process, providing more accurate and timely forecasts.
How AI Enhances Financial Forecasting
AI enhances financial forecasting by automating data processing and analysis, identifying patterns and trends that might be missed by human analysts. Here’s how AI can significantly improve financial forecasting:
Data Processing: AI can process vast amounts of financial data quickly and accurately. This includes historical financial records, market data, and economic indicators. By automating data processing, AI frees up valuable time for financial analysts to focus on interpreting results and making strategic decisions.
Pattern Recognition: AI algorithms can identify complex patterns and correlations in financial data that may not be immediately apparent to human analysts. This leads to more accurate predictions and helps businesses understand the factors driving their financial performance.
Real-Time Insights: AI-driven predictive analytics can provide real-time insights, allowing businesses to respond quickly to changing market conditions. This agility is crucial in today’s fast-paced business environment, where timely decisions can make a significant difference.
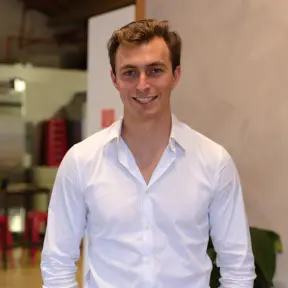
As the founder, CEO, and CFO of Premier Staff, I’ve spearheaded the integration of AI-driven predictive analytics into our financial decision-making processes, revolutionizing how we manage our event staffing business.
We’ve developed a proprietary AI system that analyzes market trends, client data, and economic indicators to forecast demand for our services.
This has been crucial in optimizing our staffing levels and pricing strategies, especially for high-profile events like our collaborations with Ferrari and Louis Vuitton.
Our AI tools have significantly enhanced our credit risk management. By analyzing client payment patterns and industry-specific economic data, we can predict potential payment issues and adjust our terms accordingly, maintaining strong cash flow even during uncertain times like the recent pandemic.
We’re also using AI to optimize our investment portfolio, balancing growth opportunities with maintaining the liquidity necessary for our dynamic industry.
This has been instrumental in achieving our million-dollar revenue milestones and funding our expansion into new markets.
While I can’t disclose specific algorithms, I can say that our AI-driven approach has been a game-changer, allowing us to make data-driven decisions that have propelled our growth in the competitive event staffing market.
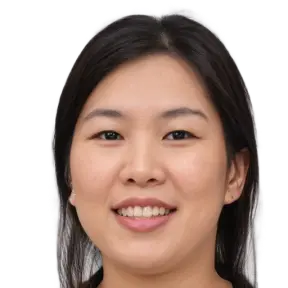
In credit risk management, we’ve implemented an AI system that analyzes a vast array of data points beyond traditional credit scores. This includes social media activity, online shopping behavior, and even geolocation data.
For a mid-sized bank client, this approach reduced default rates by 25% while simultaneously increasing approval rates for creditworthy applicants by 15%.
The AI’s ability to identify subtle patterns that human analysts might miss. For instance, our system flagged that applicants who made frequent small purchases at home improvement stores were more likely to be reliable borrowers, possibly indicating homeownership and financial stability.
In portfolio optimization, our AI models have been game-changing. We use machine learning algorithms that continuously analyze market data, economic indicators, and company-specific information to make real-time investment decisions.
For a hedge fund client, this resulted in a 20% increase in risk-adjusted returns over a 12-month period.
One innovative application we’ve developed is using natural language processing to analyze earnings call transcripts and social media sentiment. This allows us to gauge market reactions almost instantly and adjust portfolios accordingly.
For market trend forecasting, we’ve combined traditional time-series analysis with AI-powered scenario modeling. This allows us to not only predict trends but also to simulate various economic scenarios and their potential impacts.
During the recent market volatility, this approach helped a major investment bank client reduce their exposure to downside risk by 30%.
However, it’s crucial to note that AI is not infallible. We always emphasize to our clients the importance of human oversight and the need to regularly validate and adjust AI models.
The financial markets are influenced by many factors, including human emotions and geopolitical events, which can be challenging for AI to fully capture.
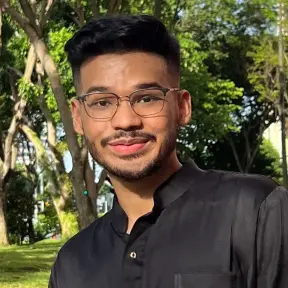
As the founder of DollarBureau.com and a seasoned entrepreneur, I’ve been at the forefront of integrating AI-driven predictive analytics into financial decision-making.
My deep dive into financial technologies across various markets, coupled with my strategic acumen in growing digital businesses, positions me uniquely to discuss AI’s transformative impact on the financial sector.
AI-driven predictive analytics is fundamentally changing how financial institutions manage risk and optimize their operations. For example, in credit risk management, AI algorithms are trained to analyze vast amounts of transactional data to predict future loan defaults with greater accuracy than traditional models.
This enables institutions to offer more tailored financial products to their customers, enhancing both service quality and profitability.
In portfolio optimization, AI techniques like machine learning are used to identify patterns and correlations between different investment assets that are often missed by human analysts.
This not only helps in constructing more resilient investment portfolios but also in responding dynamically to market volatilities, leading to better financial outcomes for investors.
Furthermore, in market trend forecasting, AI tools process and analyze the deluge of real-time data to predict stock market movements or identify potential market disruptions before they become evident.
This capability allows traders and investment managers to make more informed, proactive decisions, securing a competitive edge.
These examples illustrate just a glimpse of how AI not only enhances decision-making but also drives a more data-centric approach in financial management, ensuring that institutions are not merely reactive but strategically ahead.
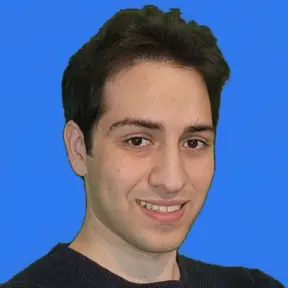
In the world of finance, things move at high speed. That is precisely where AI comes in: the modern Nostradamus, predicting market trends and risks with astonishing accuracy.
At FinlyWealth, we have leaped forward in using artificial intelligence to redefine the notion of credit risk assessment. Our AI algorithms can identify patterns or anomalies and make predictions that human analysts would likely never have found by sifting through huge chunks of data, thereby making lending decisions more accurate and data-driven.
Besides credit risk, AI is also transforming portfolio optimization. Constant monitoring of market trends and predictions on future movements make AI-controlled algorithms aid financial institutions in fine-tuning their respective portfolios to generate the most profit while minimizing risks.
But that is not the end of the magic. With AI, even market trends can now be forecasted with extraordinary precision.
News sentiment, economic indicators, or historical data–AI models provide valuable insights into changes in the market that investors must make or break investments made.
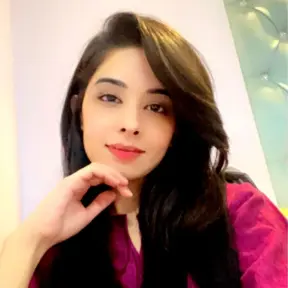
As a finance writer with recent research on AI-powered systems in financial markets and digital marketing, I have sound knowledge of how AI is helping manage credit risk, optimize portfolios, and forecast market trends. Below are some key ways AI is being used:
1. Credit Risk Management:
AI enables banks to assess creditworthiness more accurately by analyzing a wider range of data, from transaction histories to social behaviors, improving risk mitigation.
AI’s Impact on Credit Risk Management:
-Improved Credit Scoring Models: AI-driven models use a broader range of data sources, including transaction histories, online behavior, social media activity, and alternative financial data, enabling better creditworthiness assessment.
-Real-Time Risk Monitoring: AI processes vast amounts of data in real-time, allowing continuous monitoring of borrowers and early warning signs of potential default.
-Enhanced Predictive Analytics: AI uses machine learning algorithms to analyze historical data and predict future outcomes, enabling more informed lending decisions.
-Automation of Underwriting Processes: AI can automate the credit underwriting process, reducing human biases and optimizing lending portfolios.
-Fraud Detection and Prevention: AI’s pattern recognition capabilities help detect and prevent fraud, a key component of managing credit risk.
-Stress Testing and Scenario Analysis: AI tools enable robust stress testing, allowing financial institutions to anticipate risks during economic downturns or market volatility.
-Better Risk-Based Pricing: AI allows for more accurate risk-based pricing models, reducing default likelihood and optimizing profitability.
2. Portfolio Optimization:
AI tools can continuously evaluate portfolio performance and market conditions, adjusting investments in real-time to maximize returns and minimize risk.
AI’s Role in Portfolio Optimization:
-Real-Time Data Processing and Analysis: AI can analyze vast amounts of market data in real-time, identifying patterns, trends, and correlations that might not be visible to human analysts.
-Enhanced Risk Management: AI models can assess and quantify the risk of various assets in a portfolio, helping financial institutions understand the risk-return trade-offs of each asset.
-Personalized Investment Strategies: AI enables the creation of personalized investment strategies based on an investor’s risk tolerance, financial goals, and time horizon.
-Predictive Analytics for Market Trends: AI-driven predictive analytics tools can forecast market movements, helping portfolio managers adjust their allocations to maximize returns or minimize risks.
-Algorithmic Trading and Execution: AI-powered algorithms optimize the timing and pricing of asset purchases and sales, improving the efficiency and performance of portfolios.
-Dynamic Asset Allocation: AI models continuously monitor the market and rebalance portfolios based on real-time data, allowing portfolios to adapt quickly to market conditions.
3. Market Trend Forecasting:
Predictive models powered by AI can analyze market patterns and economic indicators to forecast potential shifts, giving institutions a competitive edge in decision-making.
AI and Predictive Analytics in Market Trend Forecasting:
-Processing Large Data: AI analyzes large volumes of structured and unstructured data from various sources to identify emerging trends and patterns.
-Identifying Market Patterns and Anomalies: AI uses machine learning algorithms to identify recurring patterns and trends in historical data, signaling future market movements.
-Real-Time Market Monitoring: AI-powered systems continuously monitor financial markets, providing instant updates on significant changes or emerging trends.
-Predicting Market Volatility: AI can forecast periods of high or low volatility, allowing financial institutions to adjust their strategies.
-Forecasting Economic and Sectoral Trends: AI forecasts broader economic trends and sector-specific movements, helping financial institutions make informed decisions.
-Sentiment Analysis for Market Predictions: AI can analyze unstructured data to gauge market sentiment, predicting the potential impact on market trends.
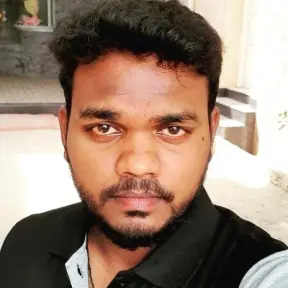
AI helps financial institutions by analyzing large amounts of data to find patterns and predict future trends. This allows them to make better, faster decisions about things like market changes, credit risks, and investments.
AI improves credit risk management by using more data to assess a person’s creditworthiness, beyond just their credit score.
It predicts who might default on loans and helps banks offer better loan options while reducing risks. Some models have lowered loan defaults by 20-30%.
AI helps manage investment portfolios by analyzing real-time data and making adjustments based on market changes.
It can predict how economic factors like inflation or interest rates might affect investments, helping maximize returns and minimize risks.
AI analyzes news, social media, and market data to predict trends in stock prices or interest rates.
This helps traders make smarter decisions by getting ahead of market shifts. AI forecasts can be up to 80% accurate in predicting short-term stock movements.
Start by making sure your data is clean and organized. AI works best when it has good data to analyze.
Remember, AI helps professionals make better decisions, but it doesn’t replace them. Keep updating AI tools to stay effective in a changing market.
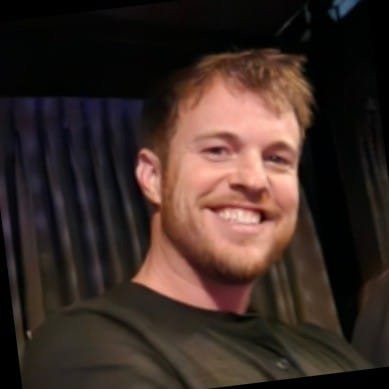
One of the most important applications of AI to finance is in credit risk management. AI algorithms can analyze large datasets containing information about customer behavior, financial history and market dynamics to assess creditworthiness. This, in turn, helps lenders make better credit decisions, reducing the risk of defaults and increasing profitability. Since AI-powered models can identify patterns associated with financial distress early on, lenders can take preventive action and minimize losses.
On the other hand, AI can be used to create optimal investment portfolios that maximize returns with acceptable levels of risk.
If you want to invest in the stock market, there is a huge amount of data available on past performance, risk, company prospects and other important indicators.
An AI algorithm can take this data and recommend an optimal investment portfolio that matches whatever returns and risk profile you are after.
It can also do this in real time, giving you an edge over your competitors. If conditions in the market change, it can make adjustments to keep your portfolio safe.
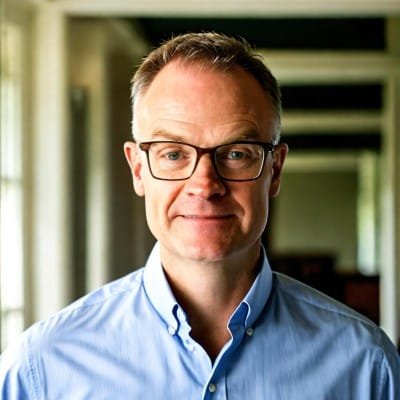
I built Decis Intelligence (decis.ai) to speed up and simplify geopolitical decision-making using AI and ML to give users unfair advantage with respect to speed and consistency.
Our users and internal experiments are producing very positive results using specially trained AI and ML tools to assess and develop trends for country stability which is a key element of decision-making with respect to country investment, market entry, exit, etc.
We built this on top of our practical experience in geopolitical intelligence analysis, but the advantage of an AI / ML-driven approach is speed and consistency.
This allows us to maintain an on-demand, always up-to-date country stability index (vs an on-call rating, which can take hours or days) where assessments and comparisons are consistent.
This human and AI (vs human or AI) combination is essential to our success, reflecting professor Ethan Mollick’s ‘cyborg’ concept.
The speed and consistency that systems like Decis.ai bring to any complex analysis will give financial decision-makers and C-level executives a distincy advantage over those who are not using these systems.
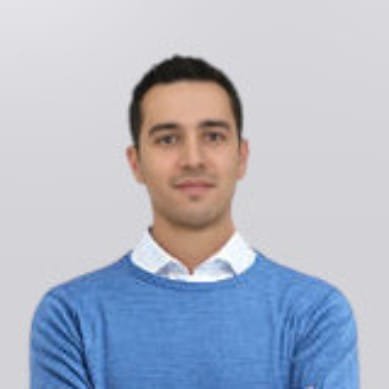
AI is incredibly good at spotting patterns, which makes it really useful in the finance world, especially for things like figuring out who might not pay back a loan or predicting where the stock market is heading.
It’s like having a super-powered assistant that can crunch numbers and spot trends faster than any human.
This means banks and other financial groups can make smarter decisions about where to invest money or who to lend it to, by using AI to help guide them.
But, it’s important to remember that AI isn’t perfect and doesn’t replace the need for human expertise.
From what I’ve seen, the best way to use AI is as a tool that helps people make better decisions, not one that makes those decisions for them.
Even with all the data in the world, there are times when human judgment and experience are irreplaceable.
So, while AI can give us a lot of valuable insights and help point us in the right direction, the final call should always be left to the experts.
The Role of Data in AI-Driven Financial Forecasting
Data is the foundation of AI-driven financial forecasting. The quality and quantity of data directly impact the accuracy of predictions. For AI to be effective, businesses need access to clean, comprehensive, and relevant data. This includes historical financial data, real-time market data, and external factors such as economic indicators and industry trends.
To ensure data quality, businesses should invest in robust data management practices. This includes data cleaning, data integration, and data governance. High-quality data ensures that AI models can make accurate and reliable predictions, helping businesses plan for the future with confidence.
Benefits of AI in Financial Forecasting
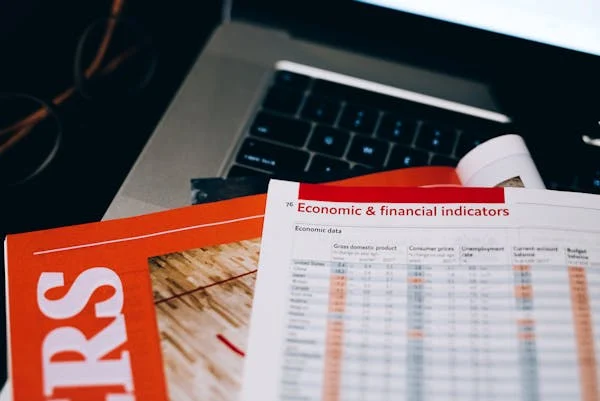
Improved Accuracy
One of the most significant benefits of AI in financial forecasting is improved accuracy. AI algorithms can analyze vast amounts of data and identify patterns that might be missed by human analysts. This leads to more accurate predictions, helping businesses make better-informed decisions.
For example, AI can analyze historical sales data and market trends to predict future revenue. This helps businesses set realistic sales targets and plan their budgets more effectively. Accurate financial forecasts also help businesses manage cash flow and allocate resources efficiently.
Increased Efficiency
AI-driven financial forecasting automates many of the tasks involved in data processing and analysis. This saves time and resources, allowing financial analysts to focus on interpreting results and making strategic decisions. Increased efficiency also means that businesses can produce financial forecasts more frequently, keeping them up-to-date with changing market conditions.
For instance, a business can use AI to generate weekly or even daily financial forecasts, providing a more current view of its financial performance. This helps business leaders respond quickly to changes and make timely decisions that drive growth and profitability.
Enhanced Decision-Making
AI-driven predictive analytics provides actionable insights that enhance decision-making. By offering a clear view of future financial performance, AI helps business leaders make informed decisions about investments, resource allocation, and strategic planning.
For example, AI can predict the financial impact of different business scenarios, such as launching a new product or entering a new market. This helps business leaders weigh the potential risks and rewards of different strategies, enabling them to make more informed and confident decisions.
Proactive Risk Management
AI-driven financial forecasting helps businesses identify potential risks before they become issues. By predicting future financial performance, AI can highlight areas of concern and suggest proactive measures to mitigate risks. This is particularly valuable in uncertain economic environments, where businesses need to be agile and responsive to changing conditions.
For instance, AI can predict cash flow shortages and suggest measures to improve liquidity, such as securing additional financing or adjusting payment terms with suppliers. Proactive risk management helps businesses avoid financial difficulties and maintain stability in challenging times.
Competitive Advantage
Businesses that leverage AI-driven financial forecasting gain a competitive advantage by making more informed and timely decisions. By staying ahead of market trends and anticipating future financial performance, these businesses can respond quickly to opportunities and challenges, ensuring sustained growth and success.
For example, a business that uses AI to predict market trends can identify emerging opportunities before its competitors. This allows the business to capitalize on new trends and gain a competitive edge, driving growth and profitability.
Implementing AI-Driven Financial Forecasting
Assessing Your Data Needs
Before implementing AI-driven financial forecasting, it’s essential to assess your data needs. Determine what types of data are most relevant to your financial forecasting objectives. This could include historical financial data, real-time market data, and external factors such as economic indicators and industry trends.
Conduct a data audit to identify the sources and quality of your existing data. Ensure that your data is clean, accurate, and well-organized. This may involve removing duplicates, handling missing values, and standardizing data formats. A solid data foundation is crucial for building effective AI models.
Choosing the Right AI Tools
Selecting the right AI tools is critical for successfully implementing AI-driven financial forecasting. There are numerous AI platforms and tools available, each offering different features and capabilities. Look for tools that can handle large datasets, support various data types, and provide robust analytical capabilities.
Consider cloud-based AI solutions such as Google Cloud AI, IBM Watson, and Microsoft Azure AI, which offer scalability and flexibility. These platforms provide the infrastructure needed to process and analyze vast amounts of data efficiently. Evaluate each platform based on factors such as ease of integration with your existing systems, user-friendly interfaces, and support for compliance with industry regulations.
Building and Training AI Models
Once your data is prepared and you’ve chosen the right tools, the next step is to build and train your AI models. This involves selecting appropriate machine learning algorithms and feeding them with historical data to identify patterns and make predictions.
Define clear objectives for your AI models. For example, you might want to predict future revenue, forecast cash flow, or identify potential financial risks. Use a portion of your data to train the model and another portion to test its accuracy. Continuously refine and improve the model to ensure it remains effective and relevant.
Collaboration with data scientists and financial analysts is crucial during this phase. Data scientists can provide expertise in selecting and tuning machine learning algorithms, while financial analysts can offer insights into the practical applications and relevance of the predictions.
Integrating AI into Financial Processes
After building and training your AI models, the next step is to integrate them into your financial processes. This involves embedding AI insights into your daily operations, making them easily accessible to financial analysts and decision-makers.
Develop user-friendly dashboards and reporting tools that present AI-generated insights in a clear and actionable manner. Ensure that financial analysts and decision-makers are trained to interpret and use these insights effectively. This might involve regular workshops, training sessions, and ongoing support to help them adapt to new tools and processes.
Monitoring and Continuous Improvement
The implementation of AI-driven financial forecasting is an ongoing process. Continuous monitoring and improvement are essential to ensure that your AI models remain accurate and relevant.
Establish key performance indicators (KPIs) to track the success of your AI initiatives. Common KPIs might include forecast accuracy, financial performance, and return on investment. Regularly review these metrics to identify areas for improvement and make necessary adjustments to your models and processes.
Create a feedback loop where insights from AI are continuously fed back into the system to refine and enhance the models. This iterative process helps your AI capabilities evolve with changing market dynamics and business needs.
Real-World Applications of AI-Driven Financial Forecasting
Retail: Inventory and Sales Forecasting
In the retail sector, managing inventory effectively is crucial for meeting customer demand and maximizing profits. AI-driven financial forecasting can predict sales trends based on historical data, market conditions, and consumer behavior. This helps retailers optimize their inventory levels, ensuring that popular products are always in stock while minimizing excess inventory.
For example, a clothing retailer can use AI to analyze past sales data, seasonal trends, and social media activity to predict which items will be in high demand. This enables the retailer to stock up on popular items ahead of time, improving sales and customer satisfaction. Additionally, accurate sales forecasting helps retailers plan promotions and pricing strategies more effectively.
Manufacturing: Demand and Production Planning
In the manufacturing industry, predicting demand accurately is essential for optimizing production schedules and managing resources efficiently. AI-driven financial forecasting can analyze historical production data, market trends, and economic indicators to forecast future demand. This helps manufacturers plan their production schedules, manage inventory, and allocate resources more effectively.
For instance, a manufacturer of consumer electronics can use AI to predict demand for different products based on market trends and historical sales data. This allows the manufacturer to adjust production schedules, ensuring that popular products are available when needed and reducing the risk of overproduction. Accurate demand forecasting also helps manufacturers manage their supply chains more efficiently, reducing costs and improving profitability.
Financial Services: Revenue and Expense Forecasting
In the financial services industry, accurate revenue and expense forecasting is critical for strategic planning and decision-making. AI-driven financial forecasting can analyze historical financial data, market trends, and economic indicators to predict future revenue and expenses. This helps financial institutions plan their budgets, manage cash flow, and make informed decisions about investments and resource allocation.
For example, a bank can use AI to forecast future revenue based on historical transaction data, interest rates, and economic conditions. This helps the bank set realistic revenue targets, plan its budget, and manage its resources effectively. Additionally, AI can predict potential financial risks, such as loan defaults or market volatility, allowing the bank to take proactive measures to mitigate these risks.
Healthcare: Financial Planning and Resource Allocation
In the healthcare industry, financial forecasting is essential for planning budgets, managing resources, and ensuring the sustainability of healthcare services. AI-driven financial forecasting can analyze historical financial data, patient volumes, and healthcare trends to predict future revenue and expenses. This helps healthcare providers plan their budgets, allocate resources, and make informed decisions about investments in facilities, equipment, and staff.
For instance, a hospital can use AI to forecast future patient volumes based on historical data, seasonal trends, and demographic changes. This helps the hospital plan its budget, allocate resources, and ensure that it has the necessary staff and equipment to meet patient needs.
Additionally, AI can predict potential financial risks, such as changes in reimbursement rates or increases in operational costs, allowing the hospital to take proactive measures to manage these risks.
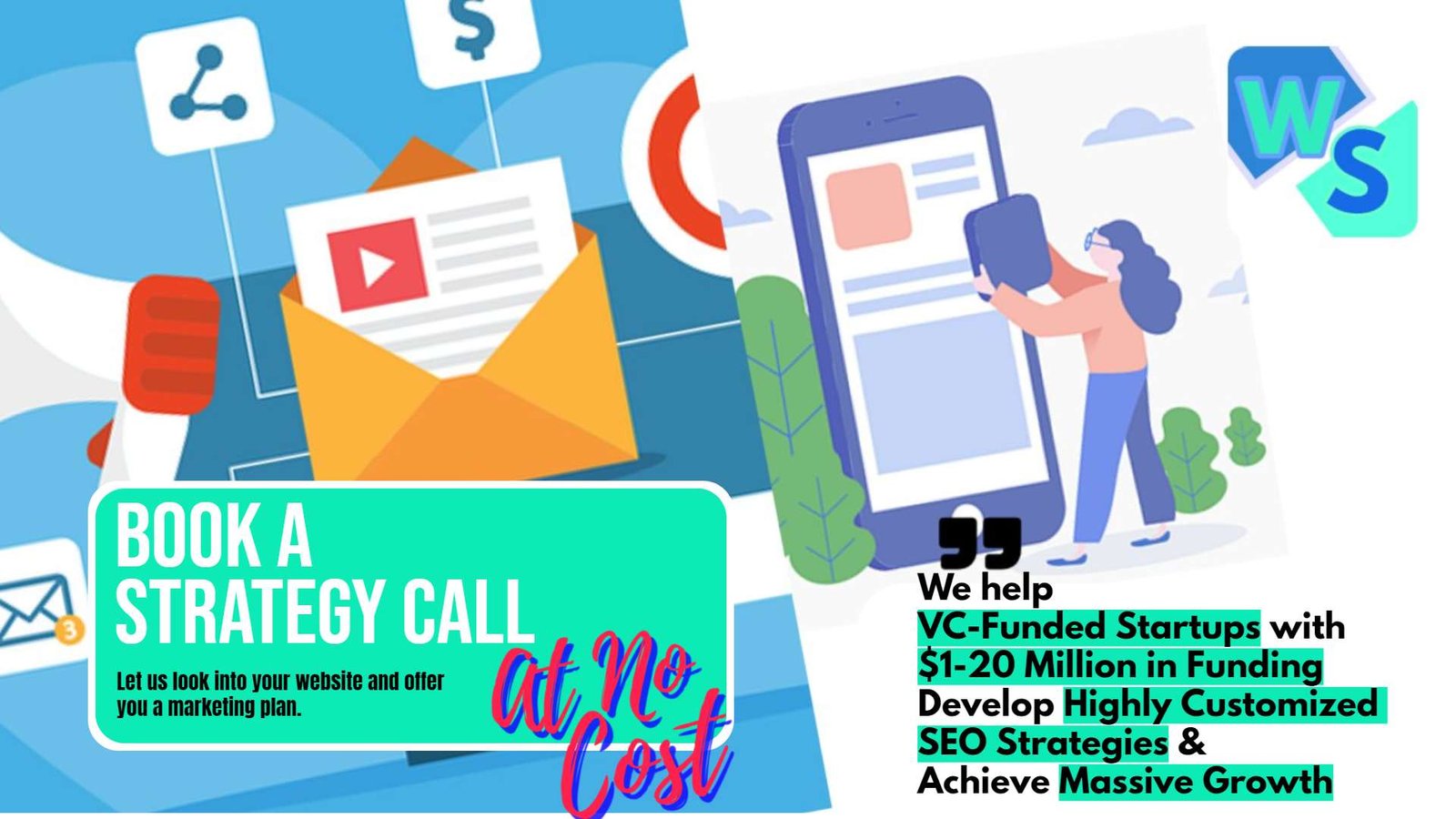
Related: Check out our free tools:
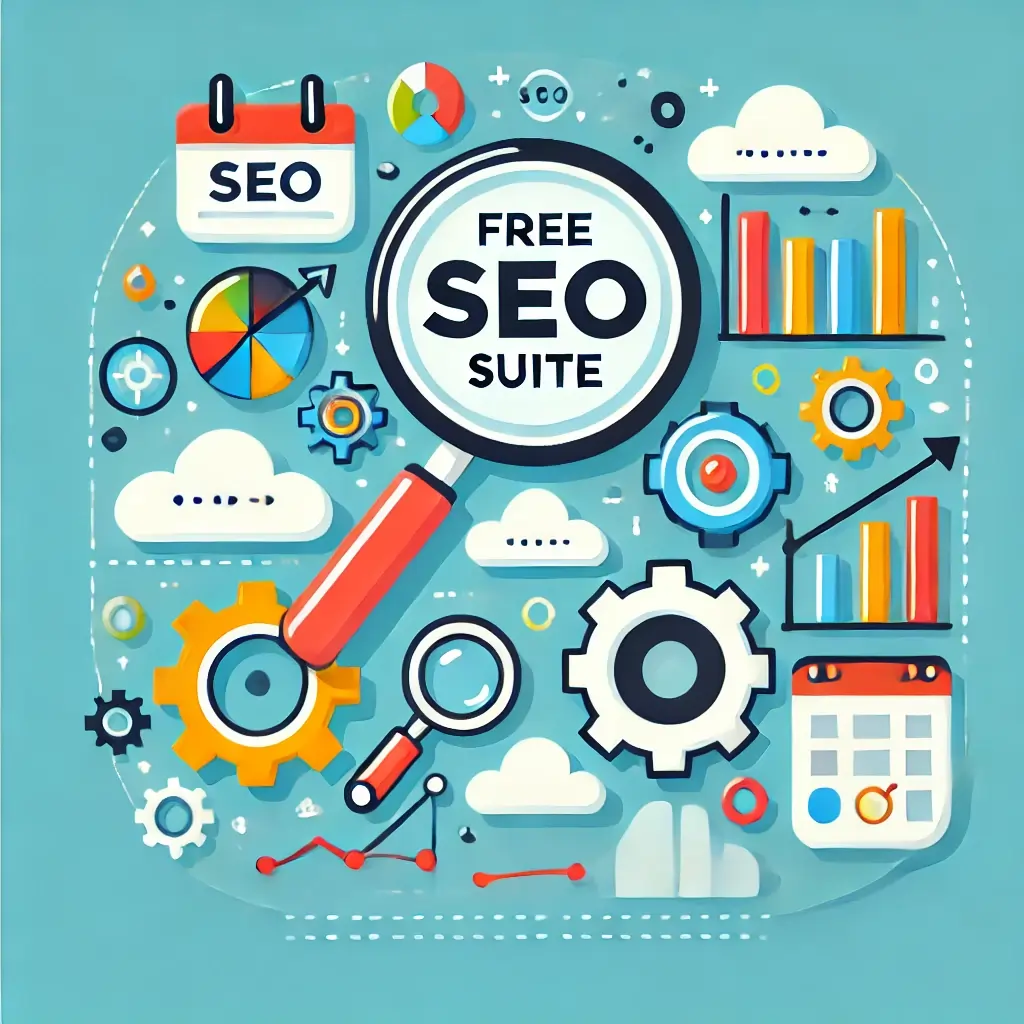
Real Estate: Market Analysis and Investment Planning
In the real estate industry, accurate financial forecasting is essential for market analysis and investment planning. AI-driven financial forecasting can analyze historical market data, economic indicators, and property trends to predict future market conditions. This helps real estate investors make informed decisions about buying, selling, and managing properties.
For example, a real estate investment firm can use AI to forecast future property values based on historical sales data, economic conditions, and market trends. This helps the firm identify investment opportunities, set realistic price targets, and plan its investment strategy more effectively. Additionally, AI can predict potential financial risks, such as market downturns or changes in interest rates, allowing the firm to take proactive measures to manage these risks.
Logistics: Cost and Revenue Forecasting
In the logistics industry, accurate cost and revenue forecasting is essential for managing budgets, optimizing operations, and ensuring profitability. AI-driven financial forecasting can analyze historical financial data, market trends, and operational metrics to predict future costs and revenue. This helps logistics companies plan their budgets, allocate resources, and make informed decisions about investments and pricing strategies.
For instance, a logistics company can use AI to forecast future transportation costs based on historical data, fuel prices, and market conditions. This helps the company set realistic pricing strategies, plan its budget, and manage its resources effectively.
Additionally, AI can predict potential financial risks, such as changes in fuel prices or disruptions in the supply chain, allowing the company to take proactive measures to manage these risks.
Overcoming Challenges in AI-Driven Financial Forecasting
Ensuring Data Quality
The accuracy and effectiveness of AI-driven financial forecasting depend heavily on the quality of the data being analyzed. Poor data quality, such as incomplete or inaccurate data, can lead to incorrect predictions and misguided decisions. Ensuring data quality is essential for building reliable AI models.
Implement a rigorous data governance strategy to ensure data quality. This includes regular data cleaning, validation, and enrichment processes. Establish clear data collection standards and train your team to adhere to these standards consistently. By maintaining high-quality data, you can ensure that your AI-driven predictions are accurate and actionable.
Addressing Bias in AI Models
AI models can sometimes exhibit biases that reflect the biases present in the training data. This can lead to unfair or discriminatory outcomes, particularly in areas such as lending, hiring, and customer segmentation. Addressing bias in AI models is essential for ensuring fairness and ethical use of AI.
Implement bias detection and mitigation techniques during the model development process. This includes analyzing the training data for potential biases, using techniques such as fairness constraints to reduce bias in the models, and regularly auditing the models to ensure they remain fair over time. Involving diverse teams in the development and review process can also help identify and address potential biases.
Managing Model Interpretability
Advanced AI models, such as deep learning neural networks, can be highly accurate but often lack interpretability. This can make it difficult for business users to understand how the models arrive at their predictions and make it challenging to trust and act on the insights.
Focus on developing interpretable models where possible, and use techniques such as LIME (Local Interpretable Model-agnostic Explanations) or SHAP (SHapley Additive exPlanations) to explain complex model predictions. Providing clear, interpretable insights helps build trust and enables business users to make informed decisions based on the AI-generated predictions.
Ensuring Seamless Integration
Integrating AI tools into existing business workflows can be challenging, especially for businesses with established processes and systems. Ensuring seamless integration and minimal disruption is essential for successful adoption.
Work closely with your IT team or a dedicated vendor to ensure smooth integration. Test the integrations thoroughly to make sure data is being accurately captured and analyzed. Develop user-friendly interfaces that allow business users to access and act on AI-generated insights easily. Provide ongoing support and training to help your team adapt to new tools and processes.
Compliance with Regulations
Compliance with regulations is a critical aspect of implementing AI-driven financial forecasting. Businesses must ensure that their AI models comply with relevant regulations and standards, such as GDPR, CCPA, and industry-specific regulations.
Implement robust data privacy and security measures to protect sensitive financial data. Ensure that your AI tools and platforms comply with relevant regulations and have built-in security features. Being transparent with regulators and stakeholders about how your AI models work and how data is used can also help build trust and ensure compliance.
Future Trends in AI-Driven Financial Forecasting
Real-Time Financial Forecasting
As technology advances, real-time financial forecasting will become increasingly important. Businesses will be able to analyze data as it is generated, providing immediate insights and enabling rapid decision-making. This capability is particularly valuable in dynamic environments where timely responses are crucial.
For instance, in finance, real-time forecasting can help identify market trends and trading opportunities as they emerge. In retail, real-time sales forecasting can help manage inventory levels and optimize pricing strategies. By leveraging real-time forecasting, businesses can stay agile and responsive to changing conditions.
Advanced Personalization
Personalization is becoming increasingly sophisticated thanks to AI. The future will see even more advanced levels of personalization, where AI not only tailors marketing messages but also customizes financial forecasts based on individual business needs and preferences.
For example, AI can analyze a company’s financial data, market conditions, and industry trends to create customized financial forecasts that align with its specific goals and challenges. This personalized approach helps businesses make more informed decisions and achieve their financial objectives.
Integration with IoT
The integration of AI with the Internet of Things (IoT) is set to revolutionize financial forecasting. IoT devices generate vast amounts of data that can be analyzed by AI to provide deeper insights into financial performance and operational efficiency.
For example, in manufacturing, IoT sensors can monitor equipment performance in real-time. AI can analyze this data to predict maintenance needs and optimize production schedules. In retail, IoT devices can track inventory levels and customer behavior, providing valuable insights for financial forecasting. By leveraging IoT data, businesses can achieve more accurate and comprehensive financial forecasts.
Ethical AI and Transparency
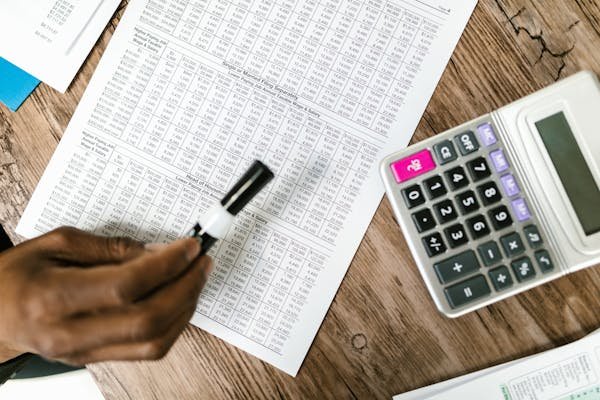
As AI becomes more pervasive, there will be a greater focus on ethical AI and transparency. Consumers and regulators are increasingly concerned about how data is used and the potential biases in AI models. Businesses will need to ensure their AI practices are transparent, fair, and ethical.
Implementing ethical AI involves regular audits of AI models to detect and mitigate biases, ensuring transparency about how data is collected and used, and complying with all relevant regulations. By prioritizing ethical AI, businesses can build trust with customers and stakeholders, which is essential for long-term success.
Predictive Maintenance and Operational Efficiency
Predictive maintenance, driven by AI, is transforming industries by improving operational efficiency and reducing costs. AI analyzes data from machinery and equipment to predict when maintenance is needed, preventing unexpected breakdowns and extending the lifespan of assets.
For instance, in logistics, AI can analyze data from vehicle sensors to predict maintenance needs, schedule repairs proactively, and minimize downtime. This leads to more efficient fleet management, reduced operational costs, and improved service reliability. Businesses adopting predictive maintenance can achieve significant cost savings and operational efficiencies.
Conclusion
AI-driven financial forecasting is a game-changer for businesses looking to navigate the complexities of the modern financial landscape. By leveraging the power of AI, businesses can transform their data into accurate, actionable insights that drive better decision-making, optimize operations, and proactively manage risks.
Understanding the fundamentals of AI in financial forecasting involves recognizing how AI enhances data processing, pattern recognition, and real-time analysis. For startup founders and business leaders, implementing AI-driven financial forecasting requires a strategic approach. This includes assessing data needs, choosing the right AI tools, building and training effective models, and integrating these insights seamlessly into financial processes.
Read Next:
- The Power of Cause Marketing: Key Statistics for 2024
- ROI of Marketing Automation: Key Statistics for 2024
- Marketing to Millennials: Key Statistics for 2024
- B2B Video Marketing: Key Statistics for 2024
- X (Twitter) Marketing: Key Statistics for 2024
Comments are closed.