Text analytics is about making sense of text data, turning words into valuable insights. With the explosion of data from social media, emails, reviews, and other sources, understanding this text has become crucial for businesses. AI technology is revolutionizing how we analyze text, making it faster, more accurate, and incredibly insightful. This article will explore how AI enhances text analytics and provide actionable advice for leveraging this powerful combination to benefit your business.
Understanding Text Analytics
Text analytics is the process of converting unstructured text data into meaningful and actionable insights. For startup founders, mastering text analytics is crucial as it offers the potential to understand customer needs, optimize operations, and drive strategic decisions. Here’s a deeper dive into understanding text analytics and how you can leverage it strategically for your startup.
The Importance of Text Analytics for Startups
In the early stages of a startup, understanding customer feedback and market trends can make or break your business.
Text analytics provides a way to systematically analyze large volumes of text data, which is often rich with insights but difficult to process manually. By utilizing text analytics, startups can identify patterns and trends that inform product development, marketing strategies, and customer service improvements.
Key Components of Text Analytics
Text analytics involves several key components, each playing a vital role in extracting valuable insights from text data. For startup founders, understanding these components helps in effectively implementing and utilizing text analytics.
Data Collection is the first step. It’s essential to gather data from diverse sources such as social media, customer reviews, emails, and surveys. The broader the data source, the richer and more comprehensive the insights.
Data Preprocessing involves cleaning and organizing the collected data. This step includes removing irrelevant information, correcting errors, and converting text into a format suitable for analysis. High-quality data preprocessing ensures that your analytics results are accurate and reliable.
Text Mining is the process of extracting useful information from large volumes of text. This involves techniques like pattern recognition, clustering, and classification. For startups, text mining can uncover hidden patterns and relationships in the data that provide a competitive edge.
Leveraging Text Analytics for Competitive Advantage
For startup founders, leveraging text analytics effectively can provide a significant competitive advantage. Here’s how you can strategically use text analytics in your business.
Understanding Customer Sentiment is one of the most direct applications. Analyzing customer reviews, social media mentions, and feedback forms can help you gauge customer sentiment towards your brand, products, or services.
Positive sentiment indicates what you are doing right, while negative sentiment highlights areas that need improvement. This understanding can inform your customer service strategies and product development processes.
Market Research is another critical area where text analytics can be invaluable. By analyzing industry reports, news articles, and competitor content, you can identify market trends and emerging opportunities. This strategic insight allows you to position your startup more effectively and capitalize on market gaps before your competitors do.
Enhancing Customer Experience involves using text analytics to personalize interactions with your customers. By understanding their preferences and pain points, you can tailor your communications and offerings to meet their specific needs. This personalized approach not only improves customer satisfaction but also fosters loyalty and long-term engagement.
Implementing Text Analytics in Your Startup
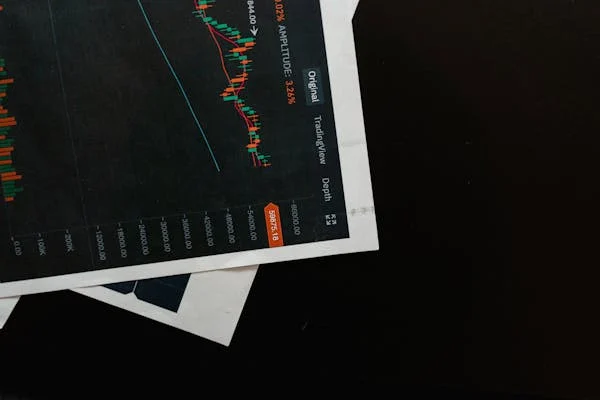
To implement text analytics in your startup, start by defining clear objectives. What specific insights are you looking to gain? Whether it’s improving customer satisfaction, optimizing marketing strategies, or understanding market trends, having clear goals will guide your text analytics efforts.
Choose the Right Tools that fit your budget and technical expertise. There are various text analytics tools available, from open-source solutions to enterprise-grade platforms.
Evaluate them based on their features, ease of use, and scalability. Tools like Python’s Natural Language Toolkit (NLTK) or commercial options like IBM Watson and Google Cloud Natural Language API offer robust text analytics capabilities.
Build a Skilled Team that understands both the technical and business aspects of text analytics. This team will be responsible for data collection, preprocessing, and analysis. Investing in training and development ensures that your team stays updated with the latest techniques and tools in text analytics.
Overcoming Challenges in Text Analytics
Implementing text analytics comes with its challenges. Data Privacy is a significant concern, especially with regulations like GDPR and CCPA. Ensure that your data collection and processing practices comply with relevant regulations to avoid legal issues.
Data Quality is another challenge. Poor quality data can lead to inaccurate insights and misguided decisions. Regularly audit your data sources and preprocessing steps to maintain high data quality.
Scalability is crucial as your startup grows. Ensure that your text analytics infrastructure can handle increasing volumes of data without compromising performance. This might involve investing in more robust hardware or cloud-based solutions that offer scalability.
Measuring the Impact of Text Analytics
For startup founders, it’s important to measure the impact of your text analytics initiatives to ensure they are delivering value. Define Key Performance Indicators (KPIs) that align with your business objectives. These could include customer satisfaction scores, engagement rates, or sales figures.
Regularly Review and Adjust your strategies based on the insights gained from text analytics. Continuous improvement ensures that your startup remains agile and responsive to changing market conditions.
What are the primary challenges in scaling AI-powered text analytics, and how can startups address them?
One of the biggest hurdles in scaling text analytics is managing the volume and quality of unstructured data. Startups can often get ahead of this challenge by implementing robust data cleaning and preprocessing pipelines from the start, which remove noise and standardize text inputs before they hit the model.
Tools like spaCy and NLTK offer libraries that are highly effective for natural language processing and can be easily adapted for scaling purposes. For instance, ensuring that text data is consistently labeled and categorized helps maintain model accuracy as the volume grows.
How can startups ensure scalability in their text analytics initiatives?
For scalability, cloud-based solutions are invaluable, as they offer on-demand computing power and storage without upfront infrastructure costs. Platforms like AWS SageMaker or Google Cloud AI enable startups to train models on smaller datasets initially and scale up as the data volume and processing needs increase.
Additionally, building modular pipelines allows startups to experiment and add components—like named entity recognition or sentiment analysis—without overhauling the entire system. This modularity supports both rapid iteration and long-term growth, which are essential for startups evolving quickly.
What steps can be taken to maintain data privacy in AI-powered text analytics?
Data privacy is crucial, especially with text data, which can contain sensitive information. Startups should implement data anonymization techniques, removing personally identifiable information (PII) before data enters the analytics pipeline. Federated learning is also an emerging solution that enables model training without direct access to user data, thus enhancing privacy.
Additionally, encrypting data both in transit and at rest ensures that information remains secure at all stages of processing. By focusing on these measures, startups can gain users’ trust, which is particularly valuable in a data-centric culture.
How can a data-driven culture support successful scaling of text analytics within a startup?
Creating a data-driven culture means empowering all team members—not just data scientists—with the ability to understand and act on data insights. This begins with providing accessible dashboards and visualizations for non-technical staff, which democratizes data insights across the organization.
Hosting regular workshops on data literacy and encouraging collaborative discussions around text analytics findings also helps align departments around the technology’s value. For example, a startup could conduct monthly briefings where team members share how text analytics insights impacted their goals, creating a feedback loop that reinforces the culture.
For startups, using AI to develop a real, working product or solution is daunting. AI allows us more open lines of thought, and can potentially improve an idea in its infancy. However, that does not turn the idea into a product.
It is crucial to move from a test product to the final version quickly, and to maintain best practices throughout development. Failing to do so results in technical debt, and the longer a product takes, the higher the cost. Initial costs are the barrier between startups and AI.
However, those costs will continue to lower as AI companies scale. AI will be easier to use, and even more accessible for startups and small businesses as the technology evolves. But it is crucial that startups find uses for AI today in order to avoid needing to catch up at a later date. Everyone is trying to find novel uses for AI today in order to develop them tomorrow.
Maintaining data quality is vital for startups. Regular data cleaning processes and clear entry guidelines help ensure consistency. Setting up audits and feedback loops catches errors early and builds a culture of accountability, making AI efforts more reliable and effective.
Scalability hinges on flexible systems. Cloud-based solutions handle growing data demands without heavy infrastructure, and modular architecture allows incremental updates. This approach keeps costs manageable and adapts smoothly as data needs grow.
Embedding encryption and access controls early on is essential. Regular team training and compliance checks reinforce privacy practices, ensuring data stays secure as the company grows and meets regulatory standards.
Building a data-driven culture starts with making data accessible to everyone. Dashboards and regular discussions help the team see how data informs their roles, fostering a collaborative culture that naturally embraces insights for growth.
I would focus on data cleansing and normalization from the start for consistent data quality. Implement automated pipelines that flag anomalies or missing data, ensuring the data used to train models is relevant and accurate. Regular audits of data sources and using pre-trained models as baselines can also help maintain quality as the dataset grows.
To scale effectively, consider a modular architecture that allows you to add or modify components without disrupting the entire system. Cloud-based platforms like AWS, with scalable data storage and processing options, help us handle increased demand flexibly. Optimizing algorithms for batch processing and deploying models at the edge can also keep performance strong as data volumes grow.
Data privacy is critical, so start by implementing clear data governance policies that control access to sensitive information. Anonymize data wherever possible and prioritize tools with built-in encryption. Regular security audits help ensure compliance, and for startups, it’s wise to bake privacy into the product roadmap from the beginning, not as an afterthought.
Building a data-driven culture is about education and accessibility. Encourage all teams—not just data scientists—to understand and use insights by holding regular workshops, sharing success stories, and making data tools user-friendly.
Empowering team members to see the direct impact of their work on company goals strengthens buy-in and ensures analytics is a shared responsibility across the organization.
Balancing these elements can set a strong foundation for scaling AI text analytics, allowing startups to grow without compromising quality or data integrity.
1. Addressing Data Quality Issues
Ensuring high-quality data is crucial for text analytics. We’ve found that implementing strict data cleansing and pre-processing workflows early on helps minimize errors and improves the reliability of the analytics. Consistency in data inputs is key, especially as the scale increases.
2. Ensuring Scalability
To achieve scalability, it’s essential to design with flexibility in mind. We’ve utilized modular architecture that allows us to scale different components independently, adapting to fluctuations in data volume without compromising performance. This modularity also helps manage costs effectively as we grow.
3. Maintaining Data Privacy
Data privacy is a significant concern, especially as we deal with sensitive customer information. We follow stringent data handling protocols and prioritize encryption and anonymization techniques. Staying compliant with data protection regulations like GDPR is fundamental to building trust with our users.
4. Fostering a Data-Driven Culture
Creating a data-driven culture within the organization has been pivotal. Encouraging the team to make decisions based on data insights, and providing training on data literacy, ensures everyone is aligned with the company’s analytical goals. This cultural shift supports scalability by making data central to our operations.
Practical Applications of AI in Text Analytics
AI in text analytics is not just a theoretical concept; it has practical applications that can significantly benefit startups. Understanding and implementing these applications strategically can give your startup a competitive edge and drive growth.
As the Founder and CEO of Zapiy.com, I’ve seen how AI-driven text analytics techniques can be transformative for businesses looking to better understand customer sentiment, enhance support, and refine market research. AI and NLP offer powerful tools that can turn vast amounts of unstructured text data into actionable insights. Here are a few of the most effective methods and their practical applications:
Named Entity Recognition (NER)
Named Entity Recognition is a foundational technique in text analytics. By identifying and categorizing entities—like names, locations, brands, and products—NER provides a clearer picture of what and who is being discussed in customer communications. For instance, when analyzing social media comments, reviews, or support tickets, NER can pinpoint the specific features or products customers frequently mention, enabling businesses to identify which areas are most important to users. NER is particularly useful in customer support, where it can quickly flag brand names or critical issues, allowing support teams to prioritize and respond more effectively.
Practical Application: At Zapiy, NER has been crucial in our AI tools for tracking brand sentiment. By tagging and categorizing mentions of our products, we can immediately identify emerging trends in customer feedback and adjust our marketing or support strategies as needed.
Topic Modeling
Topic modeling techniques, such as Latent Dirichlet Allocation (LDA), are invaluable for understanding the major themes across large bodies of text data. Topic modeling identifies common topics discussed within customer feedback, reviews, or survey responses, revealing underlying patterns that might otherwise go unnoticed. For example, a restaurant chain could use topic modeling to group feedback into categories like “food quality,” “service speed,” and “ambiance.” This breakdown helps managers quickly grasp what matters most to their customers and can guide operational improvements.
Practical Application: In market research, topic modeling allows us to rapidly analyze hundreds of survey responses or reviews, grouping feedback by theme. This helps us streamline the research process, enabling more strategic decisions based on a comprehensive understanding of customer preferences.
Sentiment Analysis
Sentiment analysis is one of the most widely adopted AI-driven techniques for text analytics, providing insights into the emotional tone of customer communications. By identifying sentiment—positive, negative, or neutral—businesses can get a quick sense of how customers feel about their brand, services, or products. More advanced sentiment analysis can even capture nuanced emotions (e.g., frustration vs. disappointment), allowing for more tailored responses in customer support.
Practical Application: In customer support, sentiment analysis helps our team prioritize responses to negative or urgent messages, which can prevent potential churn. It also allows us to keep a pulse on overall customer sentiment trends, so we can take proactive measures when satisfaction dips.
Language Generation
Language generation, often driven by models like GPT, can create human-like responses to text inputs. This technology is a game-changer for customer support, enabling automated, contextually appropriate responses to frequently asked questions. When coupled with sentiment analysis and NER, language generation can tailor replies that reflect the tone and urgency of the customer’s inquiry. It also has applications in content creation for FAQs, knowledge bases, and marketing materials, allowing small teams to expand their reach.
Practical Application: At Zapiy, we use language generation to automate responses to common support queries. It provides immediate assistance to customers for straightforward questions and frees up human agents to handle more complex issues.
Practical Advice for Implementing These Techniques
Start Small and Focused: When implementing these techniques, start with a specific goal. For instance, if customer support is your focus, begin with sentiment analysis and NER to prioritize responses. This minimizes resource strain and allows for measurable results that build the case for further AI investments.
Use Pre-Trained Models and Custom Fine-Tuning: Tools like spaCy, Hugging Face, or open-source models offer powerful pre-trained NLP capabilities. Fine-tuning these models on your data (like support tickets or feedback) allows for more tailored analysis without building an entire model from scratch.
Ensure Data Privacy and Compliance: Customer data must be handled with care, especially when analyzing sensitive feedback or support requests. Encrypt data and anonymize personal information as needed to remain compliant with regulations.
By integrating techniques like NER, topic modeling, sentiment analysis, and language generation, small businesses can better understand and respond to customer needs, gaining insights that inform product development, customer service, and marketing strategies.
With experience from leading roles at Apple and Google, I’m deeply engaged with AI applications in NLP and text analytics. I’d love to share practical insights on the most effective AI techniques for customer sentiment analysis, market research, and more.
In my experience, applying AI techniques like named entity recognition and sentiment analysis can completely shift how we understand and respond to customer feedback. I’ve seen NER bring invaluable structure to what’s often chaotic data—identifying specific brands, competitors, or issues within vast amounts of unstructured text to reveal not only what’s being said but precisely who and what matters.
Combining this with sentiment analysis creates an incredibly layered understanding, especially useful in refining customer support and guiding product decisions. For instance, if customers repeatedly mention a feature alongside positive sentiment, that’s a clear signal to prioritize its development.
Named Entity Recognition (NER) is highly effective for identifying specific entities such as organizations, names, locations, and even product mentions within relatively large datasets.
It can also play a crucial role in customer sentiment analysis since it can automatically detect brand mentions, competitors, or specific product mentions from reviews, social media posts and customer feedback.
For instance, retailers can use NER to identify which products are frequently praised or criticized by consumers. This can help you respond quickly to negative feedback and adjust your product line, increasing your average customer satisfaction rate. Use pre-trained models such as Hugging Face or SpaCy because they are fully optimized for advanced NER tasks.
This will save you a lot of time and resources. Fine-tuning these models on your domain-specific data can help you yield more accurate results.
Streamlining Customer Feedback Analysis
For startups, customer feedback is invaluable. It provides direct insights into how your product or service is perceived in the market. However, manually sifting through feedback can be time-consuming and inefficient. AI-powered text analytics can automate this process.
By using sentiment analysis and topic modeling, you can quickly identify common themes and sentiments in customer feedback. This allows you to address issues promptly and make data-driven decisions to improve your offerings. For example, if multiple customers mention a specific feature as problematic, you can prioritize fixing it in your next update.
Enhancing Content Creation and Optimization
Content marketing is a powerful tool for startups to build brand awareness and engage with their audience. AI in text analytics can help optimize your content strategy. By analyzing the performance of existing content, AI can identify what resonates with your audience and what doesn’t.
This insight can guide your content creation efforts, ensuring that you produce content that meets your audience’s needs and preferences. Additionally, AI can assist in keyword analysis and SEO optimization, helping your content rank higher in search engine results and driving more organic traffic to your site.
Automating Social Media Monitoring
Social media is a vital platform for startups to connect with their audience and build their brand. However, keeping track of every mention, comment, or review can be overwhelming. AI-powered text analytics can automate social media monitoring.
By analyzing social media data in real-time, AI can alert you to trending topics, emerging issues, or viral content related to your brand. This allows you to respond quickly to customer inquiries, manage your online reputation, and capitalize on trending topics to engage with your audience more effectively.
Optimizing Customer Support
Providing excellent customer support is crucial for startups aiming to build a loyal customer base. AI in text analytics can enhance your customer support operations by analyzing support tickets, chat logs, and email interactions.
By identifying common issues and frequently asked questions, AI can help you create a comprehensive knowledge base and automate responses to routine inquiries.
This reduces the workload on your support team and ensures that customers receive quick and accurate responses. Moreover, AI can provide insights into the overall performance of your customer support, helping you identify areas for improvement.
Driving Sales and Lead Generation
For startups, generating leads and driving sales are top priorities. AI-powered text analytics can analyze customer interactions, website behavior, and social media engagement to identify potential leads. By understanding the interests and behaviors of your audience, you can tailor your sales strategies to meet their needs.
Additionally, AI can help segment your audience more effectively, allowing you to create personalized marketing campaigns that drive conversions. This targeted approach ensures that your marketing efforts are more efficient and effective, maximizing your return on investment.
Conducting Competitive Analysis
Understanding your competitors is crucial for staying ahead in the market. AI in text analytics can automate and enhance your competitive analysis efforts. By analyzing public data such as news articles, social media posts, and online reviews, AI can provide insights into your competitors’ strategies, strengths, and weaknesses.
This information can inform your business strategy, helping you identify opportunities to differentiate your startup and capitalize on market gaps. For instance, if AI analysis reveals a common customer complaint about a competitor’s product, you can highlight how your product addresses that issue in your marketing campaigns.
Improving Recruitment and Talent Management
Hiring the right talent is critical for the success of any startup. AI-powered text analytics can streamline the recruitment process by analyzing resumes, cover letters, and other application materials. By identifying key skills and experiences, AI can help you shortlist the most suitable candidates more efficiently.
Additionally, text analytics can be used to analyze employee feedback and performance reviews, providing insights into workplace culture and identifying areas for improvement. This data-driven approach ensures that you attract and retain top talent, fostering a productive and positive work environment.
Enhancing Legal and Compliance Monitoring
Startups often operate in highly regulated industries where compliance is critical. AI in text analytics can assist in monitoring legal and regulatory documents, ensuring that your startup adheres to relevant laws and regulations.
By analyzing contracts, policies, and other legal texts, AI can identify potential compliance issues and alert you to any discrepancies. This proactive approach minimizes the risk of legal complications and ensures that your startup remains compliant with industry standards.
Facilitating Research and Development
Innovation is the lifeblood of startups, and AI-powered text analytics can significantly enhance your research and development efforts. By analyzing academic papers, patents, and industry reports, AI can provide insights into the latest trends and advancements in your field.
This information can guide your R&D initiatives, helping you stay at the forefront of innovation. Additionally, text analytics can assist in identifying potential collaborators or partners, expanding your network and fostering collaborative innovation.
The Role of Natural Language Processing (NLP)
Natural Language Processing (NLP) is at the heart of AI-powered text analytics, enabling machines to interpret, analyze, and generate human language. For startup founders, mastering NLP can unlock numerous opportunities to enhance business operations, improve customer interactions, and drive innovation. Here’s a deeper exploration of NLP’s role and how you can strategically leverage it for your startup’s success.
Advanced Natural Language Processing (NLP) techniques such as topic modelling and sentiment analysis allow startup businesses to convert raw customer feedback into highly structured, valuable insights for product/service improvement.
AI models such as GPT and BERT categorize customer feedback as “positive” or “negative.” These models can capture customer emotions, detect emerging pain points and identify sentiment patterns that may not be obvious to the human eye.
This allows you to prioritize product improvements, enhance user experience and tailor your customer support based on real feedback. You can be 100% sure that whatever change you implement, no matter how small it may seem, will result in improved customer satisfaction.
Leveraging sentiment analysis to adjust your internal process can result in an improved customer satisfaction rate, which has a direct impact on customer retention.
How can advanced NLP techniques help startups analyze customer feedback effectively?
NLP allows startups to process and interpret customer feedback at scale, transforming qualitative data into actionable insights. With tools like sentiment analysis and topic modeling, companies can automatically identify common issues, sentiments, and emerging trends within customer reviews and support tickets.
For instance, a startup can use NLP to categorize feedback into positive, neutral, or negative sentiment, which provides a snapshot of customer satisfaction while highlighting specific areas for improvement. By incorporating NLP into their feedback analysis, startups can react in real time, adjusting products or services to align with customer needs, ultimately strengthening retention and loyalty.
In what ways can NLP facilitate automated content creation?
NLP-driven tools like GPT-based text generation and summarization algorithms allow startups to automate content creation, enabling them to generate product descriptions, social media posts, and blog summaries with minimal manual effort. Startups in fast-paced industries can especially benefit, as NLP can help scale content without diluting brand voice or quality.
For example, a real estate startup might use NLP to draft property descriptions based on listing data, freeing up time for their team to focus on higher-level strategy. Additionally, AI-powered content creation can help maintain consistent messaging, ensuring that the brand’s tone resonates across multiple channels.
How does NLP enhance the ability to monitor social media trends?
NLP enables startups to analyze large volumes of social media data, helping them keep up with trends, customer sentiment, and competitor activity. Through named entity recognition and hashtag tracking, companies can track mentions of their brand, products, or relevant topics in real time.
A startup, for instance, could use NLP to track customer responses to a new product launch, quickly spotting potential issues or positive feedback that can inform their marketing and support efforts. By automating social listening through NLP, businesses can stay agile, responding to market changes as they unfold.
At Pearl Lemon, we’ve seen firsthand how NLP enhances our ability to analyze customer feedback by identifying sentiment, trends, and specific pain points. NLP-driven tools streamline this process, allowing us to make quick, data-backed adjustments to our strategy.
For instance, sentiment analysis has helped us respond to customer needs more effectively, allowing us to spot recurring issues and tailor solutions accordingly.
For content creation, NLP can automate summaries, keyword extraction, and topic generation, helping us produce targeted, high-quality content quickly.
Additionally, using NLP for social media monitoring has been transformative, enabling real-time tracking of brand mentions and emerging trends so we can engage with our audience more proactively.
Advanced Techniques in NLP
Beyond basic tokenization and sentiment analysis, NLP encompasses a variety of advanced techniques that can provide deeper insights and more sophisticated text analysis. Techniques such as named entity recognition, topic modeling, and language generation can offer significant advantages when applied strategically.
Named Entity Recognition (NER) involves identifying and classifying entities such as names, dates, and locations within text. For startups, NER can be used to automate information extraction from large documents, such as identifying key details from contracts or extracting important information from customer reviews. This not only saves time but also ensures accuracy in handling critical data.
Topic Modeling is another advanced NLP technique that identifies the main themes or topics within a body of text. By using algorithms like Latent Dirichlet Allocation (LDA), startups can analyze large volumes of text data to uncover underlying themes and trends.
This is particularly useful for market research, as it allows you to understand what topics are most relevant to your audience and adjust your content and product strategies accordingly.
Language Generation, powered by models such as GPT-4, enables machines to generate human-like text based on given inputs. This can be leveraged for creating personalized marketing messages, automating content creation, or even developing chatbots that provide a more natural and engaging customer experience. For startups, this means being able to scale communication efforts without compromising on personalization and quality.
Strategic Implementation of NLP
To effectively implement NLP in your startup, it’s important to adopt a strategic approach that aligns with your business goals. Here are some actionable steps to get you started:
Invest in the Right Infrastructure. Ensure that you have the necessary computational resources and infrastructure to support NLP initiatives. Cloud-based solutions such as AWS, Google Cloud, and Microsoft Azure offer scalable options that can grow with your business.
Focus on Data Quality. High-quality data is crucial for the success of NLP applications. Invest time in cleaning and preprocessing your text data to remove noise and ensure accuracy. This will improve the performance of your NLP models and lead to more reliable insights.
Build or Source Skilled Talent. NLP requires a combination of linguistic and technical expertise. Hire or train team members who have a strong background in both areas. Consider partnering with academic institutions or hiring consultants to fill any gaps in expertise.
Develop Custom Models. While there are many pre-built NLP models available, developing custom models tailored to your specific needs can provide a competitive advantage. Train models on your own data to improve accuracy and relevance. Continuously update these models with new data to keep them relevant.
Enhancing Customer Interaction with NLP
NLP can transform how startups interact with their customers, providing more personalized and efficient service. Implementing NLP in customer service can automate routine inquiries and free up human agents to handle more complex issues. For instance, chatbots powered by NLP can understand and respond to customer queries in a natural and conversational manner, improving the overall customer experience.
Moreover, analyzing customer interactions using NLP can provide insights into customer needs and preferences. This information can be used to tailor products and services to better meet customer expectations, leading to higher satisfaction and loyalty. By understanding the language and sentiment of your customers, you can create more targeted and effective marketing campaigns that resonate with your audience.
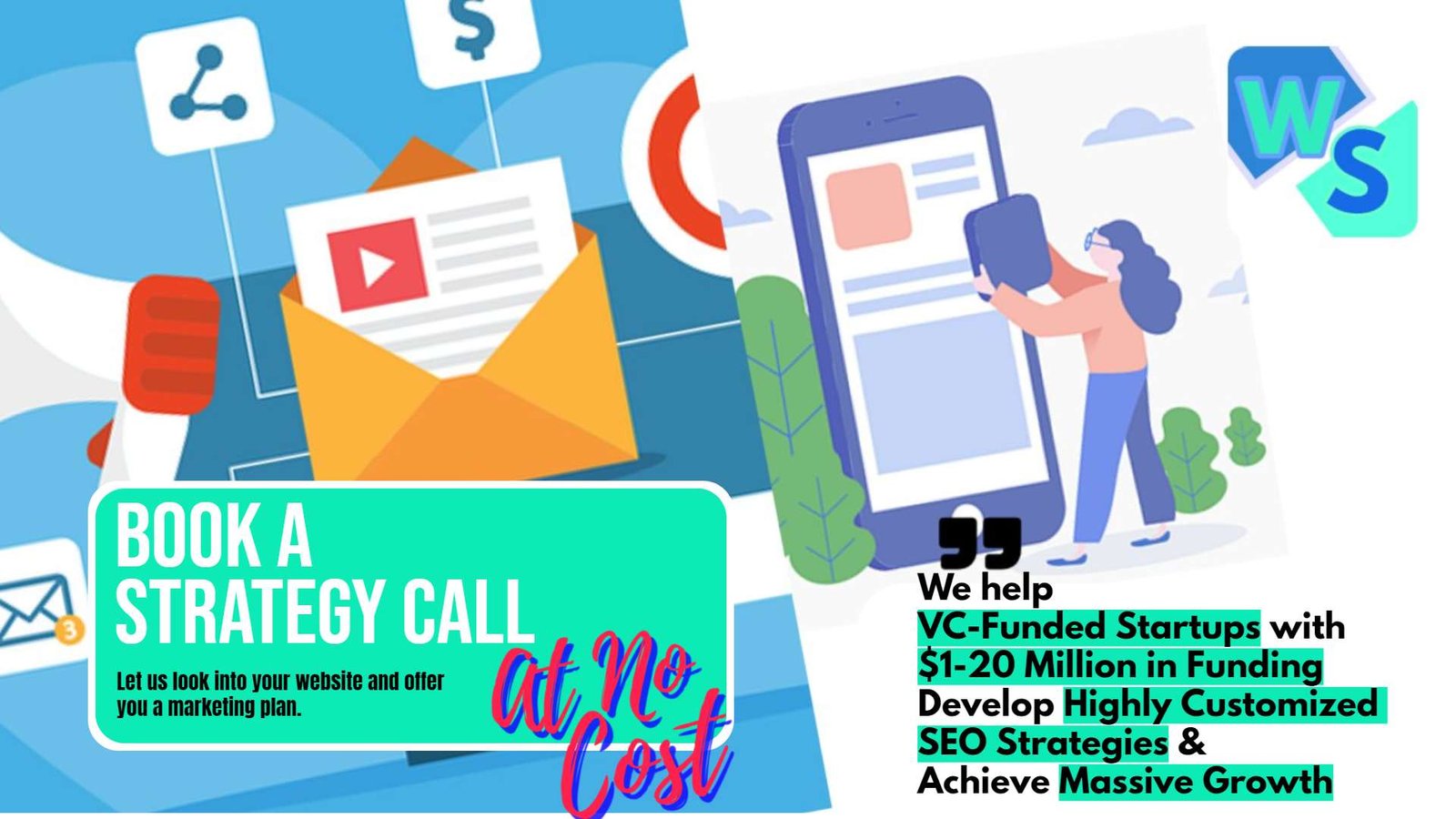
Related: Check out our free tools:
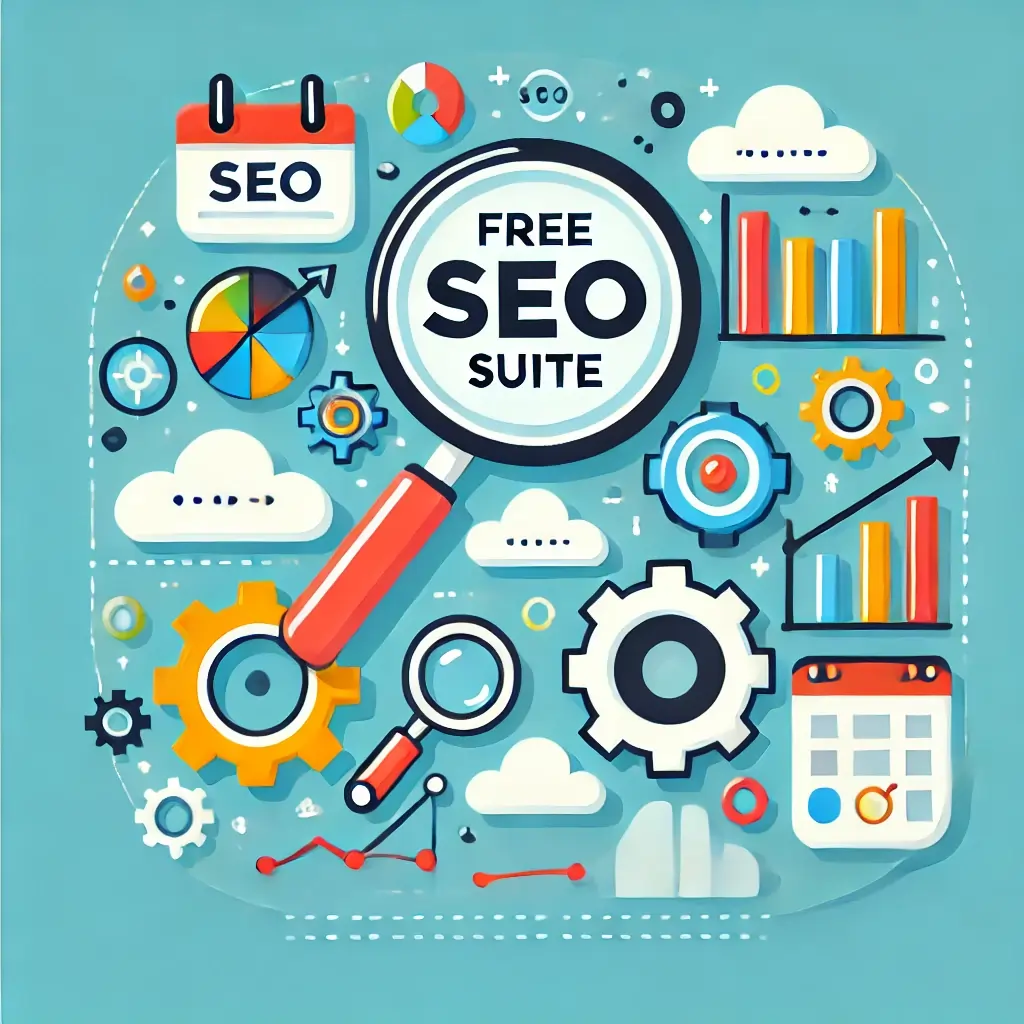
Leveraging NLP for Competitive Analysis
NLP can also be a powerful tool for competitive analysis. By analyzing publicly available text data such as news articles, press releases, and social media posts, startups can gain insights into competitors’ strategies and market positioning. This information can inform your own business strategies, helping you identify opportunities and threats in the market.
For example, NLP can be used to track mentions of competitors and analyze the sentiment around these mentions. Understanding how customers perceive your competitors can highlight areas where you can differentiate your offerings and capture market share. Additionally, monitoring industry trends and emerging topics can help you stay ahead of the curve and anticipate market shifts.
Driving Innovation with NLP
Innovation is essential for the growth and success of any startup, and NLP can play a key role in driving innovation. By analyzing large volumes of text data, NLP can uncover new insights and ideas that can fuel innovation. For instance, analyzing academic papers, patents, and industry reports using NLP can help identify emerging technologies and trends that are relevant to your business.
Furthermore, NLP can facilitate collaboration and knowledge sharing within your organization. By automating the analysis of internal documents, emails, and meeting notes, NLP can help ensure that valuable information is not overlooked and that insights are shared across teams. This can lead to more informed decision-making and foster a culture of continuous improvement and innovation.
Measuring the Impact of NLP
To ensure that your NLP initiatives are delivering value, it’s important to measure their impact. Define clear metrics that align with your business objectives, such as customer satisfaction scores, response times, or revenue growth. Regularly review these metrics to assess the effectiveness of your NLP applications and make data-driven adjustments as needed.
Additionally, gather feedback from your team and customers to understand how NLP is impacting their experience. Use this feedback to identify areas for improvement and to refine your NLP strategies. By continuously monitoring and optimizing your NLP efforts, you can ensure that they remain aligned with your business goals and continue to deliver value.
Implementing AI-Powered Text Analytics in Your Business
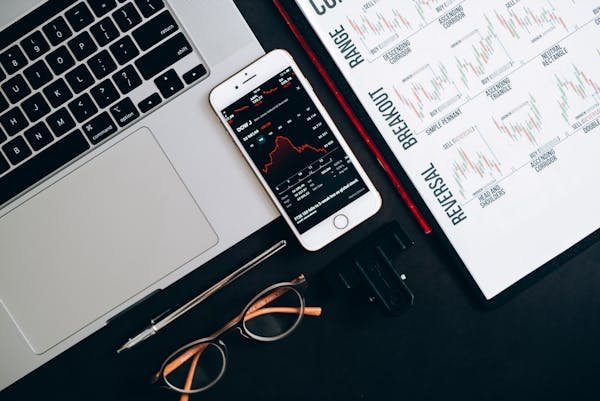
Implementing AI-powered text analytics can transform your startup, offering insights that drive growth, enhance customer satisfaction, and streamline operations. However, successful implementation requires a strategic approach. Here’s how startup founders can effectively integrate AI-powered text analytics into their business, ensuring maximum impact and value.
At JoggAI, we’ve fine-tuned a practical, adaptable approach to data handling, which enables us to scale and innovate without sacrificing data quality or compliance.
I’d be glad to share insights on how startups can lay a strong foundation for successful AI-driven text analytics, helping them extract meaningful, actionable insights from their data right from the start.
For any startup diving into AI-powered text analytics, I’d suggest beginning with a commitment to agility—both in technology choice and operational approach. Instead of jumping into rigid frameworks or tools, explore flexible APIs or modular platforms that let you scale as your data grows. For me, quality in this space comes down to having clean, well-labeled data that’s primed for analysis. It’s worth setting up lightweight automation for data cleaning and labeling early on, which will allow your team to act on insights faster without getting bogged down in data noise.
Also, don’t underestimate the power of proactive data governance: establish clear privacy and compliance guidelines that can scale with your ambitions. This structured, adaptable approach allows you to harness AI’s potential fully, even in a lean startup environment.
For startups diving into AI-driven text analytics, the foundation begins with data quality. While it’s tempting to jump right into tool selection, ensuring high-quality, relevant data upfront is where success truly starts. Poor data quality leads to skewed insights, wasting both time and resources.
One effective approach is starting with small, representative datasets, refining them to ensure they’re clean and accurately labeled, and gradually scaling from there. It’s surprising how much clarity you gain on your tool requirements when your data is already in good shape.
A useful tip: schedule regular data audits to maintain quality as your datasets grow. Clean, consistent data is the cornerstone of reliable AI-driven analytics, supporting your insights without compromising accuracy.
AI powered text analytics must be strategized and monitored with privacy and compliance in mind every step of the way.
If an organization is scraping the internet for data, and either isn’t maintaining the privacy of the data, or is compromising the security of personal data, this can turn into a regulatory nightmare.
First and foremost, keep all of your text analytics practices private and secure. Only after that can we focus on scaling, and data quality.
Choosing Scalable Tools: For startups, selecting the right AI-powered text analytics platform is crucial. I recommend considering tools that offer flexibility and scalability as your company grows, such as cloud-based platforms with pre-built models for sentiment analysis, text classification, and language processing.
Startups should evaluate solutions from well-established providers like AWS and Google Cloud AI for robust infrastructure and reliable support.
Ensuring Data Quality: The effectiveness of AI-driven analytics heavily relies on clean, high-quality data. I suggest startups invest in robust data preprocessing methods, such as text normalization and data labeling, to ensure that the machine learning models yield accurate and actionable insights. Consistent monitoring and updating of the data pipeline are key to maintaining high data integrity.
Overcoming Data Privacy and Compliance Challenges: With AI’s growing use in text analytics, data privacy is a critical concern, especially when dealing with sensitive information.
Startups should prioritize tools and practices that adhere to GDPR, CCPA, and other privacy regulations. Implementing data anonymization techniques and encryption will help mitigate risks and ensure compliance with privacy laws.
I am the CEO/Founder of Petoi, a programmable bionic robot pet shop for adults and kids with applications in robotics education, STEM, coding, AI learning, and human companionship.
An eclectic maker, photographer, and poet with a rich background in academia, I earned my PhD in Physics and Master’s in Computer Science from Wake Forest University, after completing my BS in Physics at Nanjing University.
Start by defining clear use cases for AI-powered text analytics, like customer feedback or content categorization, ensuring alignment with business goals.
Experts stress the importance of clean, structured data and selecting the right AI tools that integrate with existing systems. A phased approach with small pilots helps refine the process and ensures scalability, driving adoption and impact.
Establish Clear Objectives
The first step in implementing AI-powered text analytics is to establish clear, measurable objectives. Understand what you aim to achieve with text analytics.
Whether it’s improving customer support, enhancing marketing efforts, or optimizing product development, having specific goals will guide your strategy and help measure success. Clear objectives also help in selecting the right tools and methodologies, ensuring that your efforts are focused and aligned with your business needs.
Invest in Scalable Infrastructure
As your startup grows, so will your data needs. Investing in scalable infrastructure is crucial to support AI-powered text analytics. Cloud-based solutions offer flexibility and scalability, allowing you to handle increasing volumes of data without significant upfront costs.
Platforms like AWS, Google Cloud, and Microsoft Azure provide robust AI and machine learning tools that can be scaled according to your requirements. Ensuring your infrastructure can grow with your business prevents bottlenecks and maintains efficiency.
Develop a Comprehensive Data Strategy
A comprehensive data strategy is essential for effective text analytics. This involves identifying the data sources that are most relevant to your objectives and ensuring you have the mechanisms in place to collect, store, and manage this data.
Consider integrating data from various channels such as social media, customer reviews, emails, and internal documents. A unified data repository can streamline the analysis process and provide a holistic view of your operations and customer interactions.
Focus on Data Quality and Integrity
High-quality data is the cornerstone of reliable text analytics. Ensure that the data you collect is accurate, clean, and relevant. This involves regular data audits to remove duplicates, correct errors, and fill gaps.
Implementing strict data governance policies can help maintain data quality and integrity, ensuring that the insights generated are trustworthy and actionable. Additionally, using advanced data preprocessing techniques can enhance the quality of your data, leading to more precise analytics outcomes.
Leverage Custom AI Models
While pre-built AI models offer a good starting point, developing custom models tailored to your specific needs can provide a significant competitive advantage. Custom models can be trained on your unique datasets, improving their accuracy and relevance.
This tailored approach ensures that the insights generated are highly specific to your business context. Invest in skilled data scientists and machine learning engineers who can develop and maintain these models, ensuring they evolve with your business needs.
Foster a Data-Driven Culture
Implementing AI-powered text analytics is not just about technology; it’s also about fostering a data-driven culture within your organization. Encourage your team to rely on data for decision-making and to continuously seek insights from text analytics.
Provide training and resources to help employees understand and utilize AI tools effectively. A culture that values data-driven insights will enhance the overall impact of your text analytics initiatives, driving better decisions and more innovative solutions.
Integrate Text Analytics with Business Processes
To maximize the impact of AI-powered text analytics, integrate it seamlessly with your existing business processes. For example, use text analytics insights to inform your marketing strategies, product development, and customer service operations.
Create workflows that automatically feed analytics results into decision-making processes, ensuring that insights are acted upon promptly. This integration ensures that text analytics is not an isolated activity but a core component of your business strategy.
On behalf of Deepak Shukla, CEO of Pearl Lemon, I’m glad to share how AI-driven text analytics has transformed our business operations and those of our clients.
One impactful use case we’ve implemented is AI-powered sentiment analysis to improve customer satisfaction. By analyzing customer feedback in real time, we quickly identify recurring issues and address them proactively. This approach has directly led to a noticeable improvement in customer retention and satisfaction by making our responses more targeted and timely.
Additionally, AI-driven competitive analysis has been invaluable. With automated text analytics, we monitor competitor mentions and trends across social media and industry publications, gaining insights that inform our strategic decisions.
This has increased our operational efficiency, allowing us to stay ahead without extensive manual tracking.
In my company, we implemented a specific AI-based sentiment analysis tool (MonkeyLearn) to better understand client feedback across emails and social media interactions. This tool helped us identify subtle patterns that weren’t immediately obvious (like the fact that clients were frustrated with response times during specific types of inquiries).
With this knowledge, we improved our communication workflows and made our responses faster and more personalized. As a result, we saw an 18% increase in client satisfaction. What’s more important, our repeat business grew by about 15% within just a few months.
In simple terms, AI provided a clear roadmap for our strategic shifts. It helped me understand ‘client sentiment’ in real-time and based on this, I adapted our approach quickly. That’s how I saw measurable results.
Honestly, I believe the same approach can be applied across various industries to help businesses communicate more effectively and see tangible improvements in client relationships and even sales growth.
Monitor Performance and Iterate
Continuous monitoring and iteration are crucial for the success of AI-powered text analytics. Regularly review the performance of your analytics models and the impact of the insights generated. Use key performance indicators (KPIs) aligned with your objectives to measure success.
Gather feedback from stakeholders and make necessary adjustments to improve accuracy and relevance. This iterative approach ensures that your text analytics efforts remain aligned with your business goals and continue to deliver value over time.
Ensure Ethical and Compliant Use of Data
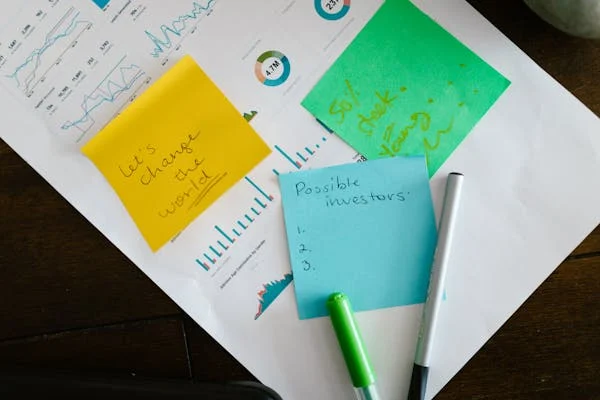
Ethical considerations and compliance with data protection regulations are paramount in implementing AI-powered text analytics. Ensure that your data collection and analysis practices adhere to relevant laws such as GDPR or CCPA.
Implement robust data security measures to protect sensitive information and maintain customer trust. Being transparent about how you use data and ensuring ethical practices can enhance your reputation and prevent legal issues.
Explore New Opportunities and Innovations
AI-powered text analytics is a rapidly evolving field with continuous advancements and new opportunities. Stay informed about the latest developments and explore innovative applications that can benefit your startup.
For instance, new NLP models, improved sentiment analysis techniques, or advancements in real-time text processing can offer fresh opportunities for enhancing your analytics capabilities. Being proactive in exploring and adopting new technologies can keep your startup at the forefront of innovation.
Collaborate and Share Knowledge
Collaboration and knowledge sharing are essential for the successful implementation of AI-powered text analytics. Engage with industry peers, participate in forums, and attend conferences to learn from others’ experiences and share your insights.
Collaboration can lead to new ideas, partnerships, and innovations that enhance your text analytics efforts. Additionally, fostering a collaborative environment within your organization encourages creativity and collective problem-solving, driving better results.
Conclusion
Enhancing text analytics with AI technology offers startups a powerful toolset to transform raw data into actionable insights. By understanding the strategic value of text analytics and leveraging AI’s capabilities, startup founders can drive significant improvements across various aspects of their business.
From establishing clear objectives and investing in scalable infrastructure to fostering a data-driven culture and ensuring ethical data practices, the journey to effective AI-powered text analytics requires careful planning and execution.
Implementing advanced NLP techniques, developing custom AI models, and integrating insights seamlessly into business processes are crucial steps in harnessing the full potential of AI in text analytics.
Read Next:
- Best AI Writing Tools for Bloggers
- Efficient Content Creation with AI Assistance
- AI-Powered Content Creation for Marketers
- How AI Transforms Data Analytics: A Deep Dive
- How to Leverage AI for Content Ideas
Comments are closed.