Understanding how much value a customer brings to your business over their entire relationship with you is crucial. This metric, known as Customer Lifetime Value (CLV), helps businesses make smarter decisions about marketing, sales, and customer service. With AI analytics, calculating CLV becomes more accurate and insightful, allowing companies to tailor strategies that enhance customer retention and maximize profits. In this article, we’ll explore how AI analytics can revolutionize the way you calculate and leverage Customer Lifetime Value.
What is Customer Lifetime Value?
Understanding the Core Concept
Customer Lifetime Value (CLV) is more than just a number; it’s a strategic metric that represents the total revenue a customer is expected to generate for a business over the entirety of their relationship. This includes all purchases, recurring revenues, and any additional value they bring through referrals and word-of-mouth.
For startup founders, understanding CLV is crucial as it provides a comprehensive view of a customer’s financial contribution, allowing for better strategic planning and resource allocation.
Calculating CLV: Basic Formula
At its simplest, CLV can be calculated using the formula:
CLV=Average Purchase Value×Purchase Frequency×Customer LifespanCLV = \text{Average Purchase Value} \times \text{Purchase Frequency} \times \text{Customer Lifespan}CLV=Average Purchase Value×Purchase Frequency×Customer Lifespan
This basic formula provides a starting point. However, for a more accurate and detailed calculation, especially with the help of AI analytics, additional factors such as gross margin, discount rates, and retention costs should be included. These elements offer a deeper insight into the profitability of each customer, considering both revenues and costs associated with retaining them.
Why CLV Matters for Startups
For startups, every dollar counts. Understanding and maximizing CLV can be a game-changer in several ways. Firstly, it helps in identifying the most valuable customer segments, allowing you to tailor your marketing and sales efforts towards acquiring and retaining these high-value customers. This targeted approach ensures a higher return on investment (ROI) for your marketing spend.
Secondly, CLV aids in financial forecasting and budgeting. By predicting future revenues from existing customers, startups can make more informed decisions about scaling operations, investing in new products, or expanding into new markets. This forward-looking approach reduces financial risks and supports sustainable growth.
Thirdly, CLV highlights the importance of customer retention. It’s generally more cost-effective to retain existing customers than to acquire new ones. By focusing on improving customer satisfaction and loyalty, startups can enhance their CLV, leading to more stable and predictable revenue streams.
Strategic Actions Based on CLV Insights
Startup founders can leverage CLV insights to drive several strategic actions. One effective approach is to create tailored retention programs for high-value customers. This might include personalized communication, exclusive offers, or loyalty rewards. Such initiatives not only improve retention rates but also increase customer satisfaction and advocacy.
Another strategic action is to refine your customer acquisition strategies. By analyzing the CLV of different customer segments, startups can identify which channels and tactics are most effective in attracting high-value customers. This enables more efficient allocation of marketing budgets and resources, ensuring that efforts are focused on the most profitable segments.
Additionally, understanding CLV allows startups to optimize their pricing strategies. If the CLV of a particular segment is high, it might justify premium pricing or upselling opportunities. Conversely, for segments with lower CLV, competitive pricing or volume discounts might be more effective. This nuanced approach to pricing ensures that your offerings are aligned with the value each customer segment brings to your business.
Incorporating AI for Enhanced CLV Calculation
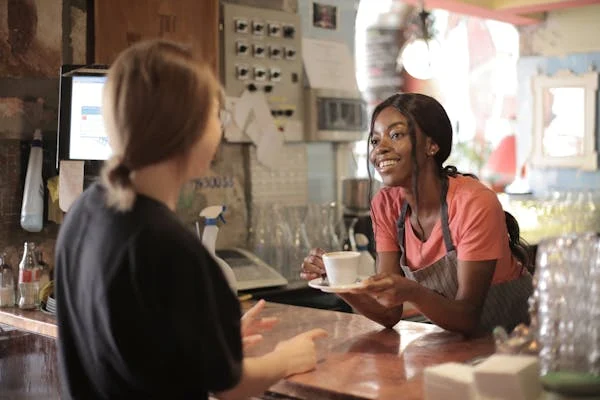
AI analytics can significantly enhance the accuracy and depth of CLV calculations. Traditional methods often rely on historical data and simple averages, which may not capture the complexities of customer behavior. AI, on the other hand, can analyze vast datasets, identify patterns, and make predictions with high precision.
Machine learning algorithms can incorporate various data points such as transaction history, customer interactions, and demographic information to provide a more holistic view of CLV. They can also adjust predictions based on real-time data, ensuring that your CLV estimates are always current and accurate.
Furthermore, AI can segment customers based on their predicted CLV, allowing for more granular targeting. This means that marketing and sales efforts can be precisely tailored to the needs and preferences of different customer groups, maximizing the impact of your initiatives.
Challenges and Considerations
While CLV is a powerful metric, it comes with challenges that startups need to address. One common challenge is data quality. Inaccurate or incomplete data can lead to incorrect CLV calculations and misguided strategies. Therefore, startups must invest in robust data management practices to ensure the reliability of their data.
Another consideration is the dynamic nature of CLV. Customer behavior and market conditions can change, impacting the accuracy of CLV predictions. Continuous monitoring and updating of your CLV models are essential to maintain their relevance and usefulness.
Moreover, ethical considerations should not be overlooked. Using customer data responsibly and transparently is crucial to maintaining trust and complying with data protection regulations. Startups should establish clear policies on data usage and ensure that their AI models are free from biases that could lead to unfair or discriminatory outcomes.
Long-Term Benefits of a CLV-Focused Approach
Adopting a CLV-focused approach offers long-term benefits beyond immediate financial gains. It fosters a customer-centric culture within the organization, emphasizing the importance of building and maintaining strong customer relationships. This cultural shift can enhance overall customer experience, leading to higher satisfaction and loyalty.
Moreover, a deep understanding of CLV can inform strategic decisions across the organization. From product development and pricing to marketing and customer service, insights derived from CLV can guide every aspect of your business, ensuring that your strategies are aligned with customer value and business objectives.
How AI Enhances CLV Calculation
Leveraging Machine Learning for Predictive Accuracy
AI, particularly machine learning, significantly enhances the accuracy of CLV calculations by learning from vast datasets. Traditional methods often rely on historical averages and simplistic formulas, which can overlook nuanced patterns in customer behavior.
Machine learning algorithms, however, can analyze thousands of variables simultaneously, identifying trends and correlations that human analysts might miss. For startup founders, this means more precise predictions of customer behavior and value.
Implementing machine learning models allows startups to predict not only how much a customer will spend but also when they will make their next purchase, how frequently they will buy, and how long they will stay engaged with the brand.
This level of detail helps businesses tailor their marketing and customer retention strategies more effectively. For instance, by identifying customers who show signs of declining engagement, startups can proactively reach out with personalized offers or content to re-engage them.
Real-Time Data Processing
One of the most significant advantages of AI is its ability to process and analyze data in real time. This is particularly valuable for startups operating in fast-paced markets where customer behavior can change rapidly. Real-time data processing ensures that CLV calculations are always based on the most current information, making them more relevant and actionable.
For example, if a customer makes a large purchase, AI can immediately update their CLV and trigger targeted follow-up actions such as thank-you emails, personalized recommendations, or loyalty rewards. This immediate response not only enhances the customer experience but also increases the likelihood of future purchases.
Personalized Customer Insights
AI-driven CLV calculations provide personalized insights that go beyond generic customer segments. By analyzing individual customer behaviors, preferences, and interactions, AI can create highly detailed customer profiles. These profiles allow startups to deliver personalized marketing messages, product recommendations, and customer service experiences.
For example, if AI identifies a high-value customer who frequently purchases a particular type of product, the business can tailor its marketing efforts to suggest similar or complementary products. This personalized approach increases the relevance of marketing messages, enhancing customer satisfaction and loyalty.
Identifying High-Value Customers Early
Another strategic benefit of AI in CLV calculation is the ability to identify high-value customers early in their lifecycle. Traditional methods might take months or years to recognize a customer’s potential value, but AI can analyze early behavior patterns to predict future value quickly. This allows startups to allocate resources more efficiently, investing in high-potential customers from the start.
For instance, if a new customer shows buying patterns similar to existing high-value customers, the business can prioritize them for special treatment, such as exclusive offers or early access to new products. This early identification helps build strong relationships with valuable customers, increasing their lifetime value.
Automating Routine Tasks
AI can automate many routine tasks associated with CLV calculation and customer management. This includes data collection, cleaning, analysis, and reporting. Automation reduces the risk of human error, saves time, and ensures that the calculations are consistently accurate and up-to-date.
For startup founders, this means more time to focus on strategic initiatives rather than getting bogged down in data management. Automated systems can continuously monitor customer behavior, update CLV predictions, and generate actionable insights without manual intervention.
Enhancing Cross-Selling and Upselling Strategies
AI can enhance cross-selling and upselling strategies by identifying products that customers are most likely to purchase based on their behavior and preferences. By integrating CLV insights, startups can prioritize recommendations for high-value customers, increasing the chances of additional sales.
For example, if AI analysis shows that customers who buy a certain product often purchase a related item within a few months, the business can create targeted marketing campaigns that suggest the complementary product shortly after the initial purchase. This strategy not only boosts sales but also enhances the overall customer experience by providing relevant and timely suggestions.
Improving Customer Segmentation
AI-driven CLV calculation improves customer segmentation by creating more granular and dynamic segments based on predicted value and behavior.
Traditional segmentation methods often use static criteria such as demographics or purchase history, which can quickly become outdated. AI, however, continuously updates segments based on real-time data, ensuring that marketing efforts are always targeted and effective.
For instance, AI can segment customers not only by their current value but also by their potential to become high-value customers. This allows startups to tailor their marketing and retention efforts more precisely, focusing resources where they will have the greatest impact.
Enhancing Retention Strategies with Predictive Analytics
AI’s predictive capabilities are particularly valuable for enhancing customer retention strategies. By analyzing patterns in customer behavior, AI can predict when customers are likely to churn and identify the factors that contribute to their decision.
This insight allows businesses to intervene before customers leave, implementing targeted retention efforts such as personalized offers, improved customer service, or loyalty rewards.
For example, if AI identifies that a customer’s engagement with your product is declining, it can trigger a retention campaign that includes personalized content, discounts, or check-in messages to re-engage the customer. By addressing potential issues proactively, startups can reduce churn rates and enhance customer loyalty.
Facilitating Strategic Decision-Making
AI-driven CLV calculations provide a solid foundation for strategic decision-making. By offering detailed insights into customer value and behavior, AI enables startup founders to make informed decisions about marketing, sales, product development, and customer service. This data-driven approach ensures that resources are allocated efficiently and strategies are aligned with business goals.
For instance, understanding the lifetime value of different customer segments can inform decisions about which markets to enter, which products to develop, and which marketing channels to prioritize. This strategic use of CLV insights helps startups optimize their operations and drive sustainable growth.
Integrating AI into Business Systems
For AI-driven CLV calculations to be most effective, they must be integrated into existing business systems. This includes CRM platforms, marketing automation tools, and customer service software. Integration ensures that AI insights are accessible across the organization and can be used to inform actions in real-time.
For startup founders, this means working closely with IT and data teams to ensure seamless integration and data flow. Investing in platforms that support easy integration and provide robust API capabilities can facilitate this process, making it easier to harness the full potential of AI-driven CLV insights.
Continuous Improvement and Adaptation
The dynamic nature of customer behavior and market conditions means that AI models for CLV calculation must be continuously updated and refined. Startup founders should establish a process for regularly reviewing model performance, incorporating new data, and making necessary adjustments. This iterative approach ensures that the models remain accurate and relevant, providing reliable insights over time.
Continuous improvement also involves staying updated with advancements in AI technology and methodologies. By keeping abreast of the latest developments, startups can leverage cutting-edge tools and techniques to enhance their CLV calculations and maintain a competitive edge.
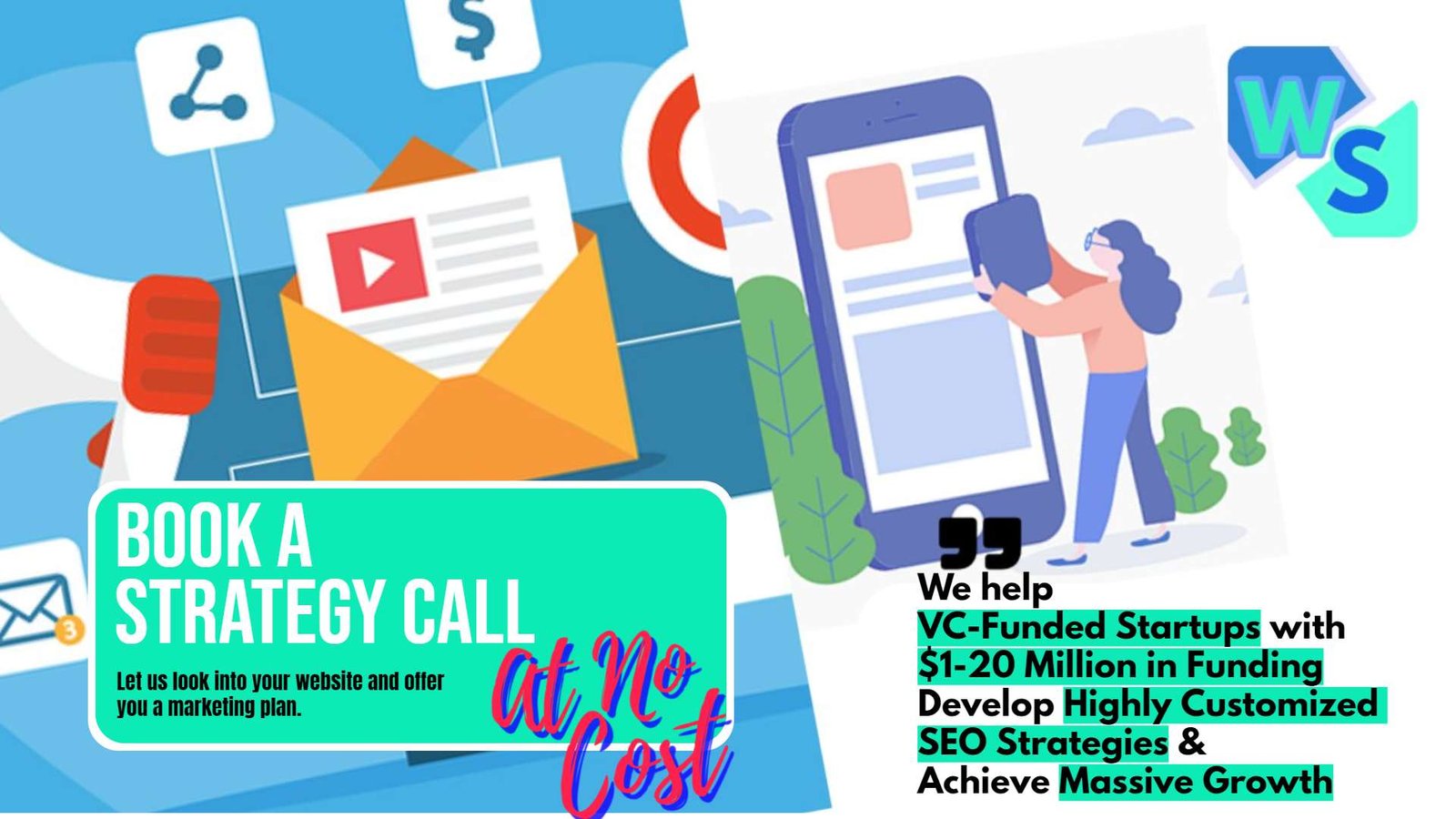
Related: Check out our free tools:
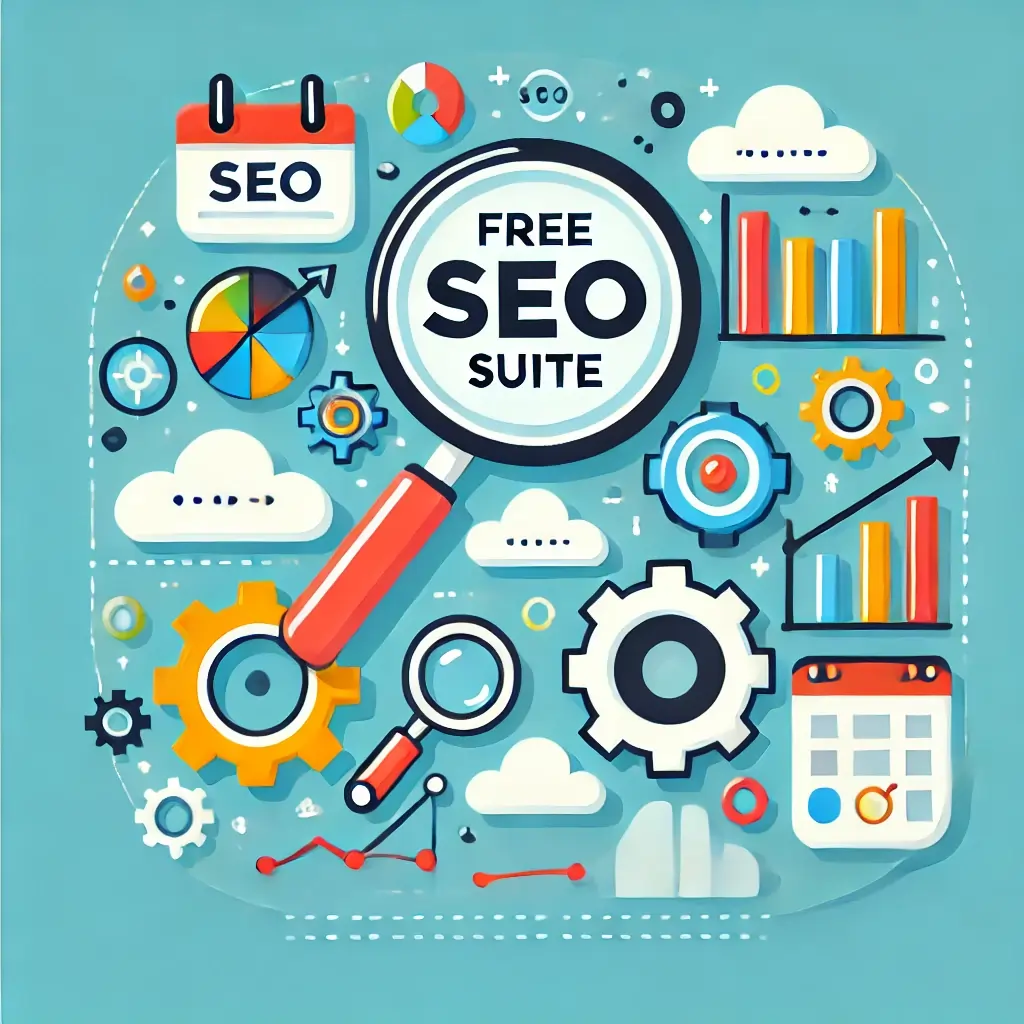
Steps to Calculate CLV with AI Analytics
Establish Clear Objectives and KPIs
Before diving into the technical aspects of calculating CLV with AI analytics, it’s crucial to establish clear objectives and key performance indicators (KPIs). As a startup founder, you need to define what you aim to achieve with your CLV calculations.
Are you looking to enhance customer retention, optimize marketing spend, or identify high-value customer segments? Establishing clear goals will guide your AI implementation and ensure that your efforts are aligned with your broader business strategy.
Once your objectives are set, identify the KPIs that will help you measure success. These might include metrics such as customer acquisition cost (CAC), average purchase value, purchase frequency, and customer retention rate. Monitoring these KPIs will provide a clear picture of how well your CLV strategies are working and where adjustments might be needed.
Data Collection and Integration
The foundation of accurate CLV calculation is high-quality data. Collect data from various sources, including transaction histories, customer interactions, website behavior, and social media activity. This comprehensive dataset will enable your AI models to analyze customer behavior from multiple angles.
Integration of data is equally important. Ensure that your data sources are interconnected and can be accessed seamlessly by your AI tools. This might involve integrating your CRM, e-commerce platform, marketing automation tools, and customer service software. By creating a unified data ecosystem, you can ensure that your AI models have the complete picture needed for precise CLV calculations.
Data Cleaning and Preparation
Raw data often contains errors, duplicates, and inconsistencies that can skew your AI-driven CLV calculations. Data cleaning and preparation are critical steps to ensure the accuracy of your analysis. This process involves removing duplicate records, correcting inaccuracies, filling in missing values, and standardizing formats.
Invest in tools that automate data cleaning and preparation tasks. Platforms like Alteryx and TIBCO Data Science offer robust data preparation features that can save time and reduce the risk of errors. By ensuring that your data is clean and well-prepared, you lay a solid foundation for accurate and reliable CLV calculations.
Feature Engineering
Feature engineering is the process of selecting and transforming variables to improve the performance of your AI models. Identify the key features that influence CLV, such as purchase frequency, average order value, customer engagement metrics, and product preferences. Transform these features into a format that can be effectively utilized by your machine learning algorithms.
For example, you might create new features such as the average time between purchases, the total number of interactions with customer service, or the recency of the last purchase. These engineered features can provide deeper insights into customer behavior and enhance the predictive power of your AI models.
Selecting and Training AI Models
Choosing the right AI models is crucial for accurate CLV calculation. Different models have different strengths, so consider using a combination of machine learning algorithms to capture various aspects of customer behavior. Commonly used models for CLV prediction include regression analysis, decision trees, random forests, and neural networks.
Train your models using historical data to identify patterns and trends. Use a portion of your data for training and reserve a separate dataset for validation. This approach ensures that your models are robust and can generalize well to new data.
Platforms like DataRobot and Google Cloud AI offer automated machine learning capabilities that simplify model selection and training, making it accessible even for startups with limited data science expertise.
Model Validation and Testing
Model validation is a critical step to ensure that your AI-driven CLV predictions are accurate and reliable. Validate your models using the reserved dataset and evaluate their performance based on metrics such as mean absolute error (MAE), root mean squared error (RMSE), and R-squared. These metrics provide insights into how well your models are predicting actual customer behavior.
Perform cross-validation by dividing your dataset into multiple folds and training/testing the model on different subsets. This helps in assessing the model’s robustness and prevents overfitting. If your models do not perform as expected, iterate by refining your feature engineering process, adjusting model parameters, or incorporating additional data.
Implementation and Integration
Once your models are validated, integrate them into your business processes. This involves deploying the models into your existing systems and ensuring that they can generate real-time predictions.
Integration with your CRM and marketing automation platforms will enable you to use CLV insights for targeted marketing campaigns, personalized customer interactions, and strategic decision-making.
For instance, your CRM can use CLV predictions to prioritize high-value customers for personalized outreach, while your marketing automation tool can segment audiences based on predicted value and tailor campaigns accordingly. Real-time integration ensures that your strategies are always based on the latest insights, enhancing their effectiveness.
Continuous Monitoring and Optimization
AI-driven CLV calculation is not a one-time task; it requires continuous monitoring and optimization. Regularly track the performance of your models and update them with new data to maintain their accuracy. Monitor key metrics such as customer retention rates, average order value, and customer engagement to assess the impact of your CLV strategies.
Establish a feedback loop where insights from your CLV models inform your business strategies, and the outcomes of these strategies feed back into your models. This iterative process ensures that your AI-driven CLV calculations remain relevant and effective in a dynamic business environment.
Leveraging Insights for Strategic Decisions
The ultimate goal of calculating CLV with AI analytics is to leverage insights for strategic decisions. Use your CLV predictions to guide investments in marketing, customer service, and product development. For example, if your models indicate that certain customer segments have high lifetime value, allocate more resources to acquiring and retaining these customers.
Additionally, use CLV insights to inform product development by identifying features or products that resonate most with high-value customers. This ensures that your offerings align with customer preferences, enhancing satisfaction and loyalty. By making data-driven decisions, you can optimize your business strategies and drive sustainable growth.
Communicate Findings Across the Organization
For CLV insights to be effective, they need to be communicated across the organization. Share your findings with relevant teams, including marketing, sales, customer service, and finance. Use visualizations and reports to make the insights accessible and actionable.
Encourage a data-driven culture where teams use CLV insights to inform their strategies and actions. Regular meetings and workshops can facilitate knowledge sharing and ensure that everyone is aligned with the business’s goals and objectives. By fostering a collaborative environment, you can maximize the impact of your AI-driven CLV calculations.
Practical Applications of AI-Driven CLV Insights
Tailoring Marketing Campaigns
Using AI-driven CLV insights, businesses can design marketing campaigns that target the most valuable customer segments. For instance, if your AI model identifies a group of customers with a high predicted CLV, you can create personalized offers and promotions specifically for them.
This increases the likelihood of repeat purchases and enhances customer loyalty. Conversely, for customers with lower CLV, you might implement cost-effective marketing strategies that maintain engagement without overspending.
Optimizing Customer Retention
Retention strategies can be significantly improved using AI insights. By understanding which customers are most likely to churn and their potential value, businesses can prioritize retention efforts on high-value customers.
AI can help identify the factors that lead to customer churn and suggest proactive measures to retain those customers, such as personalized communication, loyalty programs, or special offers. This targeted approach not only improves retention rates but also maximizes the return on investment for retention initiatives.
Enhancing Customer Service
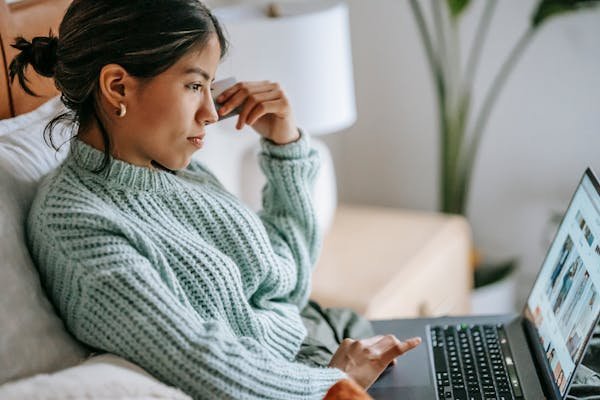
AI-driven CLV calculations can inform customer service strategies by highlighting the needs and preferences of high-value customers. Businesses can allocate more resources to provide exceptional service to these customers, ensuring their issues are resolved quickly and satisfactorily.
For instance, high-value customers might receive priority support or dedicated account managers. This level of personalized service can significantly boost customer satisfaction and loyalty, leading to higher lifetime value.
Strategic Product Development
Understanding CLV through AI analytics can also guide product development. By analyzing the purchasing behavior and preferences of high-value customers, businesses can identify which products or features are most appealing to this segment.
This insight can inform product development decisions, ensuring that new products or enhancements are aligned with customer desires. Additionally, predictive analytics can forecast future trends and demands, helping businesses stay ahead of the market and continuously meet customer needs.
Improving Sales Strategies
Sales teams can leverage AI-driven CLV insights to tailor their approaches. By identifying high-value prospects, sales efforts can be focused on customers who are more likely to contribute significantly to revenue.
Sales teams can use personalized pitches and tailor their interactions based on the predicted value of each customer. This targeted approach not only increases conversion rates but also ensures that sales resources are used efficiently.
Financial Planning and Budgeting
Accurate CLV calculations are essential for effective financial planning and budgeting. By predicting the revenue that high-value customers will generate over their lifetime, businesses can make more informed financial decisions.
This includes setting realistic revenue targets, planning for future investments, and allocating budgets to different departments based on their impact on CLV. Financial strategies can be aligned with customer value, ensuring that the business remains financially healthy and sustainable.
Conclusion
Calculating Customer Lifetime Value (CLV) with AI analytics is a powerful approach that can transform the way startups understand and engage with their customers. By leveraging AI’s capabilities, startups can achieve greater precision and insight, enabling them to make more informed and strategic decisions.
The process involves setting clear objectives, collecting and preparing high-quality data, selecting and training the right AI models, and continuously monitoring and optimizing these models for accuracy.
Read Next:
- Catchy Name Ideas for Your Digital Marketing Company
- Marketing Strategies to Grow Your Boutique
- Top Marketing Strategies for Realtors
- Effective Marketing Strategies for Staffing Agencies
- How Dentists Can Leverage Social Media Marketing
Comments are closed.