This Article has been revised, edited and added to, by Poulomi Chakraborty.
- The Basics of A/B Testing
- Setting Up Your First A/B Test
- Real-Life Example: Headline Testing
- Advanced A/B Testing Strategies
- Overcoming Common Challenges in A/B Testing
- Understanding A/B Testing Metrics
- Advanced A/B Testing Techniques
- Implementing A/B Testing Tools
- Choosing the Right A/B Testing Tool
- Integrating A/B Testing Tools with Your Platforms
- Setting Up Your First Test with an A/B Testing Tool
- Monitoring and Managing Your Test
- Analyzing Test Results with A/B Testing Tools
- Implementing the Winning Variation
- Scaling A/B Testing Efforts
- Leveraging Data from Multiple Tests
- Ensuring Long-Term Success with A/B Testing
- The Future of A/B Testing
- Conclusion
In the ever-evolving world of digital marketing, staying ahead of the competition requires constant innovation and adaptation. One of the most powerful tools at a marketer’s disposal is A/B testing. This technique allows you to compare two versions of a piece of content to determine which one performs better. It’s like having a crystal ball that shows you what your audience truly prefers. Whether you’re tweaking headlines, images, or call-to-action buttons, A/B testing provides the data-driven insights needed to make informed decisions and drive better results.
A/B testing might sound technical and complex, but it doesn’t have to be. With a little guidance, anyone can harness its power to enhance their content strategy. In this comprehensive guide, we’ll explore the ins and outs of A/B testing, from understanding its importance to implementing effective strategies that can significantly boost your content’s performance. So, let’s dive in and unlock the secrets to optimizing your content through A/B testing.
The Basics of A/B Testing
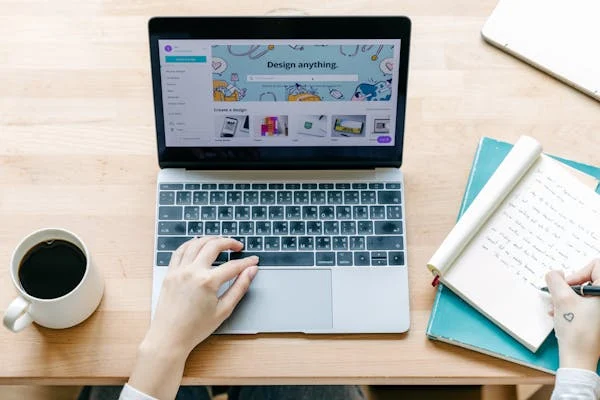
What is A/B Testing?
At its core, A/B testing is a method of comparing two versions of a webpage, email, or other content to see which one performs better. The two versions, referred to as the “A” version and the “B” version, are shown to different segments of your audience simultaneously. By measuring how each version performs based on key metrics, such as click-through rates or conversion rates, you can identify which version is more effective.
Why is A/B Testing Important?
A/B testing is crucial because it removes the guesswork from your content strategy. Instead of relying on assumptions or gut feelings about what might work, you get concrete data that shows what your audience responds to. This evidence-based approach leads to more informed decisions and, ultimately, better results. Whether you’re looking to increase website traffic, boost engagement, or drive more conversions, A/B testing provides the insights needed to achieve your goals.
How Does A/B Testing Work?
The process of A/B testing involves several key steps:
- Identify the Goal: Determine what you want to achieve with your test. This could be anything from increasing click-through rates to improving user engagement.
- Create Variations: Develop two versions of the content you want to test. Ensure that each version differs by only one element, such as a headline, image, or call-to-action button. This allows you to isolate the impact of that specific element.
- Divide Your Audience: Split your audience into two groups, with one group seeing version A and the other group seeing version B. Ensure that the groups are comparable in size and characteristics to get accurate results.
- Run the Test: Launch the A/B test and let it run for a sufficient period to gather enough data. The duration of the test will depend on your traffic and the significance of the results you need.
- Analyze the Results: Compare the performance of both versions based on the metrics you defined in step one. Determine which version performed better and why.
- Implement the Winning Version: Once you have a clear winner, implement the successful version and consider iterating with further tests to continue optimizing your content.
Setting Up Your First A/B Test
Choosing What to Test
When starting with A/B testing, it’s essential to choose elements that can significantly impact your content’s performance. Common elements to test include:
- Headlines: The headline is often the first thing your audience sees. Testing different headlines can reveal which one captures more attention and drives more clicks.
- Images: Visual elements play a crucial role in engaging your audience. Experimenting with different images can help you find the ones that resonate most with your viewers.
- Call-to-Action (CTA): The CTA is a critical component of any marketing content. Testing different wording, colors, and placements can show which version leads to more conversions.
- Content Layout: The way your content is structured and presented can influence user engagement. Testing different layouts can help you find the most effective way to present your information.
- Email Subject Lines: For email marketing, the subject line is vital. Testing different subject lines can reveal which ones lead to higher open rates.
Setting Clear Objectives
Before you start your A/B test, it’s important to define clear objectives. What do you hope to achieve with this test? Setting specific, measurable goals will help you determine the success of your test. For example, if you’re testing headlines, your objective might be to increase click-through rates by 10%.
Creating Hypotheses
A good A/B test starts with a hypothesis. This is an educated guess about what you think will happen and why. For example, you might hypothesize that changing the color of your CTA button from red to green will increase conversions because green is often associated with action and approval.
Implementing the Test
Once you’ve defined your goals and created your hypothesis, it’s time to implement the test. Use A/B testing tools that integrate with your website or email platform to make the process smoother. These tools can help you set up the test, track results, and analyze the data.
Ensuring Statistical Significance
One of the biggest mistakes in A/B testing is ending the test too early. To ensure that your results are reliable, you need to achieve statistical significance. This means that the results are unlikely to be due to chance. Most A/B testing tools will provide a significance score to help you determine when your test has reached this point.
Analyzing and Acting on Results
After running your test for a sufficient period, analyze the results. Look at the key metrics and determine which version performed better. But don’t stop there – dig deeper to understand why one version outperformed the other. Use these insights to inform future tests and continue optimizing your content.
Real-Life Example: Headline Testing
To illustrate the power of A/B testing, let’s look at a real-life example of headline testing. Suppose you run an online store, and you want to increase the click-through rate on your homepage banner. You decide to test two different headlines: “Shop Our Summer Sale” (version A) and “Get 50% Off All Summer Items” (version B).
Setting Up the Test
You split your audience into two groups. Group A sees the first headline, and Group B sees the second. Both groups are exposed to the test for two weeks, ensuring a sufficient amount of data is collected.
Analyzing the Results
After the test period, you analyze the results. You find that version B has a 25% higher click-through rate compared to version A. This suggests that the more specific, offer-focused headline resonates better with your audience.
Implementing Changes
Based on these findings, you decide to implement version B as the permanent headline for your summer sale. Additionally, you use the insights gained to inform future headline tests, focusing on specificity and clear offers.
Advanced A/B Testing Strategies
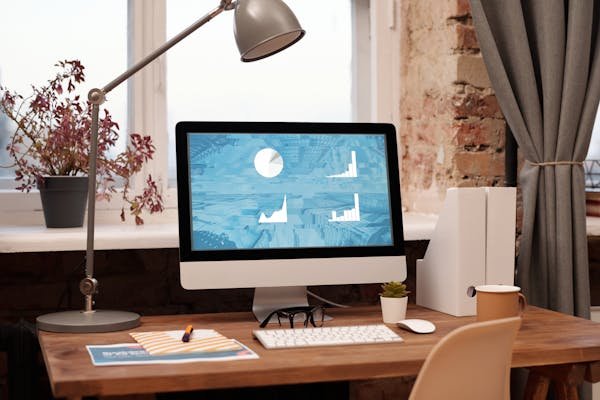
Multi-Variable Testing
Once you’re comfortable with basic A/B testing, you can move on to multi-variable testing. This involves testing multiple elements simultaneously to see how they interact. For example, you might test different combinations of headlines, images, and CTAs. While more complex, this approach can provide deeper insights into how different elements of your content work together.
Sequential Testing
Sequential testing is another advanced strategy. Instead of testing two versions simultaneously, you test them one after the other. This approach is useful when dealing with seasonal content or promotions. By testing version A during one time period and version B during another, you can see how timing affects performance.
Personalization
Personalization takes A/B testing to the next level by tailoring content to different segments of your audience. Instead of a one-size-fits-all approach, you create different versions of your content for different audience groups. This can lead to even better results, as the content is more relevant to each group.
Continuous Testing
A/B testing isn’t a one-time activity. To stay ahead of the competition, you need to adopt a mindset of continuous improvement. Regularly test different elements of your content to keep optimizing and refining your strategy. This ongoing process ensures that your content remains effective and relevant.
Using AI and Machine Learning
Incorporating AI and machine learning into your A/B testing can provide even deeper insights and more efficient testing. These technologies can help you analyze large amounts of data quickly and identify patterns that might not be immediately obvious. They can also automate parts of the testing process, allowing you to focus on strategy and implementation.
Testing Across Channels
Don’t limit your A/B testing to just one channel. Test your content across different platforms, such as your website, email, social media, and ads. This cross-channel approach ensures that your overall marketing strategy is optimized, not just individual components.
Overcoming Common Challenges in A/B Testing
Dealing with Low Traffic
One of the biggest challenges in A/B testing is having enough traffic to achieve statistical significance. If your website or email list has low traffic, it can take a long time to get reliable results. To overcome this, focus on high-impact elements that are more likely to show a significant difference. Alternatively, consider using a multi-armed bandit approach, which can yield quicker results.
Avoiding Biased Results
Bias can creep into A/B testing in many ways, from how you divide your audience to how you interpret the data. To minimize bias, ensure that your audience is split randomly and that you’re using objective metrics to measure success. Additionally, be aware of confirmation bias – the tendency to favor results that confirm your preconceptions.
Ensuring Test Validity
For an A/B test to be valid, it must be properly set up and executed. This includes ensuring that both versions are shown to comparable audience groups and that external factors (like seasonal changes or marketing campaigns) don’t skew the results. Double-check your test setup before launching to avoid invalid results.
Handling Negative Results
Not all A/B tests will yield positive results. Sometimes, neither version performs better, or the changes you test have a negative impact. This is a natural part of the testing process. Use negative results as learning opportunities to refine your hypotheses and improve future tests.
Understanding A/B Testing Metrics
Importance of Metrics in A/B Testing
In A/B testing, metrics serve as the backbone of your experiments. They provide quantifiable data that shows how each version of your content performs. Without the right metrics, your A/B tests can’t provide the actionable insights needed to optimize your content effectively. Metrics allow you to measure user engagement, conversion rates, and other key performance indicators that are essential for making informed decisions.
Key Metrics to Track
When conducting A/B tests, it’s crucial to focus on the right metrics to ensure that your experiments yield valuable insights. Some of the most important metrics to track include click-through rates (CTR), conversion rates, bounce rates, time on page, and engagement metrics like social shares and comments.
Click-through rates measure how often users click on a specific element, such as a headline or a CTA. Conversion rates track the percentage of users who complete a desired action, like making a purchase or filling out a form.
Bounce rates indicate the percentage of users who leave your site after viewing only one page, while time on page measures how long users spend on your content. Engagement metrics, such as social shares and comments, provide insights into how your content resonates with your audience.
Setting Up Goals and KPIs
Before launching an A/B test, it’s essential to establish clear goals and key performance indicators (KPIs). Goals define what you hope to achieve with your test, while KPIs are the specific metrics you’ll use to measure success.
For example, if your goal is to increase email sign-ups, your KPIs might include the conversion rate of your sign-up form and the overall number of new subscribers. Setting clear goals and KPIs ensures that your A/B tests are focused and aligned with your broader marketing objectives.
Interpreting Test Results
Once your A/B test has run for a sufficient period and you’ve gathered enough data, it’s time to analyze the results. Look at the performance of each version based on your predefined metrics. For instance, if you’re testing headlines, compare the click-through rates of each version.
If one version significantly outperforms the other, it’s a strong indicator that the winning version is more effective. However, it’s also important to consider other factors that might influence the results, such as external events or changes in your audience’s behavior. Take a holistic approach to interpreting the data, looking for patterns and insights that can inform future tests.
Statistical Significance and Confidence Levels
In A/B testing, statistical significance indicates that the results of your test are likely not due to chance. Achieving statistical significance means you can confidently conclude that one version is better than the other.
To determine statistical significance, you’ll need to calculate the p-value, which indicates the probability that the observed difference is due to chance. A p-value of less than 0.05 is typically considered statistically significant.
Confidence levels represent the degree of certainty in your results. A 95% confidence level means you can be 95% certain that your results are reliable. Ensuring statistical significance and high confidence levels helps validate your A/B testing results and supports data-driven decision-making.
Common Pitfalls in Metrics Interpretation
Misinterpreting metrics is a common pitfall in A/B testing. One mistake is focusing on vanity metrics that don’t directly impact your goals, such as the number of page views without considering conversion rates.
Another common error is ending tests prematurely before achieving statistical significance, leading to unreliable results. Additionally, it’s crucial to avoid confirmation bias, where you interpret the data to confirm your pre-existing beliefs.
To mitigate these risks, focus on relevant metrics, run tests for a sufficient duration, and maintain an objective approach to data analysis.
Leveraging Insights for Continuous Improvement
The insights gained from A/B testing are invaluable for continuous improvement. Use the data to refine your content strategy, making informed adjustments based on what works best for your audience.
For instance, if a particular headline significantly boosts click-through rates, consider applying similar principles to other parts of your content. Continuously testing and iterating allows you to stay agile and responsive to changing audience preferences and market trends.
By leveraging the insights from A/B testing, you can create a cycle of ongoing optimization that drives better results over time.
Advanced A/B Testing Techniques
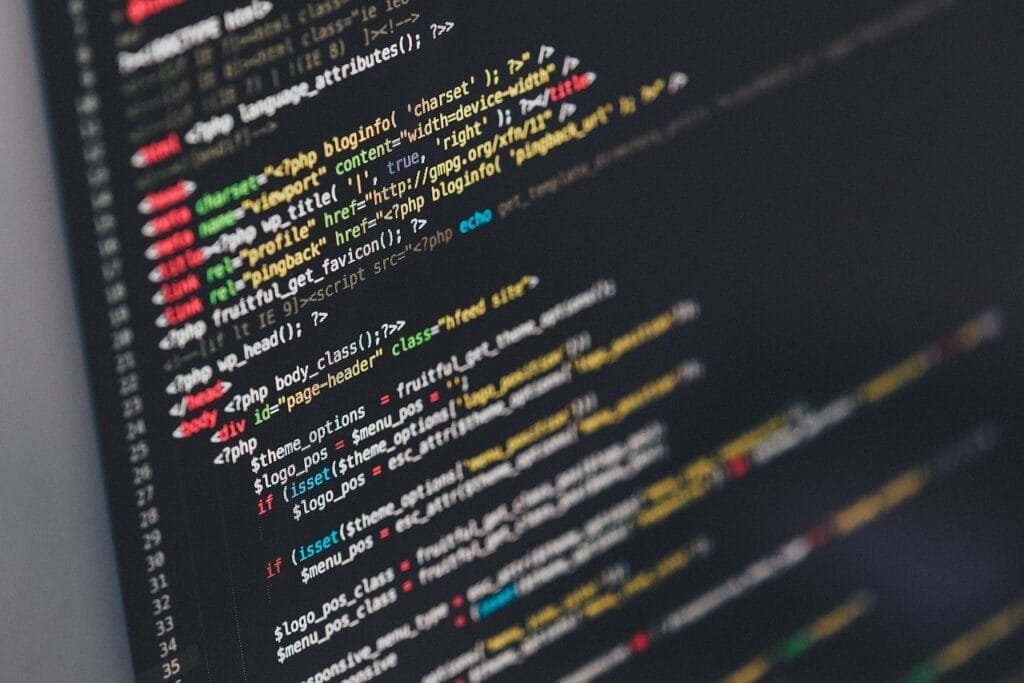
Multi-Armed Bandit Testing
Multi-armed bandit testing is an advanced technique that aims to optimize the allocation of traffic between different versions dynamically. Unlike traditional A/B testing, where traffic is split equally, multi-armed bandit testing allocates more traffic to the better-performing version in real-time.
This approach can lead to quicker insights and improved overall performance. Multi-armed bandit testing is particularly useful when you have low traffic or want to maximize conversions during the testing phase.
By continuously adjusting the traffic split based on performance, this technique helps you identify the best-performing version faster and with greater efficiency.
Sequential Testing
Sequential testing involves running A/B tests in a series, with each test building on the results of the previous one. This method allows you to refine your hypotheses and make incremental improvements over time.
Sequential testing is ideal for long-term optimization efforts, where you want to continually enhance different elements of your content. By focusing on one variable at a time and making data-driven adjustments, you can systematically improve your content’s effectiveness.
This approach ensures that each change is thoroughly tested and validated before moving on to the next element.
Personalization and A/B Testing
Personalization involves tailoring your content to different segments of your audience based on their preferences, behaviors, and demographics. Combining personalization with A/B testing allows you to create highly targeted content that resonates with specific audience segments.
For example, you might test different product recommendations for returning customers versus new visitors. Personalization can significantly enhance user experience and engagement by delivering more relevant content.
A/B testing helps you identify which personalized approaches work best for each segment, enabling you to refine your strategy and maximize impact.
Multi-Channel Testing
Testing your content across multiple channels is essential for a cohesive and effective marketing strategy. Multi-channel testing involves running A/B tests on your website, email campaigns, social media, and other platforms to ensure consistency and optimize performance across all touchpoints.
For instance, you might test different ad creatives on social media while simultaneously testing landing page variations on your website. This holistic approach helps you understand how different elements interact and perform in various contexts, providing a comprehensive view of your content’s effectiveness.
By aligning your optimization efforts across channels, you can create a seamless and impactful user experience.
Utilizing AI and Machine Learning
Incorporating AI and machine learning into your A/B testing strategy can enhance the efficiency and accuracy of your experiments. AI-powered tools can analyze vast amounts of data quickly, identify patterns, and provide predictive insights.
Machine learning algorithms can automatically adjust test parameters and traffic allocation based on real-time performance, optimizing your tests continuously. These technologies can also help you uncover hidden correlations and insights that might not be immediately apparent.
By leveraging AI and machine learning, you can streamline your A/B testing process, gain deeper insights, and make more informed decisions.
Overcoming Challenges in Advanced A/B Testing
Advanced A/B testing techniques come with their own set of challenges. One common issue is the complexity of managing and analyzing multiple variables and segments. To overcome this, use robust testing tools and platforms that offer advanced analytics and automation capabilities.
Ensuring data accuracy and reliability is another challenge, especially with personalized and multi-channel tests. Implement rigorous data validation processes and maintain consistency in how you collect and analyze data.
Additionally, it’s essential to keep your audience’s privacy and data security in mind when implementing personalized and AI-driven testing strategies. By addressing these challenges proactively, you can effectively leverage advanced A/B testing techniques to drive continuous improvement and achieve your marketing goals.
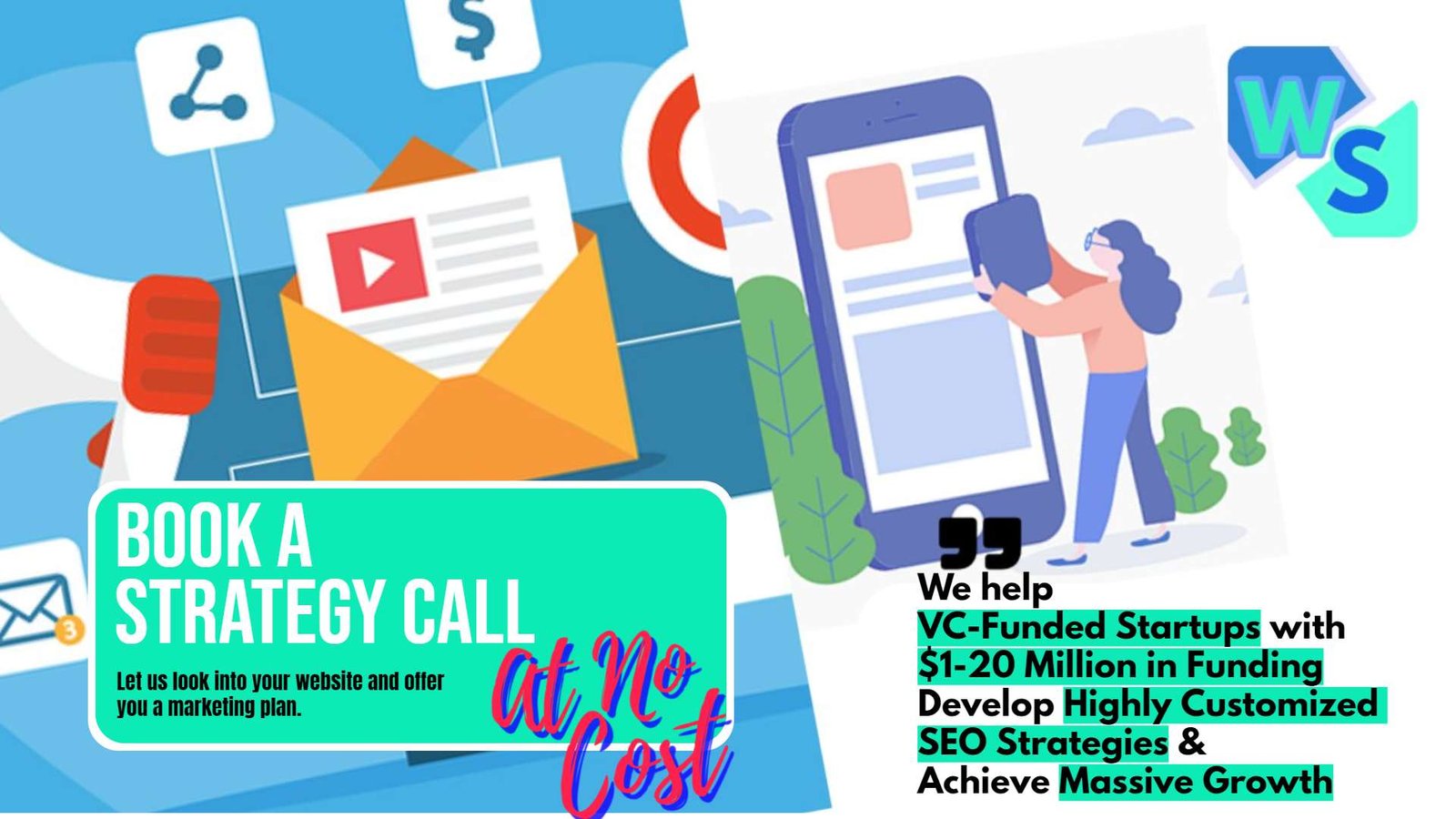
Implementing A/B Testing Tools
Choosing the Right A/B Testing Tool
Selecting the right A/B testing tool is crucial for the success of your experiments. There are numerous tools available, each offering different features and capabilities. When choosing a tool, consider factors such as ease of use, integration with your existing platforms, and the level of support provided.
Some popular A/B testing tools include Google Optimize, Optimizely, VWO (Visual Website Optimizer), and Adobe Target. Each tool has its strengths, so it’s important to evaluate them based on your specific needs and objectives.
Integrating A/B Testing Tools with Your Platforms
Once you’ve selected an A/B testing tool, the next step is to integrate it with your existing platforms. This typically involves adding a small snippet of code to your website or using a plugin if you’re using a content management system (CMS) like WordPress.
Proper integration ensures that your A/B tests run smoothly and that you can accurately track and analyze data. Make sure to follow the tool’s documentation and best practices during the integration process to avoid any issues.
Setting Up Your First Test with an A/B Testing Tool
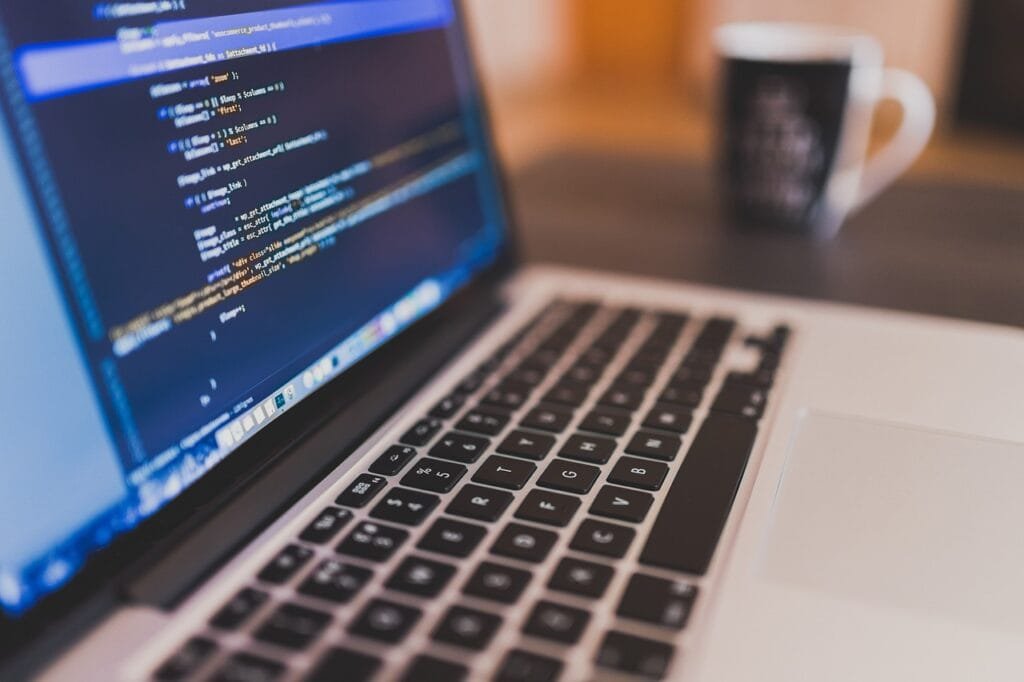
After integrating your A/B testing tool, it’s time to set up your first test. Begin by logging into the tool’s dashboard and creating a new experiment. Define the element you want to test, such as a headline, image, or CTA, and create the variations for your test.
Specify the audience segments for each variation and set the goals and metrics you want to track. Most tools offer a visual editor that makes it easy to create and modify variations without needing advanced technical skills.
Monitoring and Managing Your Test
Once your test is live, it’s important to monitor its progress and make any necessary adjustments. Regularly check the performance metrics and ensure that your test is running smoothly.
Some A/B testing tools offer real-time analytics, allowing you to see how each variation is performing as the test progresses. If you notice any issues, such as one variation significantly underperforming or technical glitches, address them promptly to ensure the validity of your test.
Analyzing Test Results with A/B Testing Tools
After your test has run for a sufficient period, analyze the results using the tool’s analytics features. Most A/B testing tools provide detailed reports that show the performance of each variation based on your defined metrics.
Look for statistically significant differences between the variations and identify the winning version. Some tools also offer advanced analytics, such as heatmaps and session recordings, to give you deeper insights into user behavior.
Implementing the Winning Variation
Once you’ve identified the winning variation, implement it across your platform. Update your website, emails, or other content to reflect the changes that performed best in your test.
Document the insights gained from the test and use them to inform future experiments. Continuous iteration and implementation of successful variations are key to optimizing your content strategy.
Scaling A/B Testing Efforts
As you become more comfortable with A/B testing, consider scaling your efforts to include more complex and frequent tests. Develop a testing roadmap that outlines the elements you plan to test and the order in which you’ll conduct the experiments.
Scaling your A/B testing efforts allows you to continuously optimize your content and stay ahead of the competition. Regularly review your testing strategy and adjust it based on the insights and results from your experiments.
Leveraging Data from Multiple Tests
Running multiple A/B tests over time provides a wealth of data that can be leveraged to improve your overall content strategy. Look for patterns and trends across different tests to identify what consistently works well for your audience.
Use this data to create best practices and guidelines for your content creation and optimization efforts. By analyzing and synthesizing data from multiple tests, you can make more informed and strategic decisions.
Ensuring Long-Term Success with A/B Testing
To ensure long-term success with A/B testing, adopt a culture of continuous improvement within your organization. Encourage team members to regularly test new ideas and approaches, and make data-driven decisions based on the insights gained from A/B testing.
Stay updated with the latest trends and advancements in A/B testing technology and methodologies. By fostering a mindset of experimentation and optimization, you can drive ongoing success and achieve your marketing goals.
The Future of A/B Testing
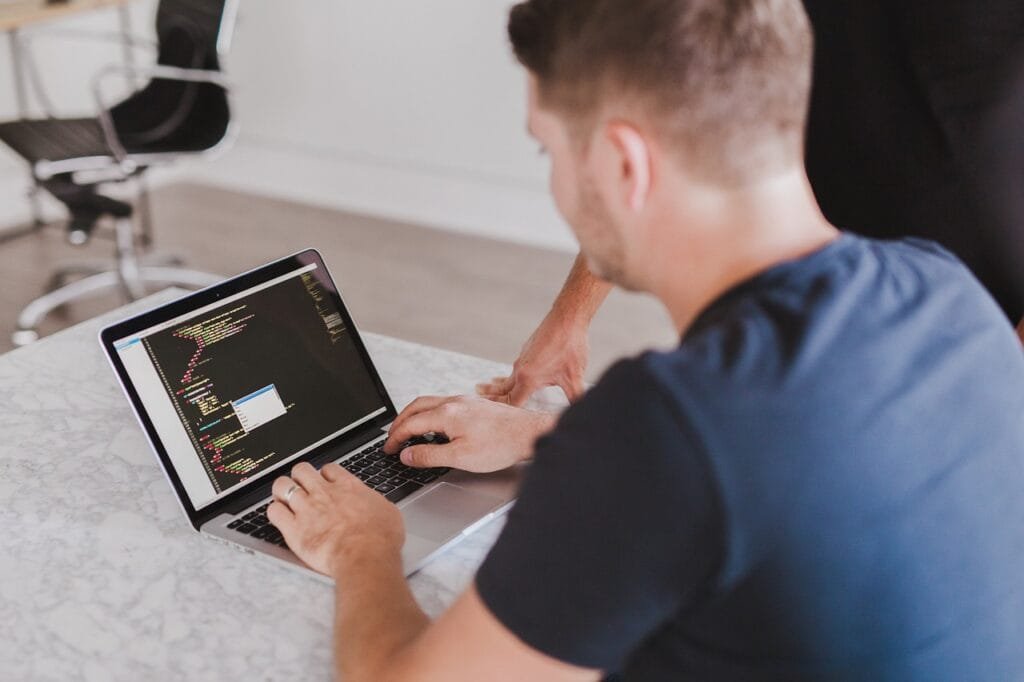
Emerging Trends in A/B Testing
As technology advances, new trends are emerging in A/B testing that promise to make the process even more effective and efficient. One major trend is the integration of AI and machine learning, which can automate parts of the testing process and provide deeper insights.
Another trend is the use of predictive analytics to forecast the potential outcomes of tests before they are even run, saving time and resources. Additionally, the rise of omnichannel marketing is driving the need for A/B testing across multiple platforms and devices to ensure a seamless user experience.
The Role of AI and Machine Learning
AI and machine learning are set to revolutionize A/B testing by enabling more sophisticated analysis and optimization. Machine learning algorithms can process vast amounts of data quickly, identify patterns, and make real-time adjustments to tests.
This allows marketers to conduct more complex tests and gain insights faster. AI can also help in personalizing content for different audience segments, improving the relevance and effectiveness of marketing efforts. As AI and machine learning technologies continue to evolve, they will play an increasingly central role in A/B testing strategies.
Predictive Analytics in A/B Testing
Predictive analytics involves using historical data and machine learning models to predict future outcomes. In A/B testing, predictive analytics can help forecast which version of a test is likely to perform better before the test is completed.
This can save time and resources by focusing efforts on the most promising variations. Predictive analytics can also help identify trends and patterns in user behavior, providing deeper insights into what drives engagement and conversions.
By incorporating predictive analytics into your A/B testing strategy, you can make more informed decisions and optimize your content more effectively.
Real-Time A/B Testing
Real-time A/B testing is another emerging trend that promises to enhance the efficiency and impact of experiments. Traditional A/B tests require running the test for a set period to gather sufficient data.
In contrast, real-time A/B testing uses advanced algorithms to analyze data and adjust traffic allocation dynamically. This approach allows you to identify winning variations more quickly and implement changes faster.
Real-time A/B testing is particularly useful in fast-paced environments where timely decisions are critical. As real-time testing technologies become more accessible, they will offer new opportunities for rapid optimization.
Cross-Device and Omnichannel Testing
With the increasing prevalence of multi-device and omnichannel user journeys, A/B testing must adapt to ensure consistency and effectiveness across all touchpoints. Cross-device and omnichannel testing involve running experiments on websites, mobile apps, email campaigns, social media, and other platforms simultaneously.
This approach helps you understand how users interact with your content across different devices and channels, providing a holistic view of your marketing efforts. By optimizing content for a seamless user experience across all touchpoints, you can enhance engagement and drive better results.
Ethical Considerations in A/B Testing
As A/B testing becomes more sophisticated, it’s important to consider the ethical implications. Ensuring user privacy and data security is paramount, especially when conducting personalized tests. Obtain user consent and be transparent about how data will be used.
Additionally, avoid manipulating or deceiving users with misleading variations. Ethical A/B testing practices build trust with your audience and maintain the integrity of your experiments. By prioritizing ethical considerations, you can conduct effective A/B tests while respecting user rights and privacy.
Preparing for the Future of A/B Testing
To prepare for the future of A/B testing, stay updated with the latest trends and technologies. Invest in tools and platforms that offer advanced features, such as AI integration and real-time analytics.
Foster a culture of continuous learning and experimentation within your organization, encouraging team members to stay informed and embrace new approaches. By staying ahead of the curve, you can leverage emerging trends and technologies to enhance your A/B testing strategy and achieve better results.
Conclusion
A/B testing is an essential tool for optimizing your content and improving your overall marketing strategy. By understanding the basics, setting clear goals, and choosing the right metrics, you can conduct effective A/B tests that provide valuable insights. Advanced techniques, such as multi-variable testing, personalization, and AI integration, offer even greater opportunities for optimization. Overcoming common challenges and leveraging data from multiple tests ensures continuous improvement and long-term success.
The future of A/B testing is bright, with emerging trends and technologies promising to make the process even more powerful and efficient. By staying informed and embracing new approaches, you can keep your content strategy ahead of the competition and achieve your marketing goals.
Read Next
- The Power of Data Analytics in Refining Your Digital Marketing Strategy
- Niche Digital Marketing: Targeting Specific Audiences for Maximum Impact
- Digital Marketing for Local Businesses: Engaging Your Community Effectively
- The Synergy of SEO and Digital Marketing: A Winning Combination
- Digital Marketing on a Budget: Maximizing Impact with Limited Resources
Comments are closed.