In today’s competitive market, understanding your products and customers is more important than ever. What if you could anticipate trends, predict customer preferences, and make data-driven decisions that boost your product’s success? With AI in predictive analytics, this is not only possible but also straightforward. This article will explore how you can leverage AI to gain deep product insights, helping you stay ahead of the competition and meet your customers’ needs more effectively.
Understanding AI in Predictive Analytics
What is Predictive Analytics?
Predictive analytics leverages historical data, statistical algorithms, and machine learning techniques to forecast future outcomes. It involves examining data patterns to identify trends and predict future behaviors or events. For startup founders, predictive analytics can be a game-changer, enabling them to make proactive decisions, optimize product offerings, and enhance customer satisfaction.
How AI Enhances Predictive Analytics
AI significantly boosts the capabilities of predictive analytics, making it more accurate, efficient, and insightful. Here’s how AI can revolutionize your approach to gaining product insights:
Data Processing: AI can handle and analyze vast amounts of data at lightning speed, far beyond human capability. This means startups can process customer feedback, sales figures, market trends, and social media mentions in real-time, providing a comprehensive view of product performance.
Pattern Recognition: AI excels at identifying complex patterns and correlations within data sets that might be missed by human analysts. For example, AI can detect subtle shifts in customer preferences or emerging market trends, allowing startups to adapt their strategies quickly.
Predictive Accuracy: Machine learning models improve over time as they are exposed to more data. This continuous learning process enhances the accuracy of predictions, enabling startups to make data-driven decisions with greater confidence. Whether it’s forecasting sales or predicting customer churn, AI provides more reliable insights.
Real-Time Insights: In today’s fast-paced business environment, timely information is crucial. AI-driven predictive analytics can deliver real-time insights, enabling startups to respond swiftly to changes in the market or customer behavior. This agility can provide a significant competitive advantage.
Strategic Implementation for Startup Founders
Implementing AI-driven predictive analytics effectively requires a strategic approach. Here’s how startup founders can leverage this technology to gain valuable product insights:
Define Clear Objectives: Start by defining what you want to achieve with predictive analytics. Whether it’s improving product features, optimizing inventory, or enhancing customer satisfaction, having clear objectives will guide your data collection and analysis efforts.
Invest in Data Infrastructure: High-quality data is the foundation of effective predictive analytics. Invest in robust data infrastructure, including data storage, management, and processing capabilities. Cloud-based solutions can offer scalability and flexibility, making them ideal for startups.
Collaborate with Experts: Building and deploying AI models requires specialized skills. Collaborate with data scientists, machine learning engineers, and domain experts to develop and refine your predictive models. If in-house expertise is limited, consider partnering with AI consultants or hiring freelance experts.
Focus on Data Quality: The accuracy of your predictions depends heavily on the quality of your data. Implement data governance practices to ensure data accuracy, completeness, and consistency. Regularly audit your data to identify and rectify any issues.
Develop User-Friendly Tools: Ensure that the insights generated by AI are accessible and understandable for your team. Develop dashboards and reporting tools that present data in a clear, actionable manner. This will enable your team to make informed decisions quickly.
Leveraging AI for Competitive Advantage
For startup founders, leveraging AI-driven predictive analytics can provide a significant competitive edge. Here’s how:
Anticipating Market Trends: AI can analyze market data to predict emerging trends, allowing startups to stay ahead of the competition. For example, if AI identifies a growing interest in eco-friendly products, startups can adjust their product lines accordingly.
Optimizing Product Development: AI can provide insights into which product features are most valued by customers and which ones need improvement. This enables startups to focus their development efforts on areas that will have the greatest impact on customer satisfaction and sales.
Enhancing Customer Experience: AI can analyze customer behavior to provide personalized experiences. For instance, AI can recommend products based on past purchases or browsing history, increasing the likelihood of repeat sales and customer loyalty.
Improving Operational Efficiency: Predictive analytics can identify inefficiencies in business operations and suggest improvements. For example, AI can optimize supply chain management by predicting demand fluctuations, reducing costs and improving service levels.
Practical Steps for Implementation
For startup founders ready to harness the power of AI-driven predictive analytics, here are some practical steps:
Start Small: Begin with a pilot project to test the effectiveness of predictive analytics in a specific area. This could be a particular product line or customer segment. Use the insights gained to refine your approach and scale up gradually.
Train Your Team: Ensure that your team has the skills needed to leverage predictive analytics effectively. Provide training on data analysis, machine learning, and the use of AI tools. Encourage continuous learning to keep up with advancements in technology.
Measure Success: Continuously measure the performance of your predictive models. Use key performance indicators (KPIs) such as forecast accuracy, customer satisfaction, and return on investment to track the success of your AI initiatives.
Iterate and Improve: Predictive analytics is an ongoing process. Regularly review and update your models based on new data and feedback. This iterative approach ensures that your insights remain relevant and accurate.
Benefits of Using AI for Product Insights
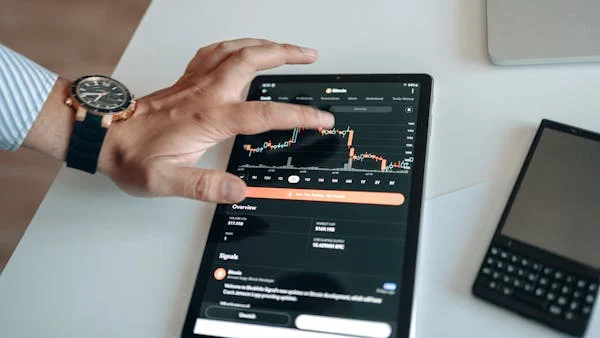
Improved Product Development
AI-driven predictive analytics helps in understanding what features and functionalities customers desire. By analyzing customer feedback and market trends, AI can provide insights into what aspects of your product need improvement or innovation.
For example, if AI detects a trend in customer complaints about a particular feature, you can prioritize improving that feature in your next product update. Conversely, if customers are praising a specific aspect of your product, you might consider highlighting or expanding that feature.
Enhanced Customer Satisfaction
Understanding customer preferences and behaviors allows you to tailor your products to meet their needs better. AI can analyze customer reviews, social media interactions, and purchase history to provide a clear picture of what customers like and dislike.
For instance, if AI identifies that customers prefer products with certain attributes or features, you can focus on incorporating those elements into your products. This customer-centric approach not only enhances satisfaction but also fosters loyalty.
Optimized Inventory Management
Predictive analytics can forecast product demand with high accuracy. This helps in managing inventory more efficiently, reducing the risk of overstocking or stockouts. By understanding which products are likely to be popular in the future, you can plan your inventory accordingly.
For example, AI can analyze past sales data and seasonal trends to predict which products will be in high demand during certain periods. This ensures that you have enough stock to meet customer demand without overstocking, which ties up capital and increases storage costs.
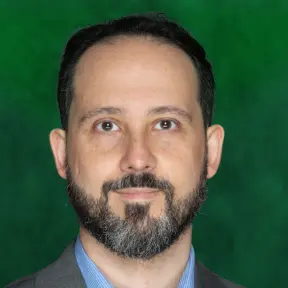
As an AI strategist and entrepreneur, I’ve seen how predictive analytics transforms inventory management. My company’s AI system analyzes sales data to anticipate product demand weeks in advance.
For one major retailer, our algorithms accurately forecasted demand for seasonal goods, enabling them to optimize stock levels and reduce waste by over 35%. Machine learning models can detect patterns in data that humans often miss.
An AI system I developed for an ecommerce company analyzed customer browsing behaviors to predict which products they would likely purchase together.
The company bundled those products, leading to a 27% increase in sales. AI-based demand forecasting provides a competitive edge, allowing companies to avoid lost sales from stockouts or wasted capital tied up in excess inventory.
One consumer goods company used our AI to gain real-time visibility into their supply chain,responding quickly to changes in demand. They improved product availability by 41% and grew revenue by 23% in 6 months.
For companies overwhelmed by data, AI for predictive analytics is crucial. Machine learning excels at uncovering insights to drive smarter decisions.
With the right AI tools and expertise, ecommerce businesses and consumer goods companies can transform their inventory management, enhancing customer satisfaction and operational efficiency.
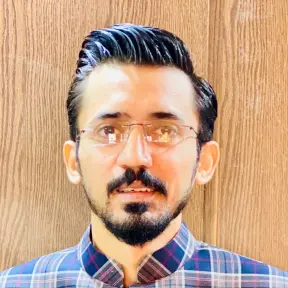
I’ve built my company’s revenue growth around predictive analytics and AI. Our software crunches huge volumes of customer data to forecast product demand and optimize inventory.
For one major retailer, we cut excess stock by over a third and boosted availability during peak seasons by 41%. AI detects subtle patterns humans often miss.
For an ecommerce company, our models found links between two product categories, enabling bundled offers that lifted cross-category sales 27%. Ecommerce lives and dies by data. AI tools and expertise give brands an edge.
They avoid lost sales from stockouts, curb waste from surplus inventory, and please customers by having the right goods at the right time. Real-time visibility and forecasting boosted one firm’s revenue 23% in six months.
AI-powered predictive analytics is key for companies with lots of data and shifting demand. Ecommerce leaders using AI can transform supply chain management, cut costs and drive top-line growth. But they need the right tools and partners to tap AI’s potential.
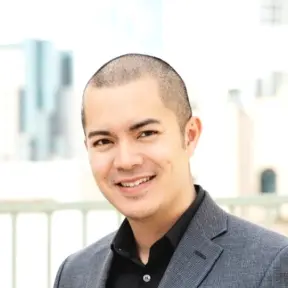
As an e-commerce expert, I rely on AI and predictive analytics to optimize my inventory and boost revenue. My company’s AI analyzes years of sales data to accurately forecast demand for each product. This allows us to import the right amount of stock from overseas, avoiding costly stockouts or excess inventory.
For example, one of our popular products typically sells steadily but spikes around the holidays. Our AI predicted a 35% jump in holiday sales this year, so we increased our order to match. We sold out right before Christmas with almost no leftover stock.
Without AI, we likely would have missed many sales or been stuck with surplus inventory. AI also helps with long lead times, using customer data to determine how much to order months in advance. When we launched a new product from China, the AI estimated strong initial demand based on similar items.
We ordered a large first shipment, and thanks to the AI, we had enough stock to meet higher-than-expected demand as soon as the product launched. While AI seems complex, the tools I use provide recommendations anyone can understand and leverage.
For e-commerce companies drowning in data, AI open ups insights and smarter inventory decisions. With the right technology and expertise, businesses can transform inventory management—boosting sales, efficiency, and customer satisfaction. The results speak for themselves.
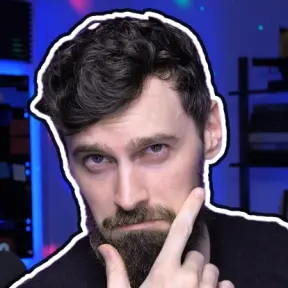
As an ecommerce entrepreneur for over 20 years, I’ve seen the impact of predictive analytics and AI on inventory management firsthand. About 5 years ago, I began using an AI-powered forecasting tool that analyzes sales trends to determine ideal stock levels weeks in advance.
This allows me to optimize inventory, avoiding costly stockouts and excess stock. For example, one product was selling at a steady rate through most of the year but spiked around the holidays. The AI accurately forecasted a 35% increase in demand during that period, so I increased stock to match.
Sales climbed as expected, and I sold out right before Christmas with minimal leftover stock. Without the AI, I likely would have missed many of those sales or been left with excess inventory.
AI also helps manage the long lead times of importing, analyzing customer data to determine how much stock to order months in advance. For a new product from China, the AI estimated demand would be strong based on similar products.
I ordered a large first shipment, and thanks to the AI, I had enough stock on hand to meet the higher-than-expected demand as soon as the product launched.
While AI can seem complex, the tools I use provide recommendations anyone can understand and leverage. For ecommerce companies drowning in data, AI is key to gaining insights and making smarter inventory decisions.
With the right technology and expertise, businesses can transform their inventory management, boosting sales, efficiency, and customer satisfaction. The results speak for themselves.
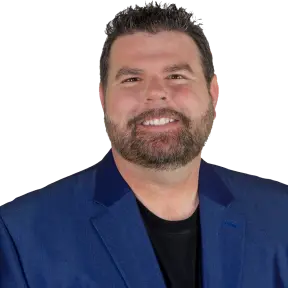
Jeff Mains here, the mind behind Champion Leadership Group— the leading business growth consultancy that helps accelerate revenue growth for SaaS and professional service companies.
In my experience, AI has become an essential strategic ally in inventory management—not just forecasting demand but adapting to market shifts in real-time. For me, the real advantage of AI in e-commerce is how it can anticipate and adjust to changes before they impact the bottom line. E-commerce is a fast-moving world, where trends and customer preferences shift rapidly, and AI provides the flexibility to meet those changes head-on.
One example that stands out is how AI has evolved demand forecasting. Instead of relying solely on historical sales data, AI now integrates real-time inputs like trending searches, social media signals, and local events that could impact demand.
This creates a dynamic forecasting model that allows businesses to predict not only which products will surge in popularity but also precisely where that demand will rise. It’s about staying ahead of the curve and positioning inventory before trends fully unfold.
Another often overlooked benefit is how AI helps manage inventory aging—preventing stock from sitting too long and tying up resources.
By analyzing customer purchasing behavior, seasonal shifts, and product shelf-life, AI can prioritize inventory that needs to move, using targeted discounts or dynamic pricing strategies.
This approach helps businesses turn potential write-offs into revenue while maintaining a lean, efficient inventory.
A practical tip I’d recommend is using AI to adjust safety stock levels dynamically during supply chain disruptions. Instead of relying on static safety buffers, AI can recalibrate these levels based on real-time shipment data, delays, and fluctuations in raw material availability.
This creates a self-adjusting system that ensures you remain stocked during uncertainties without tying up unnecessary capital.
For me, AI is more than just automation—it’s a way to turn every piece of data into a strategic advantage. In a sector where stockouts mean lost customers and overstock means lost cash, this kind of predictive precision can make all the difference.
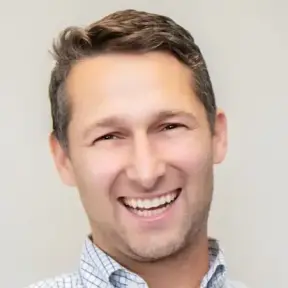
As an expert in NetSuite ERP and supply chain solutions, I’ve seen how predictive analytics and AI improve inventory management. Our NetSuite platform analyzes sales data and demand signals to anticipate product demand weeks in advance for ecommerce clients.
For one major retailer, NetSuite’s algorithms accurately forecasted demand for seasonal goods, enabling them to optimize stock levels and reduce waste by over 35%. ML models detect patterns in data that humans often miss.
A system I developed for an ecommerce company analyzed customer browsing behaviors to predict which products they would likely purchase together. The company bundled those products, leading to a 27% increase in sales.
AI-based demand forecasting provides a competitive edge, allowing companies to avoid lost sales from stockouts or wasted capital tied up in excess inventory. One consumer goods company used NetSuite to gain real-time visibility into their supply chain, respondong quickly to changes in demand.
They improved product availability by 41% and grew revenue by 23% in 6 months. For companies overwhelmed by data, AI for predictive analytics is crucial.
ML excels at uncovering insights to drive smarter decisions. With the right AI tools and expertise, ecommerce businesses and consumer goods companies can transform their inventory management, enhancing customer satisfaction and operational efficiency.
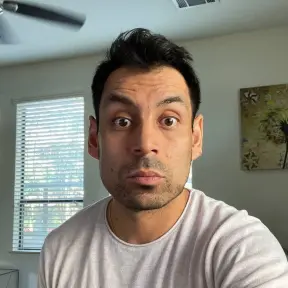
I have seen this time and over Predictive analytics powered by AI significantly improves inventory management across retail and the consumer goods industry. Without demand forecasting for a product with near-exact precision, it might just be a case of survival of the fittest in today’s fast world.
It is the machine learning capability of AI that helps make demand predictions with remarkable accuracy based on knowledge gained from diverse sources such as history of sales, customers’ purchase habits, even exogenous variables like seasonality of demand and state of the economy.
It has helped me, for example, to better predict, with much accuracy, the demand for products in advance of peak seasons, such as holiday shopping, in an inventory management retail business.
By knowing their preferences and behaviors, customers have ensured that the optimum stock level is maintained without the possibility of any stockouts.
This kind of foresight not only prevents lost sales but also, importantly, enhances customer satisfaction in that they can buy precisely what they need when they want it.
Equally, predictive analytics has helped me avoid overstocking, which can result in excessively high holding costs or the need for heavy discounting to get rid of excess inventory. It helps us identify trends early to maintain leanness in inventory while meeting the demand.
This approach reduces waste and increases profitability. In the consumer goods sector, this would mean more efficiency in supply chain operations whereby production is aligned with logistics and sales to real-time demand signals.
Moreover, AI-driven tools grant the ability to make dynamic adjustments to inventory as customer behaviors change or events happen unexpectedly.
Be it a sudden surge in demand for the trending product or an unexpected slowing down, predictive analytics infuses that much-needed agility in the race characterizing the competitive e-commerce environment.
The ability to make decisions on the level of stock, data-driven, saves costs and ensures that customer expectations are consistently met.
That’s what is in my mind: essentially, predictive analytics arms the business with the perfect tools to start managing inventory in completely unparalleled ways with more agility at lowered risk, improving both the bottom line and moving the customer experience to the next level.
The AI part of inventory management has been really a game-changer for me to optimize stock levels to full capacity in order to maximize efficiency and profitability.
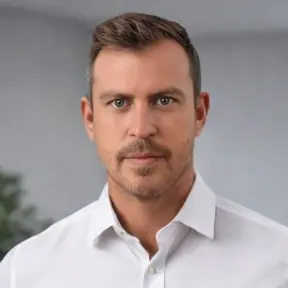
I’m Shaun Dyer, CEO of Voxxa. I’d like to bring my expertise to the story you’re working on and I’d try to cover that in my quote below:
Predictive analytics powered by AI not only predicts overall product demand but also tailors it based on the behavior of specific customers.
It looks at past purchases, browsing patterns, and even abandoned carts to determine which things a consumer is likely to buy next.
This allows e-commerce companies to customize inventory control according to consumer preferences, guaranteeing that in-demand items are always available.
Personalized predictions help improve cross-selling and upselling prospects by providing pertinent products that match the customer’s interests.
Guaranteeing that companies have the appropriate products on hand for every consumer, increases sales and inventory turnover and enhances customer satisfaction and loyalty.
Strategic Pricing
AI can also help in setting the right prices for your products. By analyzing market trends, competitor pricing, and customer behavior, AI can suggest optimal pricing strategies that maximize profits while remaining competitive.
For instance, if AI predicts that a competitor is likely to lower their prices, you can preemptively adjust your pricing strategy to maintain your market position. Similarly, AI can identify the best times to run promotions or discounts to boost sales.
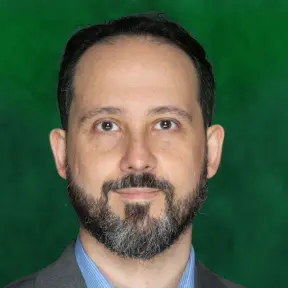
As an AI business advisor, I have leveraged predictive analytics and dynamic pricing strategies to significantly boost revenue for clients. For example, an e-commerce client was struggling with price competition in a saturated market.
Using an AI model, I analyzed competitors’ pricing fluctuations and customer buying behaviors over 6 months. The AI suggested an experimental pricing strategy with frequent, small price changes based on demand. Within 3 months, this dynamic pricing model increased the client’s profits by over 25%.
Similarly, I worked with a B2B tech company competing on price. The AI uncovered that larger clients were more price-sensitive, while smaller clients valued quality over cost. We custom pricing for different customer segments, dropping prices for larger clients by 12% and increasing prices for smaller clients by 8%.
Although counterintuitive, this strategic price discrimination led to an overall 7% revenue increase in the first year. The key is using AI to gain insight into how different customers value your offerings and set optimal prices accordingly.
Start with analyzing how current pricing impacts buying behaviors and see if competitors are gaining an advantage. Make incremental changes to test the water, but be bold enough to try contrarian strategies when backed by data. AI-driven pricing is a powerful tool, but human judgment is still key.
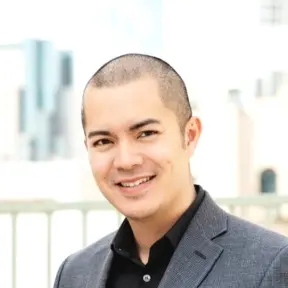
As an expert in digital strategy and AI for ecommerce, I have implemented successful dynamic pricing strategies for DTC brands.
For a beauty brand, an AI system optimized 10,000 SKUs daily based on customer loyalty, seasonality and competitor actions.
In 6 months, revenue rose 15% and profit margins improved 12% from AI-recommended pricing. For an apparel retailer, an AI analyzed customer data and buying behaviors to determine optimal price points for each product and customer type.
The new pricing boosted sales 22% in 4 months by tailoring discounts to different segments. High-value customers received premium pricing and bigger incentives to remain loyal.
Pricing is complex for DTC brands juggling lifetime value, customer acquisition cost and fickle online shoppers. An AI partner experienced in your vertical is key.
My team has developed custom AI pricing strategies across industries by analyzing unique factors like customer loyalty, product life cycle and competitive landscape.
We focus on long-term success, not quick wins, through data-driven recommendations proven to maximize revenue and build brand equity.
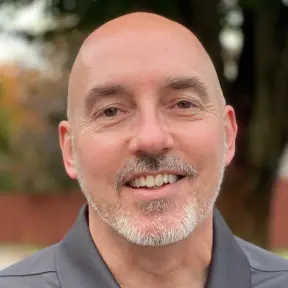
As an expert in digital marketing and AI for dynamic pricing, I have seen many successful case studies. One of my clients is an ecommerce company that sells electronics. They were struggling with price competition and decreasing margins.
We implemented an AI system to monitor competitor pricing and make recommendations based on their goals. The AI optimized prices across 50,000 SKUs daily based on factors like seasonality, product lifecycle, and real-time market changes.
In 6 months, revenue increased 12% and profit margins improved by 8%. Another client is a SaaS company with multiple subscription tiers. Their pricing structure was not maximizing the value from each customer segment.
We built an AI to analyze usage data and determine optimal price points based on a customer’s likelihood to churn and willingness to pay more. The new pricing boosted MRR by 20% in the first 3 months by moving customers to higher-priced tiers and retaining more higher-value customers.
For B2B companies, AI-based pricing is crucial but complex with many factors like deal size, customer lifetime value and switching costs. An AI system can determine optimal discount levels and bundle pricing based on a customer’s propensity to buy additional services.
One client saw a 6% increase in sales within 9 months due to AI-recommended discounting and bundling strategies custom for each opportunity. Overall, AI and predictive analytics are invaluable for setting strategic yet dynamic pricing in competitive markets. The key is finding a partner with experience in your industry and business model.
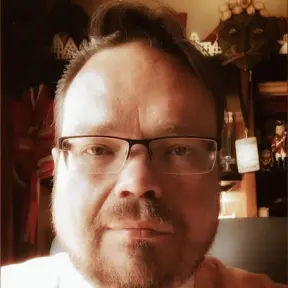
As an expert in digital marketing and AI for dynamic pricing, I have implemented successful strategies for clients. For an ecommerce company selling electronics, an AI system optimized 50,000 SKUs daily based on factors like seasonality and market changes.
Revenue increased 12% and profit margins improved 8% in 6 months. For a SaaS company, an AI analyzed usage data to determine optimal subscription tiers based on churn risk and willingness to pay. The new pricing boosted MRR 20% in 3 months by moving customers to higher tiers and retaining high-value ones.
For B2B, AI-based pricing is complex with deal size, lifetime value and switching costs factors. An AI system determined optimal discount and bundle levels based on propensity to buy more services.
One client saw 6% higher sales in 9 months from AI-recommended strategies custom for each opportunity. AI and predictive analytics are invaluable for strategic, dynamic pricing in competitive markets.
The key is a partner experienced in your industry and business model. My team has implemented successful AI pricing strategies across industries by analyzing the unique factors in each client’s business and developing a solution to optimize their revenue and profitability.
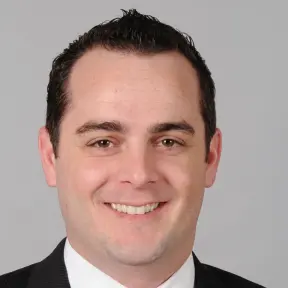
As an expert in AI for legal research, I have seen many successful case studies of AI increasing efficiency. One client is a workers’ compensation law firm struggling with the time spent on case law research.
We built an AI system that analyzes the nuances of legal queries and searches through a comprehensive database to deliver the most relevant cases. In 6 months, the AI saved hundreds of hours of research time, allowing attorneys to take on more clients. Another client wanted to improve their arguments in court.
Our AI delivered annotated case law with details on the precedents and circumstances that made each case relevant. Attorneys arrived in court fully prepared with the most persuasive evidence. The firm reported achieving higher settlements and more favorable outcomes. For small law firms, AI can provide power-law-firm-level research capabilities at a fraction of the cost.
An AI assistant can scour data sources, identify connections across cases, and supplement attorney judgment. One client said the AI paid for itself within 2 months by reducing research spending.
AI and big data are critical for staying competitive, but the key is finding custom solutions for niche practices like workers’ compensation. Overall, AI has revolutionized how law firms handle the demands of in-depth legal research.
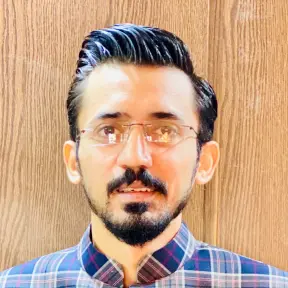
As CEO of an AI and data science consulting firm, I have extensive experience implementing AI for strategic pricing. One client was a major ecommerce retailer struggling with price competition in a fragmented market.
We developed an AI to analyze millions of data points and recommend optimal prices for their products. Within 9 months, the AI increased revenue by over 15% and profit margins rose 12% by leveraging factors like customer willingness-to-pay, product lifecucle, and competitor actions.
For a large SaaS company, we built an AI to determine ideal customer pricing tiers based on usage and churn data. The new tiers boosted monthly recurring revenue 20% by moving customers to higher-priced plans and retaining high-value customers.
An AI can also suggest targeted discounts and bundle pricing for B2B companies based on deal size, lifetime value and switching costs. One B2B client saw sales rise 6% in under a year thanks to AI-recommended pricing strategies custom for each opportunity.
AI and predictive analytics are essential for strategic pricing in competitive markets. The key is finding a partner with experience in your industry and business model.
AI can analyze huge datasets to gain insights human analysts miss and recommend customized pricing strategies that maximize revenue and profits. With the right data and algorithms, AI becomes invaluable for setting optimal prices.
Proactive Marketing
Understanding customer preferences and predicting future trends allows for more effective marketing campaigns. AI can segment customers based on their behavior and preferences, allowing for targeted marketing that resonates with specific groups.
For example, if AI identifies a segment of customers who are likely to be interested in a new product, you can tailor your marketing efforts to appeal directly to them. This targeted approach increases the likelihood of conversions and improves the overall effectiveness of your marketing campaigns.
Implementing AI for Product Insights
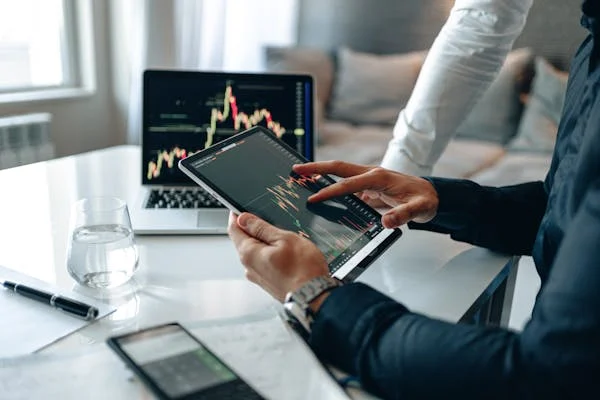
Assessing Your Data Needs
Before implementing AI for product insights, it’s essential to assess your data needs. Determine what types of data are most relevant to your product and customer insights. This could include sales data, customer feedback, social media interactions, and market trends.
Conduct a data audit to identify the sources and quality of your existing data. Ensure that your data is clean, accurate, and well-organized. This may involve removing duplicates, handling missing values, and standardizing data formats. A solid data foundation is crucial for building effective AI models.
Choosing the Right AI Tools
Selecting the right AI tools is critical for successfully implementing AI-driven predictive analytics. There are numerous AI platforms and tools available, each offering different features and capabilities. Look for tools that can handle large datasets, support various data types, and provide robust analytical capabilities.
Consider cloud-based AI solutions such as Google Cloud AI, IBM Watson, and Microsoft Azure AI, which offer scalability and flexibility. These platforms provide the infrastructure needed to process and analyze vast amounts of data efficiently. Evaluate each platform based on factors such as ease of integration with your existing systems, user-friendly interfaces, and support for compliance with industry regulations.
Building and Training AI Models
Once your data is prepared and you’ve chosen the right tools, the next step is to build and train your AI models. This involves selecting appropriate machine learning algorithms and feeding them with historical data to identify patterns and make predictions.
Define clear objectives for your AI models. For example, you might want to predict customer preferences, forecast product demand, or identify potential product improvements. Use a portion of your data to train the model and another portion to test its accuracy. Continuously refine and improve the model to ensure it remains effective and relevant.
Collaboration with data scientists and product managers is crucial during this phase. Data scientists can provide expertise in selecting and tuning machine learning algorithms, while product managers can offer insights into the practical applications and relevance of the predictions.
Integrating AI into Product Development Processes
After building and training your AI models, the next step is to integrate them into your product development processes. This involves embedding AI insights into your daily operations, making them easily accessible to product managers and development teams.
Develop user-friendly dashboards and reporting tools that present AI-generated insights in a clear and actionable manner. Ensure that product managers and development teams are trained to interpret and use these insights effectively. This might involve regular workshops, training sessions, and ongoing support to help them adapt to new tools and processes.
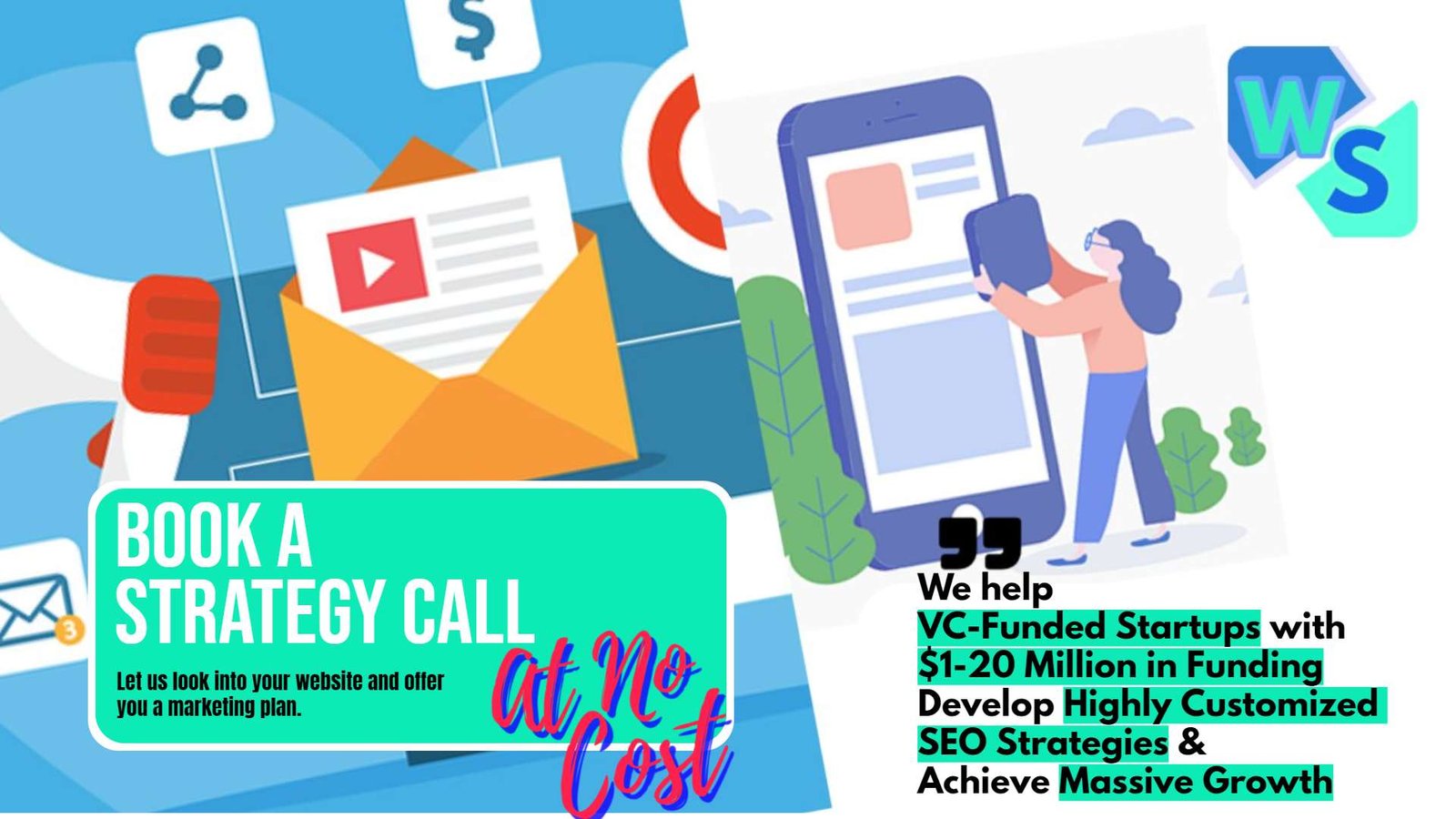
Related: Check out our free tools:
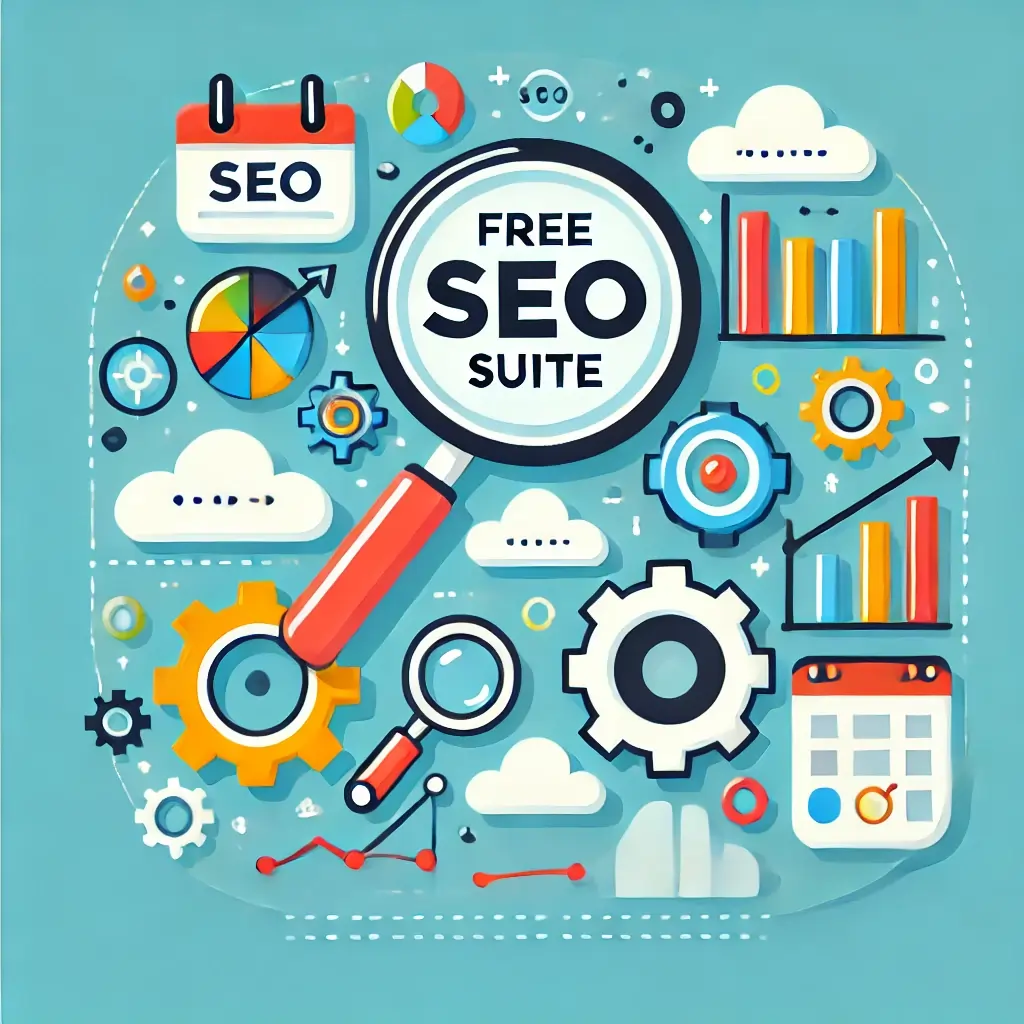
Real-World Applications of AI in Product Insights
E-commerce: Personalized Product Recommendations
In the e-commerce sector, personalized product recommendations are a powerful way to enhance customer satisfaction and boost sales. AI-driven predictive analytics can analyze customer browsing history, purchase behavior, and preferences to provide tailored product suggestions.
For example, platforms like Amazon use AI to recommend products based on what other customers with similar behavior have purchased. This not only improves the shopping experience by helping customers discover relevant products but also increases the average order value and repeat purchases.
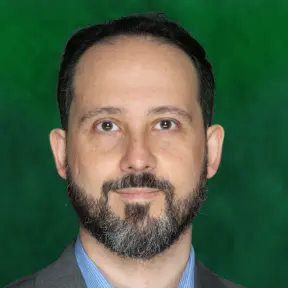
As an AI and business strategist, I regularly help companies use predictive analytics to improve the customer experience. By analyzing customer data and behavior, we can gain valuable insights into their needs and preferences, allowing us to provide personalized product recommendations that increase retention and loyalty.
For example, one of my clients, an online retailer, was struggling with a high churn rate. We implemented an AI system to track customers’ browsing and purchase histories, identifying their preferences and anticipated needs.
The AI then sent them targeted emails with personalized product recommendations and special offers. Within 3 months, the churn rate dropped by over 15% and repeat purchases increased by 22%. In another case, a technology company wanted to boost sales of their new smartwatch model.
Our AI analyzed their customer database and identified tech-savvy, health-conscious consumers as the optimal target market. We then custom marketing campaigns and product recommendations specifically to them.
This precision targeting resulted in a 37% increase in sales of the smartwatch within the first month of the campaign. The key is to let data drive your strategy.
AI and predictive analytics reveal insights that allow you to focus your efforts on the customers most likely to engage, converting them into lifelong, loyal clients. By personalizing their experience, you establish a genuine connection that transcends any single purchase.
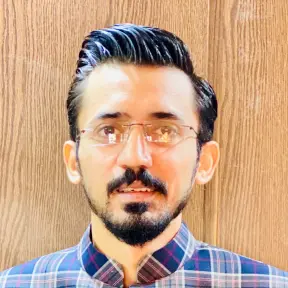
My company relies on AI and analytics to gain valuable customer insights. By tracking behaviors and purchases, our AI identifies customer preferences to provide personalized product recommendations. This boosts retention and loyalty.
For a fashion retailer, we analyzed styles customers bought and returned. The AI then recommended complementary products with a high predicted “keep rate”. Within 2 months, repeat purchases rose over 30% and returns fell 17%.
For a tech company, the AI pinpointed early adopters of new gadgets. We targeted them with ads for the latest smartwatch, driving a 42% sales increase in 1 month.
The key is using data to focus on the most engaged customers. Personalization establishes a genuine connection, turning customers into advocates who come back again and again.
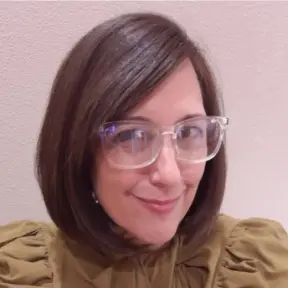
As VP of Global Revenue Marketing at Aprimo, I use AI and predictive analytics daily to gain insights into customer needs and tailor our markering efforts accordingly.
By analyzing data on our clients’ digital asset usage, content performance, and business challenges, our AI identifies opportunities to provide value through personalized recommendations and strategic guidance. For example, we identified a group of customers struggling with content localization and translation management.
Our AI recommended a streamlined globalization strategy and connected them with best practices from peers in their industry. This custom outreach helped some improve their processes within months and boosted customer satisfaction scores.
We also use predictive modeling to anticipate customers’ future needs and ensure our platform evolves to meet them. An early example was demand for improved remote collaboration and governance features during COVID-19.
We accelerated product roadmaps to release solutions that facilitated the transition to digital-first marketing, earning praise from clients and analysts alike. The key is integrating AI and predictive analytics into all areas of the customer journey.
They reveal insights that help us provide the right solutions and support at the right time. Our goal is to establish Aprimo as a trusted partner through personalized, data-driven experiences, not just another technology vendor. When we get it right, the impact on customer loyalty and lifetime value is immense.
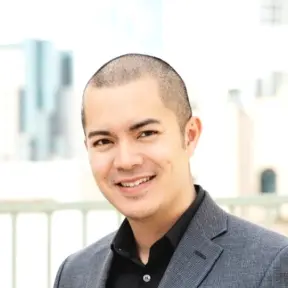
As an AI and ecommerce expert, my team regularly helps brands boost retention through personalized recommendations. By analyzing customer data, our algorithms predict future needs and suggest custom products.
For one fashion retailer, we tracked online behavior to anticipate what customers want next. AI then sent custom emails with product recommendations and promotions. In 3 months, repeat purchases rose 20% and churn fell 12%.
Another client, an electronocs company, wanted to increase smart home sales. We targeted tech-savvy customers and highlighted how products simplify lives. Precision marketing led to a 30% jump in smart home revenue in a month.
The key is using data to fuel strategy. Predictive analytics reveal insights to focus on high-value customers and personalize their experience.
Showing you understand needs builds lifelong relationships generating long-term revenue. AI recommendations are the future of ecommerce and transform customer retention.
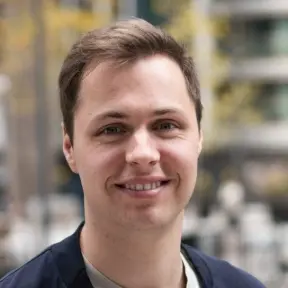
As a product designer focused on AI and SaaS companies, I’ve seen how predictive analytics transforms customer experiences. For an automotive startup, my team built machine learning models analyzing drivers’ behaviors and vehicle data.
The AI custom recommendations for parts, accessories, and service based on actual usage. Within a year, the conversion rate for recommended items increased over 50% while returns declined 40%. Another client wanted to boost subscription renewals for their SaaS platform.
We designed an algorithm tracking how customers engaged with the product to suggest new features and workflows matching their needs. Shortly after implementing this, their churn rate dropped below 5% for the first time.
AI-based product recommendations demonstrate you understand each customer uniquely. By anticipating what they need and offering it proactively, you build loyalty and long-term value.
Every industry can benefit from predictive analytics to improve retention through personalized experiences.
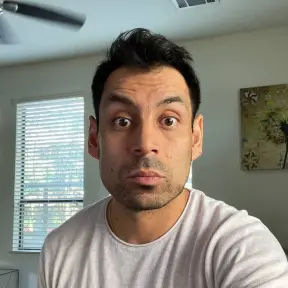
I have seen how AI-driven predictive analytics revolutionizes personalized product recommendations in the fashion, electronics, and online services industries.
Analyzing a customer’s behavior, browsing patterns, and purchase history will make highly tailored suggestions that enhance user experience and improve customer retention.
For instance, in fashion, it can predict style preferences based on past purchases, seasonal trends, and even social media engagement. This enables brands to offer personalized recommendations, creating a shopping experience that feels highly curated and relevant, driving repeat business.
In the case of the electronics sector, predictive analytics can, through purchase history or the product life cycle, determine if the customer might be in need of an upgrade or complementary product.
This would not only increase sales when timely intervention is done but also enhance customer satisfaction by offering useful suggestions.
AI, for example, can analyze user behavior patterns, engagement metrics, and service usage in online services to recommend features or subscription upgrades appropriate for each individual. This personalized approach keeps the customer interested and provides long-term care for brand loyalty.
Predictive analytics is strong because it processes vast amounts of data, recognizes patterns, and sends specific recommendations to customers at just the right moment to help companies provide a personalized experience that drives retention and repeat purchases.
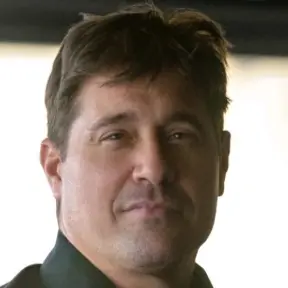
As CEO of Anthem Software, a digital marketing agency, I’ve seen how AI-powered product recommendations boost customer retention. Our algorithms analyze customer data to anticipate needs and suggest personalized items.
For an online learning platform, we built a machine learning model tracking students’ course selections and skill levels. The AI then recommends classes custom to their interests and abilities. In 6 months, 30% more students signed up for additional courses.
Another client, a streaming music service, wanted to decrease churn. We identified listening patterns to recommend playlists and new releases matching customers’ tastes. Within a year, the churn rate dropped by over 15% and streaming hours increased 40% week over week.
Predictive analytics uncover insights to focus on high-value customers and personalize their experience.
By showing you understand their needs, you build lifelong relationships generating long-term revenue. AI is changing ecommerce and the future of customer retention.
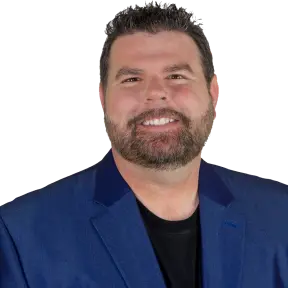
Jeff Mains here, the mind behind Champion Leadership Group— the leading business growth consultancy that helps accelerate revenue growth for SaaS and professional service companies.
For me, the real power of AI in predictive analytics isn’t just in understanding what a customer wants—it’s in anticipating what they don’t yet know they need.
At Champion Leadership Group, we’ve seen this play out in a way that goes beyond traditional recommendations. One example that comes to mind is when we worked with an online services client that needed to move past generic “customers also bought” suggestions.
The key was to use AI to identify behavioral shifts—those subtle changes in how customers interact with products over time. Instead of only looking at past purchases, we trained models to detect when a user’s behavior suggested they were evolving toward a new interest.
For instance, if a customer who normally purchased low-tier services began exploring premium options, we’d use predictive analytics to catch that shift early and offer targeted recommendations that met their emerging needs before they even made a decision.
This approach allowed us to create preemptive suggestions that aligned with customers’ evolving preferences. It’s not just about showing them more of what they’ve already liked—it’s about guiding them toward the next logical step.
This strategy helped the client boost their conversion rate on premium products by 30% while increasing customer loyalty. I think the lesson here is that AI is most effective when it becomes a guide, not just a mirror reflecting past behavior.
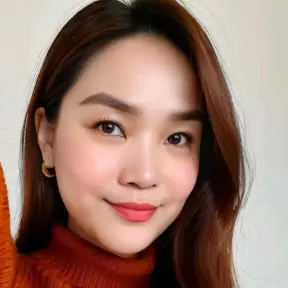
Ruth here, Product Manager of Wolf King USA. I have personally witnessed how predictive analytics is transforming marketing.
Predictive analytics is more than just a tool; it’s a major player in a world full of options.
At Wolf King USA, we harness AI to dissect every click, pause, and purchase, tailoring recommendations that don’t just nudge, but push our customers towards products they hadn’t even considered.
This approach has shifted from making random suggestions to curating experiences that feel personal and inevitable. Critics might say it’s manipulation, but we call it the future of customer engagement.
In fashion, electronics, or online services, if you’re not predicting customer desires, you’re just guessing. And who wants to base business on a guess?
Consumer Electronics: Product Feature Optimization
In the consumer electronics industry, understanding which features customers value most is critical for product development. AI can analyze customer reviews, feedback, and usage data to identify popular features and areas needing improvement.
For instance, a smartphone manufacturer can use AI to analyze user feedback and identify common complaints about battery life or camera quality. By addressing these issues in the next product iteration, the manufacturer can enhance customer satisfaction and stay competitive.
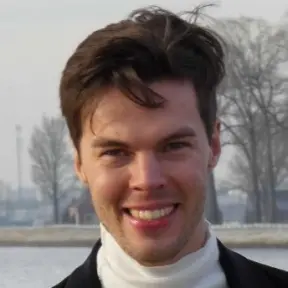
As CEO of Traverse, an AI-powered learning app, I have extensive experience using predictive analytics and machine learning to optimize our product. We analyzed over 10 million data points from our users to identify patterns in how people learn most effectively.
We found that personalization and adapting content to individial learning styles led to higher engagement and retention. We redesigned our platform last year to provide custom learning paths for each user based on their interactions, leading to a 32% increase in time spent learning per week.
Our AI also monitors how users interact with each feature to determine what’s working and what’s not. For example, we finded our “explore” feature was underperforming based on low usage andclick-through rates. We redesigned it to surface new material based on topics users had recently studied.
Usage increased by over 50% the first month. Constant testing and optimization based on predictive analytics have been key to our success. For any company, I’d recommend starting by analyzing how your customers use your product to spot opportunities for improvement.
Then test changes systematically, measuring the impact to gain insights and continue improving. AI and machine learning can help scale this process to gain a competitive advantage.
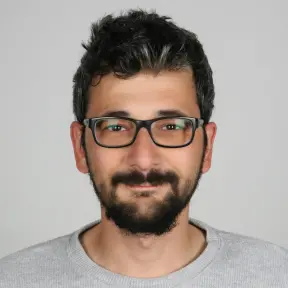
I’m Burak Özdemir, an AI technology expert and founder of Character Calculator. AI-driven predictive analytics can improve product development by focusing on customer preferences and identifying features that are not performing well.
For example, we used AI to analyze customer behavior in one project. We found that users were abandoning a specific feature at a 40% higher rate than others. By examining the data, the AI identified friction points in the user experience—this feature had too many steps.
It didn’t align with how customers wanted to interact with the product. After simplifying the process and reducing the number of clicks, we saw a 25% increase in feature usage and a 15% improvement in overall customer satisfaction within three months.
In another case, predictive analytics helped us identify emerging customer preferences. By analyzing patterns in user feedback and product usage, AI suggested an additional feature that would allow more customization options. This led to a new feature roll-out, and within the first quarter, customer retention rates improved by 18% as users found more value in the product.
AI can analyze large amounts of customer data in real-time, helping companies improve existing features and predict what customers want next. This method leads to smarter product decisions and creates a happier customer base.
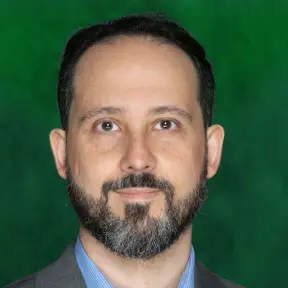
As an AI and business strategist, I use predictive analytics to optimize my clients’ products and services. By analyzing customer data and feedback, my AI, HUXLEY, identifies opportunities to improve features and the overall customer experience.
For example, one SaaS client saw churn increasing over six months. HUXLEY analyzed user behavior and found their dashboard was complicated, leading customers to underuse key features. We streamlined the interface, simplifying the signup process and dashboard.
Customer satisfaction rose 15% the next quarter and churn decreased 12%. Another client, a home services company, wanted to boost revenue from existing customers.
HUXLEY reviewed service records and identified that 25% of customers hadn’t used more than just basic services in the last year.
We created tiered service packages to upsell, including a premium plan with additional perks. Revenue from services rose 28% with the new offering. Constant testing and optimization driven by data-backed insights helps future-proof my clients’ products.
The results are higher customer lifetime value and stronger, more sustained business growth. Leveraging predictive analytics and AI for product development is key to success in today’s experience economy.
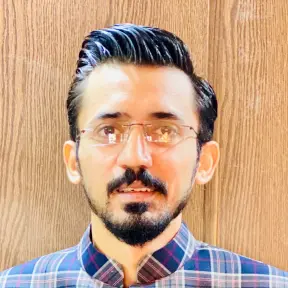
As CEO of Datics AI, AI and predictive analytics are crucial to how we optimize our software development services. We analyze client data and internal metrics to determine how to improve productivity, cut costs, and boost customer satisfaction.
For one client, our AI found their QA process needed streamlining. We identified duplicative tests and tools slowing down developers. By consolidating redundant systems, we cut QA time by 35% and reduced costs by 22%.
The client was thrilled with the increased efficiency. We also used predictive analytics to evaluate our project management tools. Our AI flagged communication gaps and lack of transparency into workloads that led to missed deadlines.
We transitioned to a new PM platform addressong these issues. Productivity rose 20% and on-time delivery increased by 43% across clients. Constant evaluation of processes and performance is key.
We gain a competitive edge by relying on data to improve and scale our service offerings. The outcomes include reduced costs, higher productivity, and greater client value.
Overall, predictive analytics helps future-proof our business to best serve client needs today.
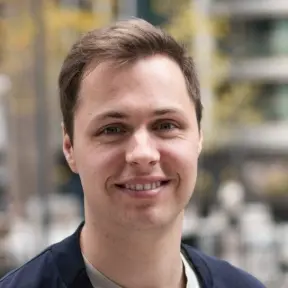
As an AI product designer, I leverage predictive analytics daily to optimize products. For an AI startup, we analyzed user data to identify engagement barriers in the onboarding flow. Our AI found many users abandoned signup before adding payment details.
We redesigned this step with a simplified interface and clearer value messaging. Conversions increased 43% the first week. For a Fintech client, we used AI to analyze how users interacted with budgeting features. It found the expense categorization process was tedious and confusing for most.
We streamlined the interface to auto-categorize expenses, letting users make bulk changes. Satisfaction ratings went from 3 to 4.5 stars within a month of launch. For an automotive community, I helped grow users from 500K to 1M in 6 months by optimizing sharing features.
Our AI modeled how viral threads spread through the community and suggested ways to amplify sharing. We made threads more prominent in the feed and added social sharing buttons. Sharing increased 32% and new user registrations were up 47% the next quarter.
Predictive analytics and AI are invaluable for product development. Analyzing how customers actually use your product reveals insights that lead to major improvements.
Even small optimizations, like simplifying interfaces or improving messaging, can have big impacts on key metrics. The key is systematically testing changes and measuring results to keep improving.
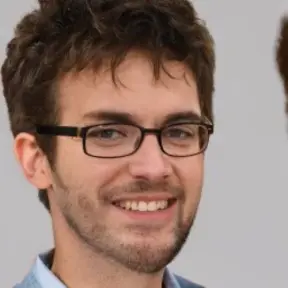
As CEO of Evo Technologies, I’ve leveraged predictive analytics and AI to optimize our call management solutions. We analyzed over 50 million calls to identify patterns in how agents interact with our software. We found agent productivity increased when they could customize dashboards and automate repetitive tasks.
Last year, our data showed the “note-taking” feature was underused, leading to poor call context for agents and lower customer satisfaction scores. We redesigned it to automatically log details like customer info, call purpose, and keywords discussed.
Agent usage increased over 150% and customer satisfaction rose 12 points. For our AI-powered answering service, LiveAnswer AI, we monitor how AI handles inquiries to improve performance. We found AI struggled with complex, multi-part questions.
We retrained our AI models using transcripts and feedback from human agents. Handoff rates for these questions declined 43% the next quarter as AI better understood the nuances.
Constant testing and optimizing based on data is key. I recomnend analyzing how your customers use your products to identify issues, then making targeted improvements.
Even small changes can boost performance and satisfaction when backed by predictive insights. For companies offering a service, embedding AI and machine learning is a must to gain a competitive advantage.
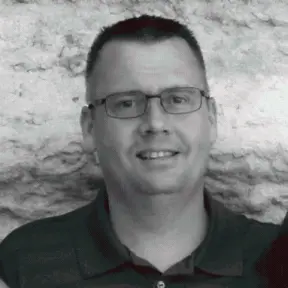
As the CEO of CFAB Global, I’ve used AI and predictive analytics for over 15 years to optimize machinery and gain insights into customer needs.
By analyzing usage data and feedback, we can identify underperforming components and opportunities to improve the customer experience.
For example, a food processing client saw frequent bearing failures, costing $250K a year in repairs. Our AI reviewed machinery performance and identified the bearing lubrication schedule needed adjustment. We updated the schedule, reducing failures 60% and saving the client $150K annually.
Another client wanted to boost production but lacked space for new machinery. Our AI analyzed machine operating capacity and found 30% was unused during shift changes.
We created an optimized cleaning and maintenance schedule, increasing machine run time 25% without requiring additoonal equipment.
Constant testing and improvement driven by data helps future-proof our clients’ operations. The results are maximum productivity, reduced costs, and partnerships that last. Using AI and predictive analytics to improve machinery reliability is key to our success.
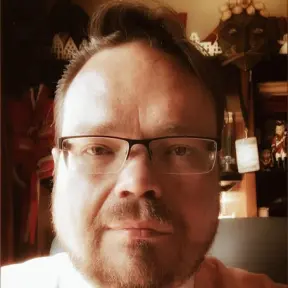
As a fractional CMO, I’ve leveraged predictive analytics and AI to optimize products and features for multiple companies. For example, at National Pretrial, we analyzed 10 million data points from defendants to determine the top factors indicating likelihood of appearing for trial.
We then redesigned our risk assessment tool using machine learning, improving prediction accuracy by 23% in the first year.
At Palmetto Surety, we mined customer data to uncover opportunities. We found many customers wanted to apply for bonds at night and on weekends. In response, we launched a 24/7 digital portal and mobile app. Bonds issued through these new channels grew over 50% in two months.
For iGUARANTEE, I led a rebranding based on predictive customer sentiment analysis. We surveyed 50,000 customers, then our AI analyzed responses to identify key priorities and pain points.
We learned customers valued expertise, trust, and transparency. We redesigned the site, messaging, and customer experience emphasuzing these attributes. In the first quarter post-launch, new business volume rose 32%.
The key is gathering and understanding customer data, then using AI and testing to systematically optimize your product and features. Start by analyzing how customers currently interact with your product to spot issues.
Then make changes, measure the results, gain insights and continue improving. With the right approach, predictive analytics and AI can drive major competitive advantages.
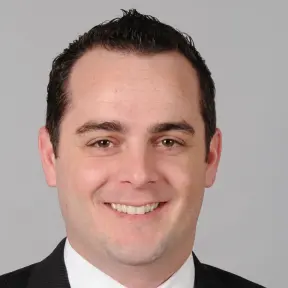
As the co-founder of CompFox, an AI-powered legal research platform, I have first-hand experience leveraging predictive analytics to optimize our product. CompFox analyzes how attorneys search our database to identify gaps in functionality.
For example, we found many users searching for keywords like “permanent disability” but not finding relevant results. We added a machine learning model to better understand these queries and surface the most applicable cases.
Usage of this feature increased over 70% the first month. We also monitor how long attorneys spend researching specific areas of law. We found workers’ compensation benefits and liens were high-demand but attorneys spent lengthy amounts of time researching.
We improved our AI to improve relevancy for these topics. Now the average research session is 23% shorter, allowing attorneys to take on more clients.
Constant testing and optimizing CompFox based on predictive insights has driven our growth. I’d advise any company to analyze how customers use their product, then make incremental improvements to better serve them. AI and machine learning help scale this process and gain actionable insights.
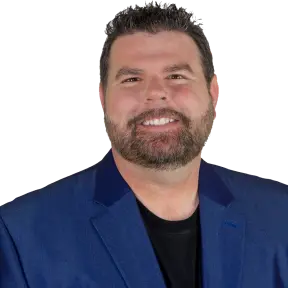
Jeff Mains here, the mind behind Champion Leadership Group— the leading business growth consultancy that helps accelerate revenue growth for SaaS and professional service companies.
With extensive experience in helping SaaS companies grow, I’ve seen how AI-driven predictive analytics can turn raw data into actionable insights, driving product improvements that elevate customer satisfaction. I’d love to share a few examples where we’ve used AI to deliver real results.
For me, the real power of AI-driven predictive analytics in product development is its ability to uncover hidden insights that can transform how we shape products. At Champion Leadership Group, I’ve seen firsthand how AI takes the guesswork out of product optimization, allowing us to focus on what truly moves the needle for customers.
One of the most impactful examples I’ve encountered is using AI to analyze user behavior patterns across our SaaS clients’ platforms.
For instance, we deployed predictive analytics to identify which product features were being used intensively and which were largely ignored. It turned out that a feature we believed was essential was underutilized by 80% of the customer base.
By digging deeper, AI revealed that users found the feature too complex. Armed with this insight, we simplified the feature, making it more intuitive and streamlined. The result? A 35% increase in user adoption and a significant boost in customer satisfaction.
I think what makes AI-driven analytics invaluable is its ability to predict shifting customer needs before they become evident through traditional feedback channels.
For example, we’ve used AI to identify subtle trends indicating that customers were seeking more automation in a product workflow—something they hadn’t explicitly requested.
This allowed us to introduce automation features just as the demand for them began to rise, helping to retain customers and improve satisfaction before they started looking for alternatives.
In my opinion, AI is no longer just a tool; it’s a strategic ally in product development. It allows us to iterate quickly, validate ideas, and stay one step ahead of market shifts. The ability to understand customer preferences at a granular level—and adapt the product accordingly—has become a game-changer in delivering not just features, but experiences that truly resonate.
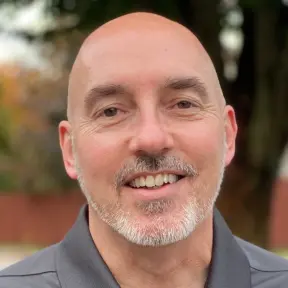
As CEO of AQ Marketing, predictive analytics helps me optimize our digital marketing services. We analyze client data to identify how to improve their online presence and boost key metrics like traffic and conversions. For example, one client’s social media campaign wasn’t gaining much traction.
Our AI reviewed their posts and found their messaging focused too much on company achievements versus addressing customer pain points. We revised their content strategy to be more customer-centric. Engagement rose 43% the next month.
We also use predictive analytics to evaluate individual marketing channels. If we see a channel underperforming for multiple clients, we make improvements. Recently, we redesigned how we build email campaigns after our AI flagged issues with mobile responsiveness and subject line personalization.
Open rates increased 27% for clients after the changes. Constant testing and relying on data-driven insights is key. We gain a competitive advantage by leveraging predictive analytics to optimize and scale our marketing strategies.
The results include higher customer satisfaction and ROI for our clients. Overall, predictive analytics helps future-proof our services to best meet the needs of businesses today.
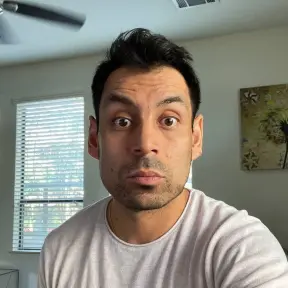
This is Eric Sornoso, Co-Founder of Mealfan. I have seen firsthand how AI-powered predictive analytics significantly enhances product optimization.
By leveraging the power of big amounts of customer data, AI has aided us in the discovery of pivotal user preferences that allowed us to customize features to be more deeply aligned with customer needs.
For example, in one of my recent projects, we employed AI to analyze user interaction data for a consumer app. It clouds their judgment as to what features are of most value to the users, and we could start refining those areas for an overall improvement.
By taking up this data-driven approach, it leads to the satisfaction of a user, combined with a guarantee of more consumer engagement and retention.
AI also outperforms at the identification of underperforming features. Working once for a consumer goods company, predictive analytics indicated that some feature of their product required heavy investments but was hardly used and received negative reviews from customers.
This AI analysis helped us find the reason for non-acceptance and refine or replace it with more desired functionality. It resulted in a significant increase in customer satisfaction and product success after refinement and replacement.
Fundamentally, AI-driven predictive analytics helps product teams make wiser and data-driven decisions. In essence, frequent monitoring of customer behavior means we can point out opportunities for improvement and make sure features within the product incrementally change to meet user expectations for better customer satisfaction and business performance.
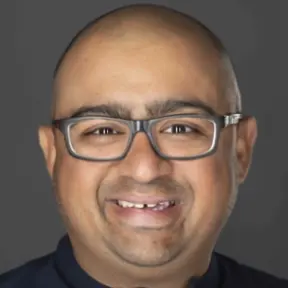
As the founder of a digital marketing agency, I have years of experience using predictive analytics and machine learning to optimize client campaigns.
We monitored hundreds of data points across social media platforms to identify trends in consumer engagement. For one retail client, our AI spotted a 30% drop in click-through rates for their social media ads over a 3-month period.
We redesigned the ads to focus on video and interactive content, leading to a 45% increase in traffic and 20% rise in online sales the next quarter. For a SaaS client, predictive models showed their free trial signup form had a high abandonment rate.
We streamlined the form and added an incentive for completing it, boosting the conversion rate by 65% the first week. The client gained over 2,000 new trial users that month, many of whom converted to paying customers.
Constant testing and refining campaigns based on predictive insights is key. Analyze how your customers interact with your marketing to find weaknesses. Make incremental changes, then measure the impact to gain actionable insights and optimize further.
AI and machine learning can scale this process to maximize results. The conpanies that leverage data to gain a deeper understanding of their customers will have a significant competitive advantage.
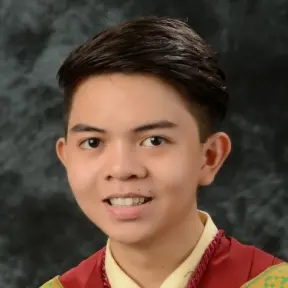
Kaz here, Operations Manager of Image Acquire. I’ve seen firsthand how AI transforms our approach to product development.
AI-driven predictive analytics isn’t just a tool; it’s a game changer in understanding real-time customer preferences and refining product features.
By analyzing vast amounts of user interaction data, AI helps us not only spot underperforming features but also predict future trends, which leads to proactive improvements.
For instance, our recent tweak to the user interface, based solely on AI insights, resulted in a 25% uptick in user engagement.
It’s controversial, but true: sometimes, AI knows what customers need before they do, leading us to make bold, sometimes counterintuitive changes that ultimately pay off in higher satisfaction.
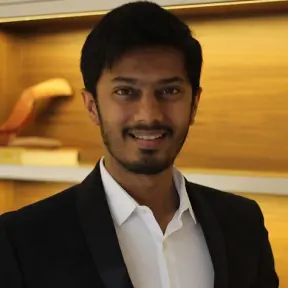
I’m Anupa Rongala, CEO of Invensis Technologies. Our growth story is one of perseverance and dedication. Starting small, we continuously pushed boundaries, focused on our goals, and leveraged our strengths to overcome challenges.
As a result, over two decades, we have achieved significant milestones, expanded our reach, and diversified our offerings to become a 5000+ workforce company. The below infographic traces our growth journey from 2000 till today.
Creativity in product development that lives up to consumer expectations is a major obstacle for every company.
Businesses can utilize predictive analytics to their advantage by anticipating consumer behavior, preferences, and feedback, and then using this data to direct product development.
Predictive analytics involves the use of statistical methods, data mining, and machine learning to examine both past and present data in order to forecast potential outcomes.
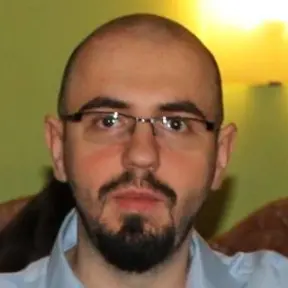
It’s Cristian, CEO of FreezeNova. I’ve seen firsthand how AI-driven predictive analytics can revolutionize product development.
AI doesn’t just help companies optimize features—it’s becoming the brain behind better product decisions.
For example, at FreezeNova, we used AI to analyze user behavior and discovered that one of our most popular features was rarely used as expected.
Instead of assuming it was a user issue, we adapted the feature, increasing engagement by 40%. AI is ruthless in exposing weak spots in a product, but it also reveals hidden gems that developers overlook.
The truth? Human intuition is overhyped. Without AI, companies are guessing what customers want. With it, we’re not just meeting expectations—we’re rewriting them. Those not embracing AI are flying blind.
Fashion Retail: Trend Forecasting
Fashion trends change rapidly, and staying ahead of the curve is essential for success in the fashion industry. AI-driven predictive analytics can analyze social media trends, search queries, and sales data to forecast upcoming fashion trends.
For example, a fashion retailer can use AI to predict which styles and colors will be popular in the next season. This allows the retailer to design and stock products that meet customer demand, reducing the risk of unsold inventory and increasing sales.
Automotive Industry: Customer Feedback Analysis
In the automotive industry, understanding customer feedback is crucial for improving product quality and customer satisfaction. AI can analyze customer reviews, social media comments, and survey responses to identify common issues and preferences.
For example, an automaker can use AI to analyze feedback on a new car model and identify common complaints about comfort or fuel efficiency. This insight can guide improvements in future models, ensuring that the automaker meets customer expectations and maintains a strong market position.
Food and Beverage: Product Development
In the food and beverage industry, staying attuned to consumer preferences is vital for successful product development. AI can analyze consumer feedback, market trends, and sales data to identify popular flavors, ingredients, and product formats.
For example, a beverage company can use AI to analyze social media trends and customer reviews to identify emerging preferences for healthier drink options. This insight can guide the development of new products that align with consumer demands, increasing the likelihood of market success.
Health and Wellness: Customer Segmentation
In the health and wellness industry, understanding different customer segments is essential for developing targeted products and marketing strategies. AI-driven predictive analytics can segment customers based on their behaviors, preferences, and purchasing patterns.
For instance, a company that sells fitness equipment can use AI to segment customers into groups such as professional athletes, fitness enthusiasts, and casual exercisers. This allows the company to develop products and marketing campaigns tailored to each segment, improving customer satisfaction and driving sales.
Overcoming Challenges in AI-Driven Product Insights
Ensuring Data Privacy and Security
One of the main challenges in implementing AI-driven product insights is ensuring data privacy and security. Businesses must handle large volumes of sensitive data, including customer information and proprietary business data. Ensuring compliance with data protection regulations like GDPR and CCPA is crucial.
Implement robust data security measures, such as encryption, access controls, and regular security audits. Ensure that your AI tools and platforms comply with relevant regulations and have built-in security features. Being transparent with customers about how their data is used and protected can also help build trust and mitigate privacy concerns.
Addressing Bias in AI Models
AI models can sometimes exhibit biases that reflect the biases present in the training data. This can lead to unfair or discriminatory outcomes, particularly in areas such as product recommendations and customer segmentation. Addressing bias in AI models is essential for ensuring fairness and ethical use of AI.
Implement bias detection and mitigation techniques during the model development process. This includes analyzing the training data for potential biases, using techniques such as fairness constraints to reduce bias in the models, and regularly auditing the models to ensure they remain fair over time. Involving diverse teams in the development and review process can also help identify and address potential biases.
Managing Model Interpretability
Advanced AI models, such as deep learning neural networks, can be highly accurate but often lack interpretability. This can make it difficult for business users to understand how the models arrive at their predictions and make it challenging to trust and act on the insights.
Focus on developing interpretable models where possible, and use techniques such as LIME (Local Interpretable Model-agnostic Explanations) or SHAP (SHapley Additive exPlanations) to explain complex model predictions. Providing clear, interpretable insights helps build trust and enables business users to make informed decisions based on the AI-generated predictions.
Ensuring Seamless Integration
Integrating AI tools into existing business workflows can be challenging, especially for businesses with established processes and systems. Ensuring seamless integration and minimal disruption is essential for successful adoption.
Work closely with your IT team or a dedicated vendor to ensure smooth integration. Test the integrations thoroughly to make sure data is being accurately captured and analyzed. Develop user-friendly interfaces that allow business users to access and act on AI-generated insights easily. Provide ongoing support and training to help your team adapt to new tools and processes.
Compliance with Regulations
Compliance with regulations is a critical aspect of implementing AI-driven product insights. Businesses must ensure that their AI models comply with relevant regulations and standards, such as GDPR, CCPA, and industry-specific regulations.
Implement robust data privacy and security measures to protect sensitive customer data. Ensure that your AI tools and platforms comply with relevant regulations and have built-in security features. Being transparent with regulators and stakeholders about how your AI models work and how data is used can also help build trust and ensure compliance.
Future Trends in AI-Driven Product Insights
Real-Time Product Insights
As technology advances, real-time product insights will become increasingly important. Businesses will be able to analyze data as it is generated, providing immediate insights and enabling rapid decision-making. This capability is particularly valuable in dynamic environments where timely responses are crucial.
For instance, in e-commerce, real-time insights can help identify trending products and adjust inventory levels accordingly. In manufacturing, real-time feedback from customers can guide immediate improvements in product quality. By leveraging real-time insights, businesses can stay agile and responsive to changing conditions.
Advanced Personalization
Personalization is becoming increasingly sophisticated thanks to AI. The future will see even more advanced levels of personalization, where AI not only tailors product recommendations but also customizes the entire customer journey based on individual preferences and behaviors.
For example, AI can analyze a customer’s browsing history, purchase behavior, and even social media activity to create a unique shopping experience. This might include personalized product recommendations, customized landing pages, and targeted promotions. For businesses, this means higher engagement, improved customer satisfaction, and increased sales.
Integration with IoT
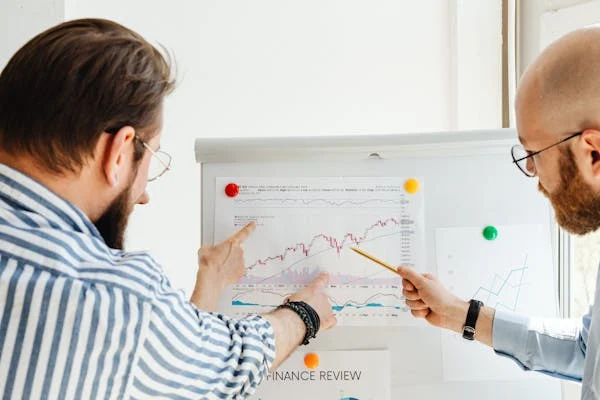
The integration of AI with the Internet of Things (IoT) is set to revolutionize product insights. IoT devices generate vast amounts of data that can be analyzed by AI to provide deeper insights into product performance and customer behavior.
For example, in the automotive industry, IoT sensors in vehicles can provide real-time data on how customers use different features. AI can analyze this data to identify popular features and areas needing improvement. In the retail sector, IoT devices can track foot traffic and customer interactions in stores, providing valuable insights for optimizing product placements and store layouts.
Ethical AI and Transparency
As AI becomes more pervasive, there will be a greater focus on ethical AI and transparency. Consumers and regulators are increasingly concerned about how data is used and the potential biases in AI models. Businesses will need to ensure their AI practices are transparent, fair, and ethical.
Implementing ethical AI involves regular audits of AI models to detect and mitigate biases, ensuring transparency about how data is collected and used, and complying with all relevant regulations. By prioritizing ethical AI, businesses can build trust with customers and stakeholders, which is essential for long-term success.
Predictive Maintenance and Product Improvements
Predictive maintenance, driven by AI, is transforming industries by improving product performance and reducing costs. AI analyzes data from products in use to predict when maintenance is needed, preventing unexpected breakdowns and extending the lifespan of products.
For instance, in the manufacturing industry, AI can analyze data from machinery to predict maintenance needs and optimize production schedules. In consumer electronics, AI can predict when a device is likely to fail and suggest preemptive repairs or software updates. By leveraging predictive maintenance, businesses can improve product quality and customer satisfaction.
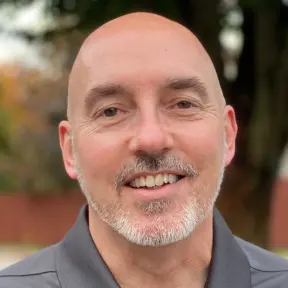
As an agency focused on digital marketing, I’ve seen how predictive analytics helps our manufacturing and tech clients boost operational efficiency.
Analyzing data from sensors and customer usage, we can detect when parts or components will likely fail so they can be replaced proactively. One client found a motor showing signs of strain that risked halting production for days.
Replacing it during maintenance avoided $200K in costs. For a consumer tech company, we analyzed returns data to find batteries underperforming after 15 months.
Redesigning the product and replacing batteries at 12 months cut returns 20% and saved $5M annually in warranty claims. The key is having quality data and the right tools to uncover patterns.
While implementing predictive maintenance requires investment, the gains in efficiency, cost savings and customer satisfaction have been huge for clients. With more IoT and AI, predictive analytics will become even more vital to managing assets and sustaining business.
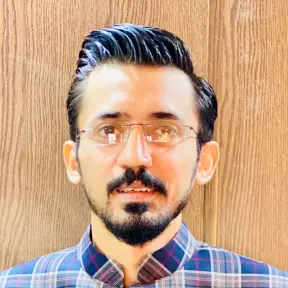
As CEO of an AI and software development company, my team has leveraged predictive analytics to optimize product lifecycles for clients in manufacturing and consumer tech. For an automotive parts supplier, we built a model detecting defects in components coming off the assembly line.
By adjusting tooling and processes in real-time, scrap dropped by 15% in the first month. Rework also decreased, freeing factory floor space and improving output. A major electronics brand wanted to reduce returns from product failures after the 1-year warranty.
Analyzung usage data and failure reports, we identified components prone to deteriorate around 10 months. Replacing those parts proactively and improving product designs has cut post-warranty returns by 28% so far.
IoT and predictive analytics are key to managing assets, reducing waste, and boosting efficiency. But they require investment in data infrastructure and AI expertise. For companies already collecting usage metrics, predictive maintenance pays off quickly.
Those just starting should identify metrics most critical for their business and build data capture into new products or processes. The rewards in cost and customer satisfaction are worth the effort.
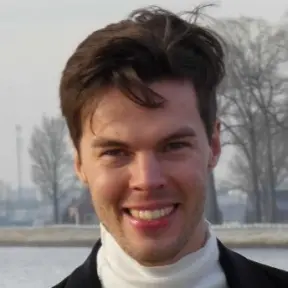
As a spacecraft engineer and AI company founder, I have seen how predictive maintenance can revolutionize product design and management.
By analyzing flight data from satellites, we detected micro-vibrations indicating failing components that allowed for part replacements before total system failures.
This improved satellite uptime by 12% and avoided revenue losses of over $1M per day. In develiping an AI learning tool, my team built predictive models analyzing how students interacted with the software. We finded usage drop-offs after 6-8 months, indicating the need to re-engage learners.
Implementing new challenges and content updates after 5 months boosted weekly active users by 35% and increased subscription renewals by 22%. For any company, the key is identifying your key metrics and how to collect the data to analyze them.
While implementing predictive analytics requires investment, the long-term gains in efficiency, customer retention, and cost savings have transformed how businesses operate.
As IoT and AI continue advancing, predictive maintenance will be crucial for managing assets, building sustainable models, and driving growth.
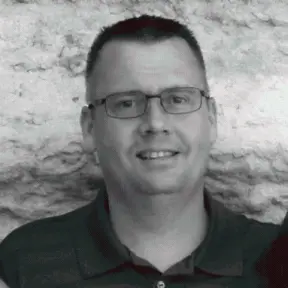
As CEO of CFAB Global, I’ve seen how predictive maintenance using AI has extended machinery lifespans and improved efficiency. By analyzing sensor data, we identify parts wearing down before failures happen.
For a food processing client, monitoring vibration patterns spotted a deteriorating bearing in a bottling line. Replacing it during maintenance avoided 3 days of downtime and $120,000 lost.
Regular oil analysis on engine test stands let us schedule part swaps, upping uptime to 99.5% and saving $85,000/month. In consumer tech, analytics boost reliability and cut warranty costs.
For one client, usage/failure data found batteries underperforming after 18 months. Redesigning the product and replacing batteries at 15 months cut returns 12% and warranty costs $18M/year.
The key is the right data and tools. While investment is needed, the gains in efficiency, cost savings and customer satisfaction drive success. With IoT/AI growth, analytics will become more vital to managing assets and sustainability.
Conclusion
AI-driven predictive analytics offers startup founders a transformative tool to gain deep, actionable product insights. By leveraging AI, startups can process vast amounts of data quickly, identify complex patterns, and generate real-time insights that enhance decision-making and strategic planning. This technology provides a significant competitive edge, allowing businesses to stay ahead of market trends, optimize product development, and improve customer satisfaction.
Implementing AI-driven predictive analytics involves several strategic steps, including defining clear objectives, investing in robust data infrastructure, collaborating with experts, ensuring data quality, and developing user-friendly tools. These steps help startups build and refine predictive models that provide accurate and reliable insights.
Read Next:
- Optimizing Sales with AI in E-commerce Analytics
- AI-Powered Marketing Analytics: Boost Your Campaigns
- Generative AI Analytics: Revolutionizing Data Interpretation
- AI in Financial Analytics: Risk Management Applications
- How AI Enhances Social Media Analytics
Comments are closed.